Architectures for Detecting Interleaved Multi-Stage Network Attacks Using Hidden Markov Models
IEEE TRANSACTIONS ON DEPENDABLE AND SECURE COMPUTING(2021)
摘要
With the growing amount of cyber threats, the need for development of high-assurance cyber systems is becoming increasingly important. The objective of this article is to address the challenges of modeling and detecting sophisticated network attacks, such as multiple interleaved attacks. We present the interleaving concept and investigate how interleaving multiple attacks can deceive intrusion detection systems. Using one of the important statistical machine learning (ML) techniques, Hidden Markov Models (HMM), we develop two architectures that take into account the stealth nature of the interleaving attacks, and that can detect and track the progress of these attacks. These architectures deploy a database of HMM templates of known attacks and exhibit varying performance and complexity. For performance evaluation, in the presence of multiple multi-stage attack scenarios, various metrics are proposed which include (1) attack risk probability, (2) detection error rate, and (3) the number of correctly detected stages. Extensive simulation experiments are used to demonstrate the efficacy of the proposed architectures.
更多查看译文
关键词
Hidden Markov models,Intrusion detection,Computer crime,Computer architecture,Complexity theory,Servers,Cyber systems,network security,intrusion detection,Hidden Markov Model,interleaved attacks
AI 理解论文
溯源树
样例
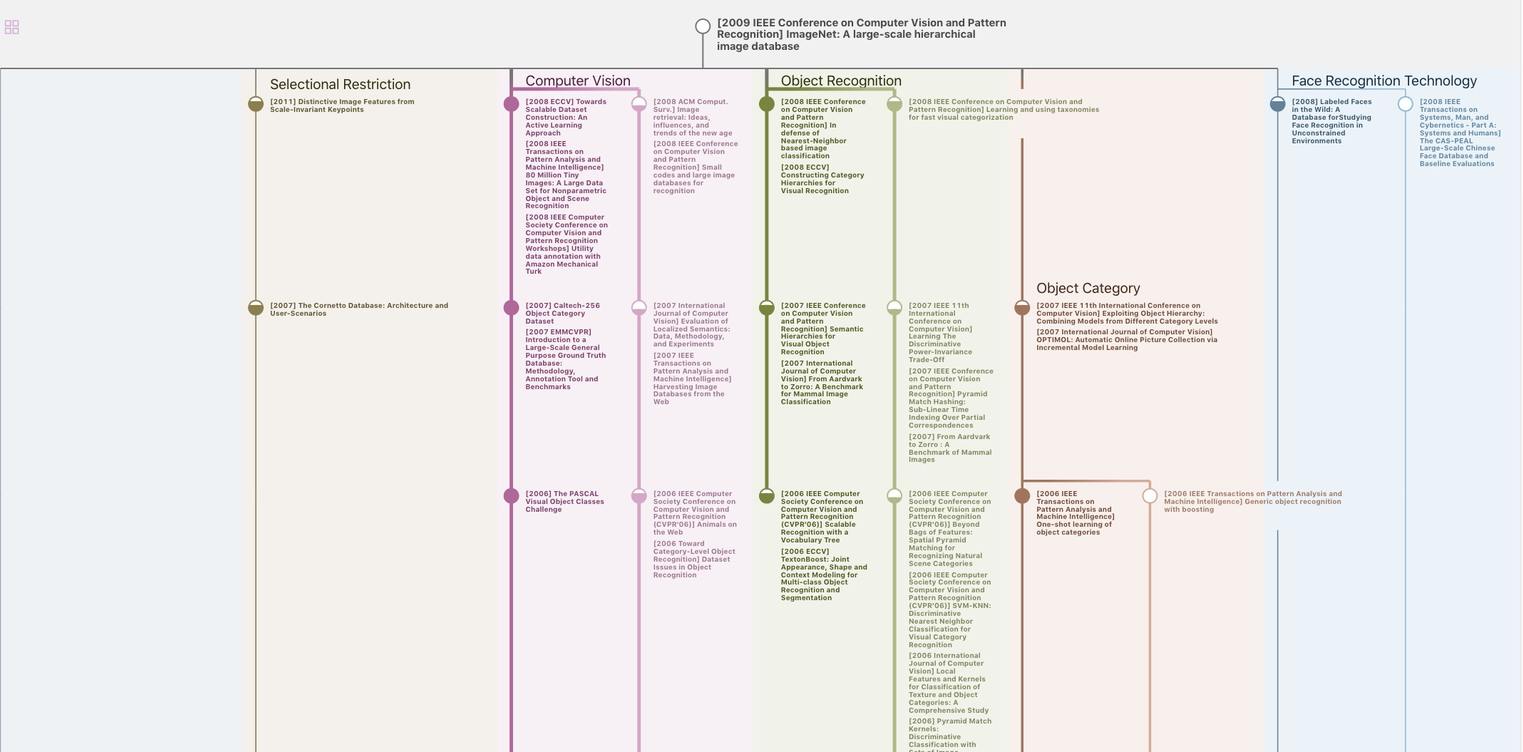
生成溯源树,研究论文发展脉络
Chat Paper
正在生成论文摘要