Spiking Reservoir Networks: Brain-inspired recurrent algorithms that use random, fixed synaptic strengths
IEEE Signal Processing Magazine(2019)
摘要
A class of brain-inspired recurrent algorithms known as reservoir computing (RC) networks reduces the computational complexity and cost of training machine-learning models by using random, fixed synaptic strengths. This article offers insights about a spiking reservoir network, the liquid state machine (LSM), the inner workings of the algorithm, the design metrics, and neuromorphic designs. The discussion extends to variations of the LSM that incorporate local plasticity mechanisms and hierarchy to improve performance and memory capacity.
更多查看译文
关键词
Neurons, Signal processing algorithms, Machine learning, Artificial neural networks, Neuromorphics, Bio-inspired computing, Brain modeling, Recurrent neural networks, Computational modeling
AI 理解论文
溯源树
样例
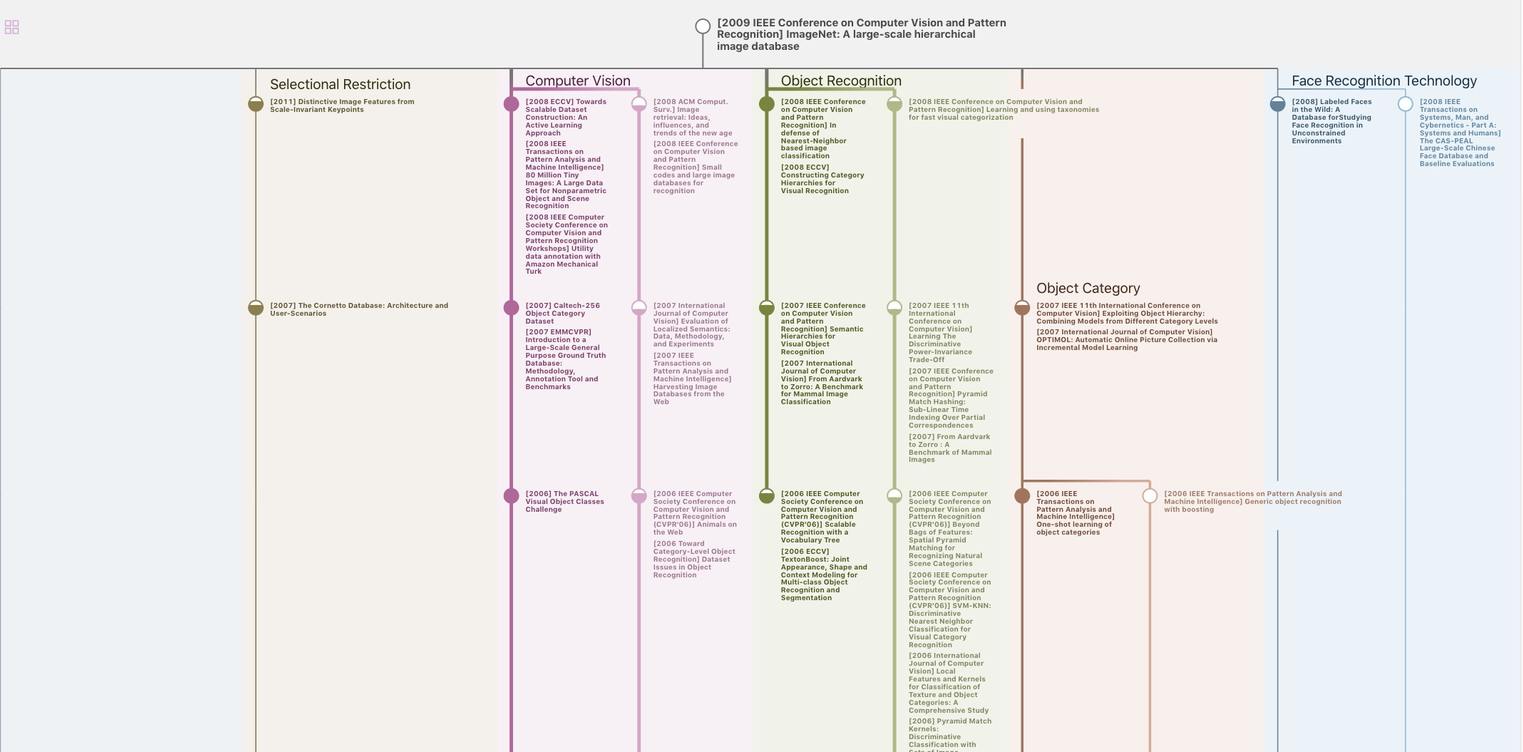
生成溯源树,研究论文发展脉络
Chat Paper
正在生成论文摘要