BHIN2vec: Balancing the Type of Relation in Heterogeneous Information Network
Proceedings of the 28th ACM International Conference on Information and Knowledge Management(2019)
摘要
The goal of network embedding is to transform nodes in a network to a low-dimensional embedding vectors. Recently, heterogeneous network has shown to be effective in representing diverse information in data. However, heterogeneous network embedding suffers from the imbalance issue, i.e. the size of relation types (or the number of edges in the network regarding the type) is imbalanced. In this paper, we devise a new heterogeneous network embedding method, called BHIN2vec, which considers the balance among all relation types in a network. We view the heterogeneous network embedding as simultaneously solving multiple tasks in which each task corresponds to each relation type in a network. After splitting the skip-gram loss into multiple losses corresponding to different tasks, we propose a novel random-walk strategy to focus on the tasks with high loss values by considering the relative training ratio. Unlike previous random walk strategies, our proposed random-walk strategy generates training samples according to the relative training ratio among different tasks, which results in a balanced training for the node embedding. Our extensive experiments on node classification and recommendation demonstrate the superiority of BHIN2vec compared to the state-of-the-art methods. Also, based on the relative training ratio, we analyze how much each relation type is represented in the embedding space.
更多查看译文
关键词
heterogeneous network, inverse training ratio, multitask learning, network embedding, random-walk strategy, stochastic matrix
AI 理解论文
溯源树
样例
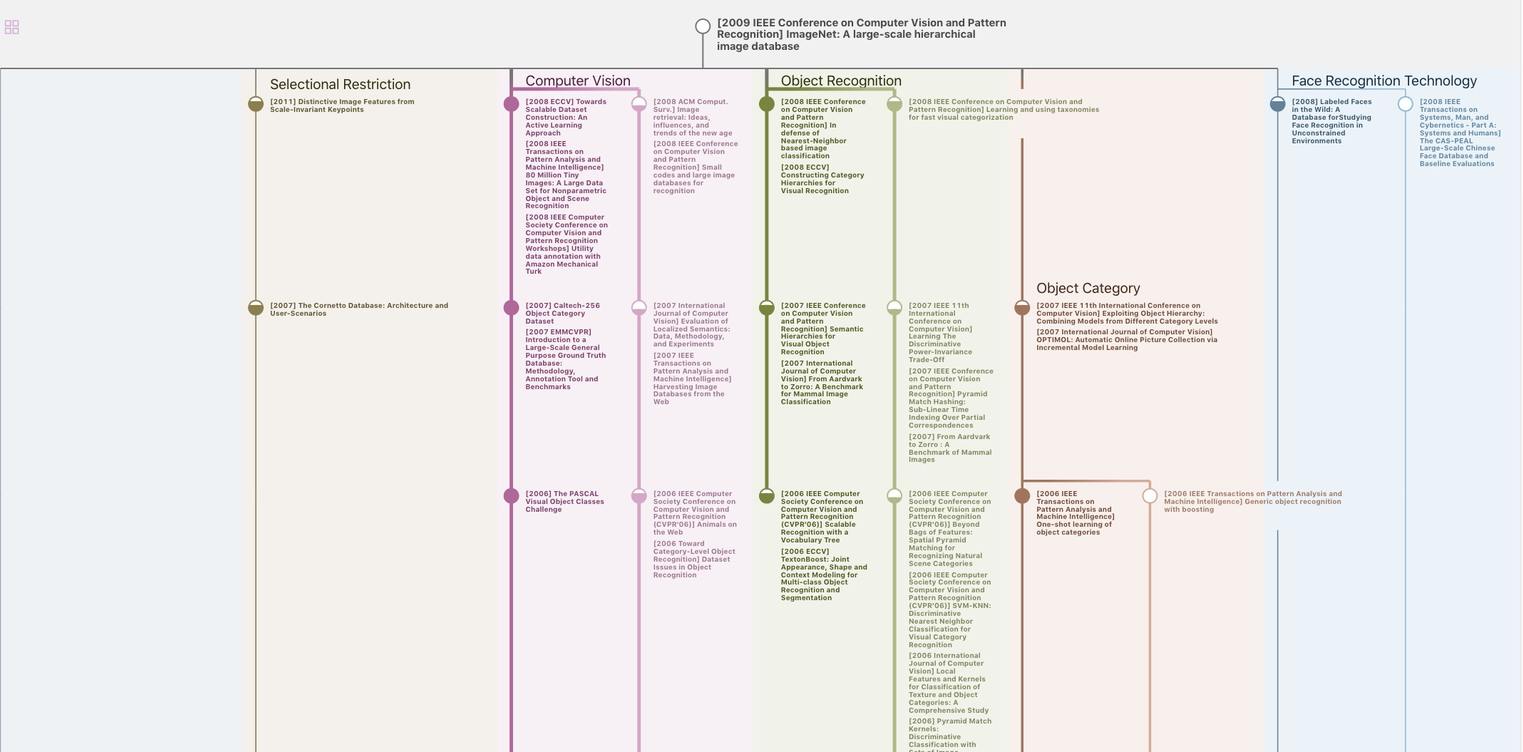
生成溯源树,研究论文发展脉络
Chat Paper
正在生成论文摘要