PODIUM: Probabilistic Datalog Analysis via Contribution Maximization
Proceedings of the 28th ACM International Conference on Information and Knowledge Management(2019)
摘要
The use of probabilistic datalog programs has been advocated for applications that involve recursive computation and uncertainty. While using such programs allows for a flexible knowledge derivation, it makes the analysis of query results a challenging task. Particularly, given a set O of output tuples and a number k, one would like to understand which k-size subset of the input tuples has affected the most the derivation of O. This is useful for multiple tasks, such as identifying critical sources of errors and understanding surprising results. To this end, we formalize the Contribution Maximization problem and present an efficient algorithm to solve it. Our algorithm injects a refined variant of the classic Magic Sets technique, integrated with a sampling method, into top-performing algorithms for the well-studied Influence Maximization problem. We propose to demonstrate our solution in a system called PODIUM. We will demonstrate the usefulness of PODIUM using real-life data and programs, and illustrate the effectiveness of our algorithm.
更多查看译文
关键词
probabilistic datalog, results explanations
AI 理解论文
溯源树
样例
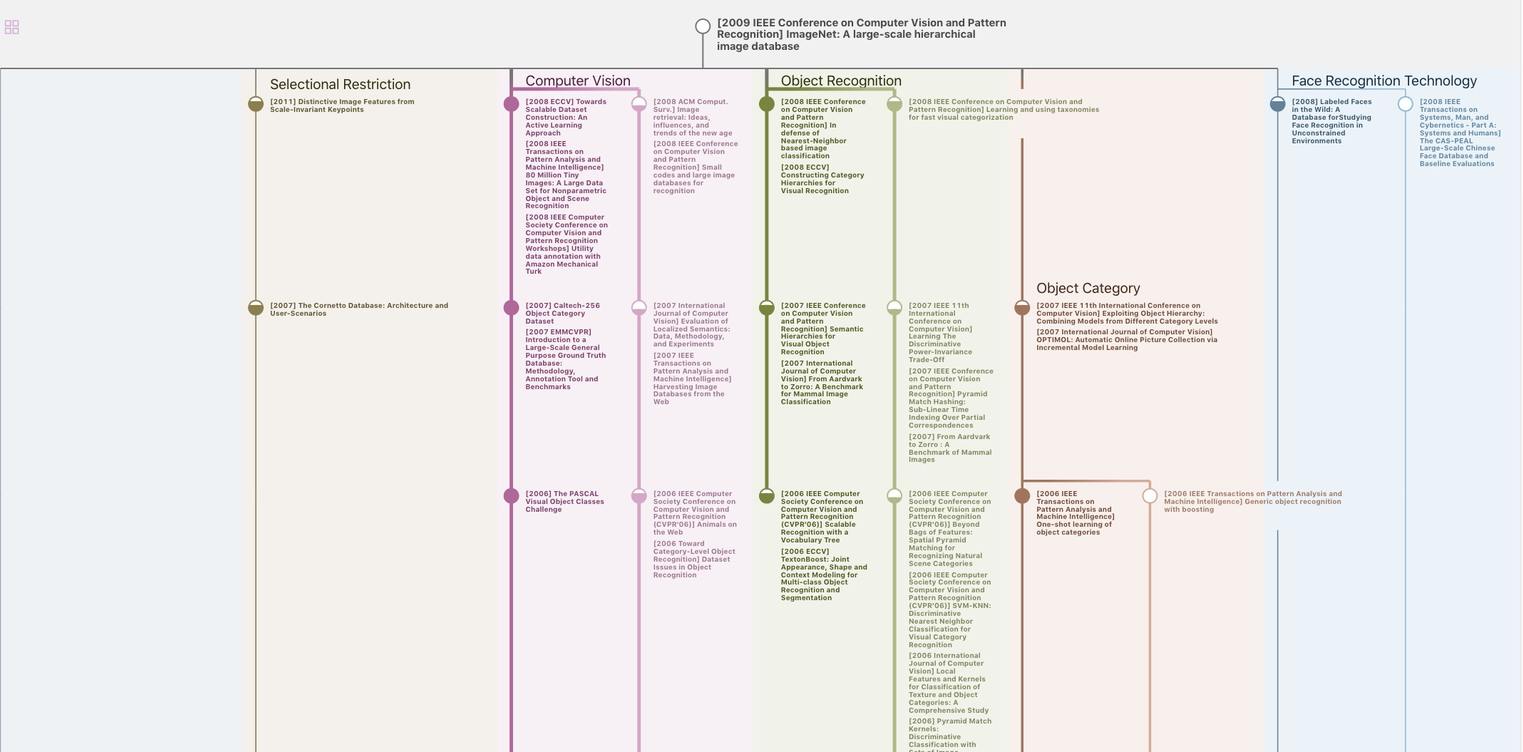
生成溯源树,研究论文发展脉络
Chat Paper
正在生成论文摘要