Adaptive Feature Sampling for Recommendation with Missing Content Feature Values
Proceedings of the 28th ACM International Conference on Information and Knowledge Management(2019)
摘要
Most recommendation algorithms mainly make use of user history interactions in the model, while these methods often suffer from the cold-start problem (user/item has no history information). On the other sides, content features help on cold-start scenarios for modeling new users or items. So it is essential to utilize content features to enhance different recommendation models. To take full advantage of content features, feature interactions such as cross features are used by some models and outperform than using raw features. However, in real-world systems, many content features are incomplete, e.g., we may know the occupation and gender of a user, but the values of other features (location, interests, etc.) are missing. This missing-feature-value (MFV) problem is harmful to the model performance, especially for models that rely heavily on rich feature interactions. Unfortunately, this problem has not been well studied previously.
In this work, we propose a new adaptive "Feature Sampling'' strategy to help train different models to fit distinct scenarios, no matter for cold-start or missing feature value cases. With the help of this strategy, more feature interactions can be utilized. A novel model named CC-CC is proposed. The model takes both raw features and the feature interactions into consideration. It has a linear part to memorize useful variant information from the user or item contents and contexts (Content & Context Module), and a deep attentive neural module that models both content and collaborate information to enhance the generalization ability (Content & Collaborate Module). Both parts have feature interactions. The model is evaluated on two public datasets. Comparative results show that the proposed CC-CC model outperforms the state-of-the-art algorithms on both warm and cold scenarios significantly (up to 6.3%). To the best of our knowledge, this model is the first clear and powerful model that proposed to handle the missing feature values problem in deep neural network frameworks for recommender systems.
更多查看译文
关键词
feature interaction, feature sampling, missing feature value, neural recommendation model
AI 理解论文
溯源树
样例
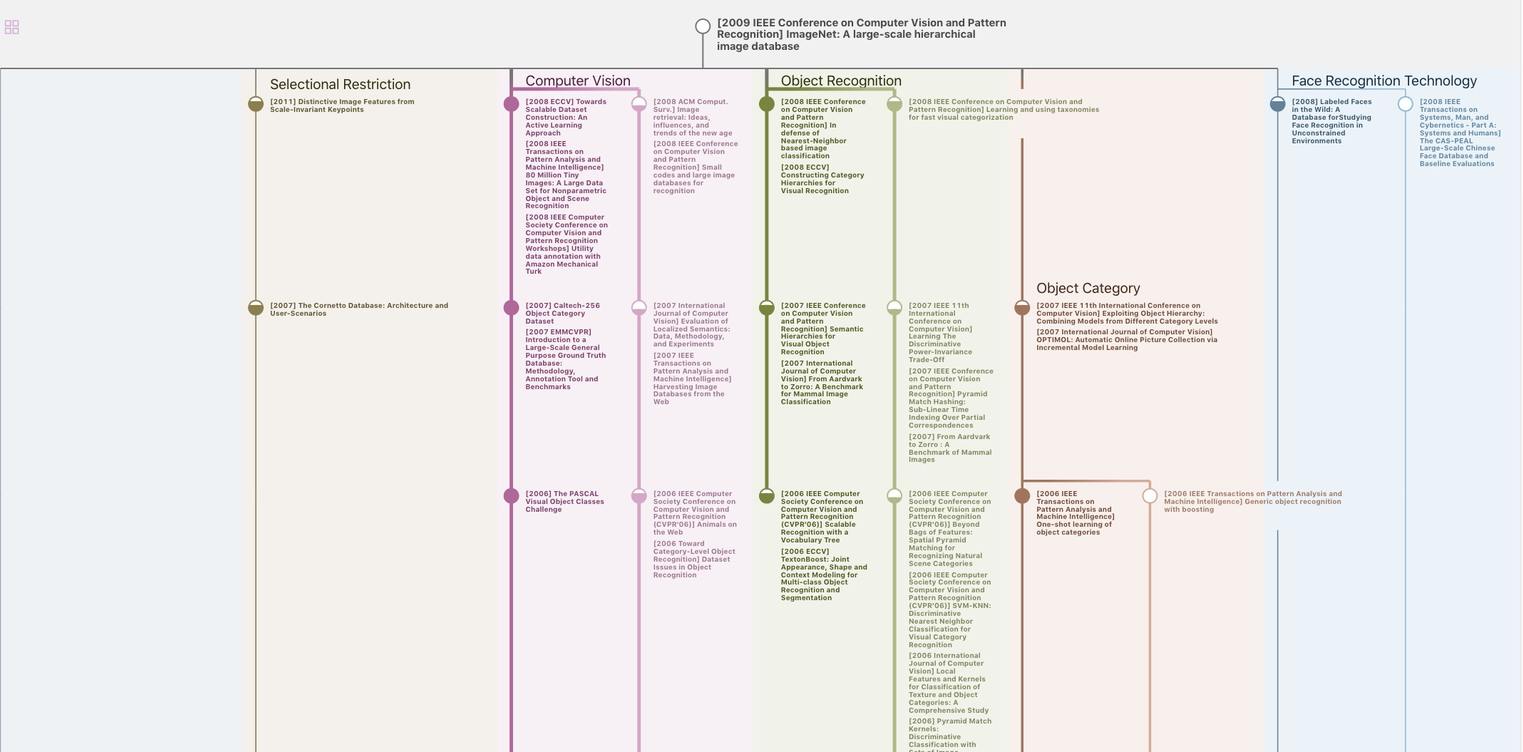
生成溯源树,研究论文发展脉络
Chat Paper
正在生成论文摘要