Time-Series Aware Precision and Recall for Anomaly Detection: Considering Variety of Detection Result and Addressing Ambiguous Labeling
Proceedings of the 28th ACM International Conference on Information and Knowledge Management(2019)
摘要
We proposetime-series aware precision andrecall, which are appropriate for evaluating anomaly detection methods in time-series data. In time-series data, an anomaly corresponds toa series of instances. The conventional metrics, however, overlook this characteristic, so they suffer from a problem of giving a high score to the method that only detects a long anomaly. To overcome the problem, our metrics consider thevariety of the detected anomalies to be more important through two scoring strategies,detection scoring (\ie, how many anomalies are detected) andportion scoring (\ie, how precisely each anomaly is detected). Moreover, our metrics concernambiguous instances, which indicate the instances labeled as 'normal' although they are affected by their precedent anomaly. Our metrics give smaller scores to those instances as they are likely to be anomalous. We demonstrate that our metrics are more suitable for time-series data compared to existing metrics by evaluations using a real-world dataset as well as several examples.\footnoteOur code and the detection results are available at: https://github.com/saurf4ng/TaPR.
更多查看译文
关键词
accuracy metric, anomaly detection, precision, recall, time-series
AI 理解论文
溯源树
样例
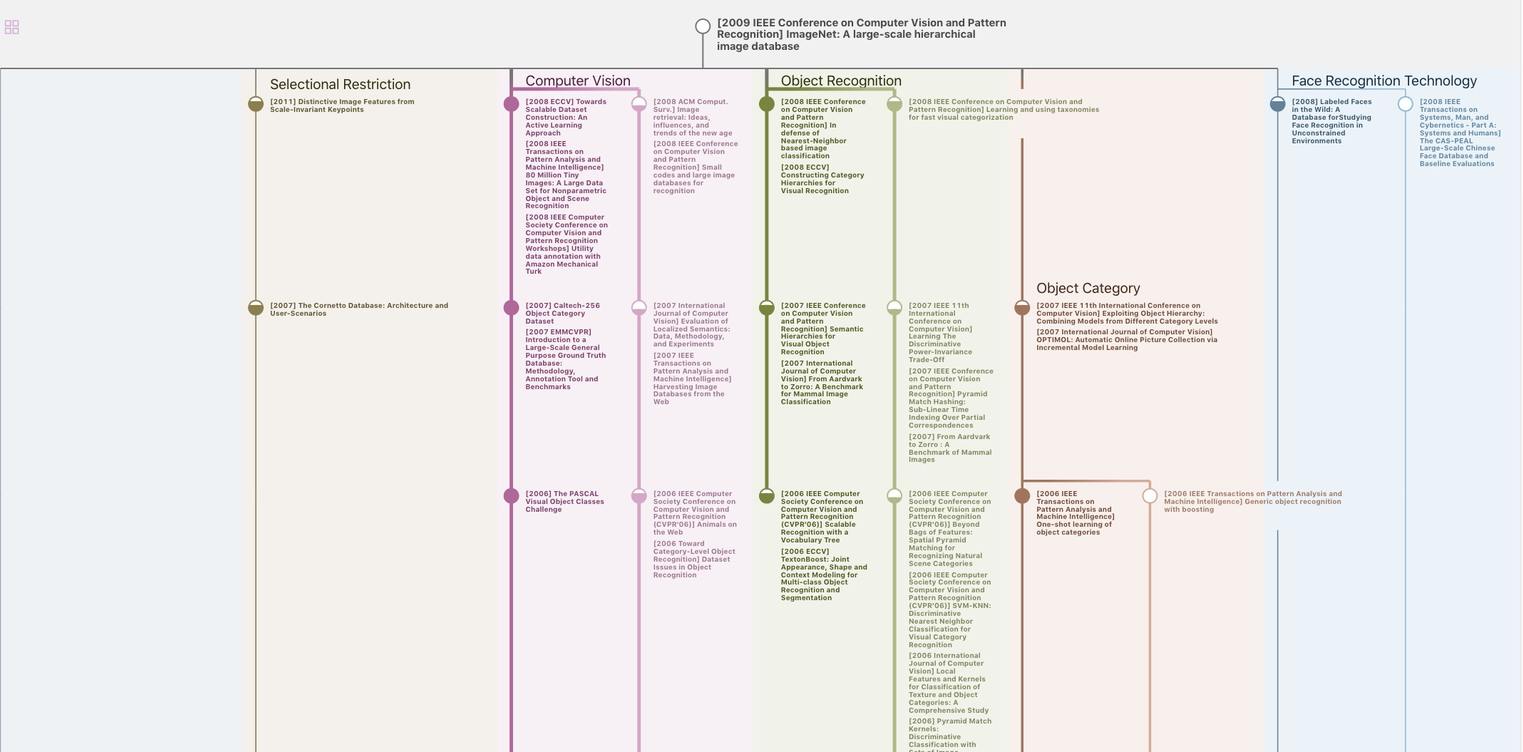
生成溯源树,研究论文发展脉络
Chat Paper
正在生成论文摘要