Semi-Supervised Topological Analysis for Elucidating Hidden Structures in High-Dimensional Transcriptome Datasets.
IEEE/ACM Transactions on Computational Biology and Bioinformatics(2021)
摘要
Topological data analysis (TDA) is a powerful method for reducing data dimensionality, mining underlying data relationships, and intuitively representing the data structure. The Mapper algorithm is one such tool that projects high-dimensional data to 1-dimensional space by using a filter function that is subsequently used to reconstruct the data topology relationships. However, domain context information and prior knowledge have not been considered in current TDA modeling frameworks. Here, we report the development and evaluation of a semi-supervised topological analysis (STA) framework that incorporates discrete or continuously labeled data points and selects the most relevant filter functions accordingly. We validate the proposed STA framework with simulation data and then apply it to samples from Genotype-Tissue Expression data and ovarian cancer transcriptome datasets. The graphs generated by STA for these 2 datasets, based on gene expression profiles, are consistent with prior knowledge, thereby supporting the effectiveness of the proposed framework.
更多查看译文
关键词
Algorithms,Computational Biology,Computer Simulation,Data Mining,Databases, Genetic,Female,Gene Expression Profiling,Humans,Ovarian Neoplasms,Supervised Machine Learning,Transcriptome
AI 理解论文
溯源树
样例
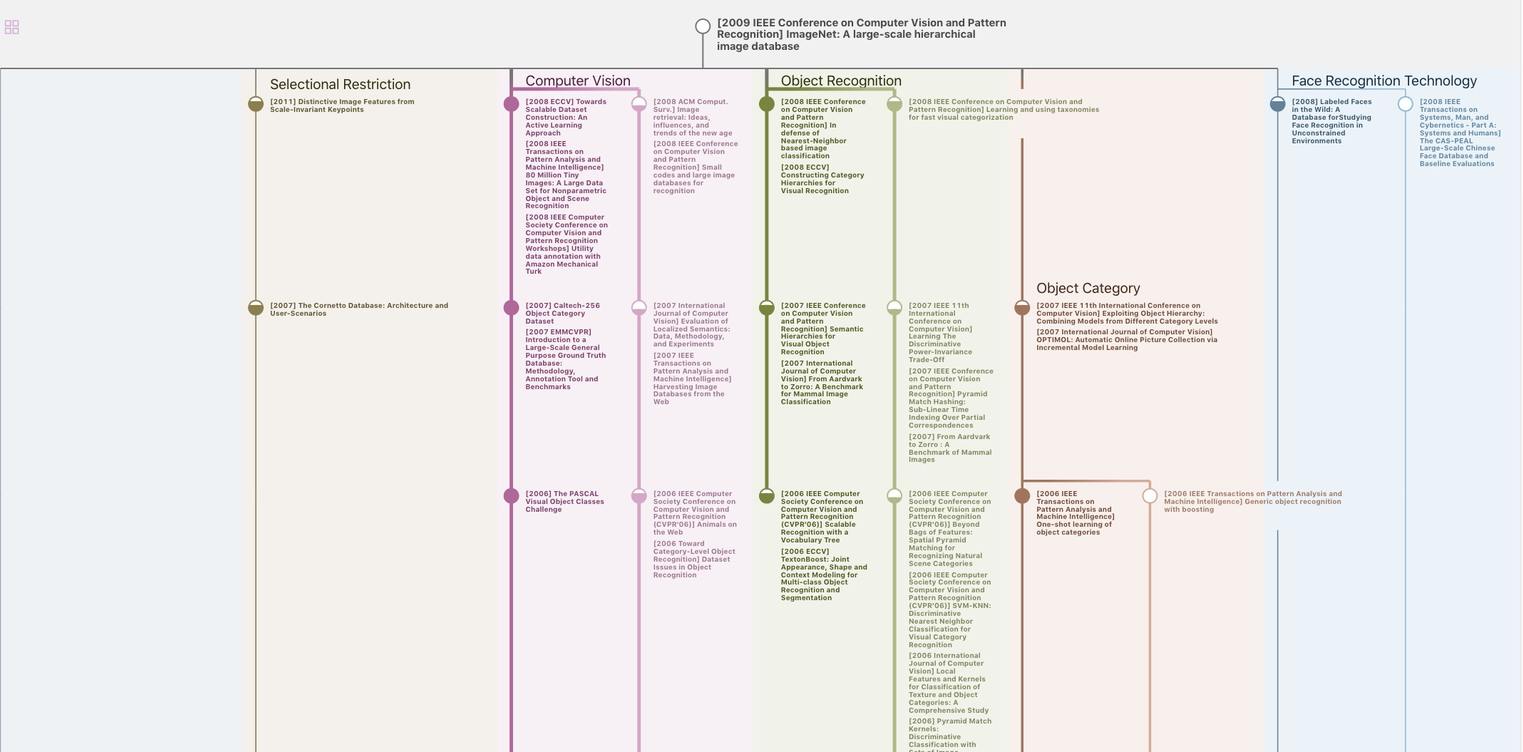
生成溯源树,研究论文发展脉络
Chat Paper
正在生成论文摘要