Multi-Moments in Time: Learning and Interpreting Models for Multi-Action Video Understanding
arxiv(2021)
摘要
An event happening in the world is often made of different activities and actions that can unfold simultaneously or sequentially within a few seconds. However, most large-scale datasets built to train models for action recognition provide a single label per video clip. Consequently, models can be incorrectly penalized for classifying actions that exist in the videos but are not explicitly labeled and do not learn the full spectrum of information that would be mandatory to more completely comprehend different events and eventually learn causality between them. Towards this goal, we augmented the existing video dataset, Moments in Time (MiT), to include over two million action labels for over one million three second videos. This multi-label dataset introduces novel challenges on how to train and analyze models for multi-action detection. Here, we present baseline results for multi-action recognition using loss functions adapted for long tail multi-label learning and provide improved methods for visualizing and interpreting models trained for multi-label action detection.
更多查看译文
关键词
Visualization,Annotations,Training,Analytical models,Three-dimensional displays,Semantics,Convolutional neural networks,Computer vision,machine learning,video,vision and scene understanding,benchmarking,multi-modal recognition,modeling from video,methods of data collection,neural nets
AI 理解论文
溯源树
样例
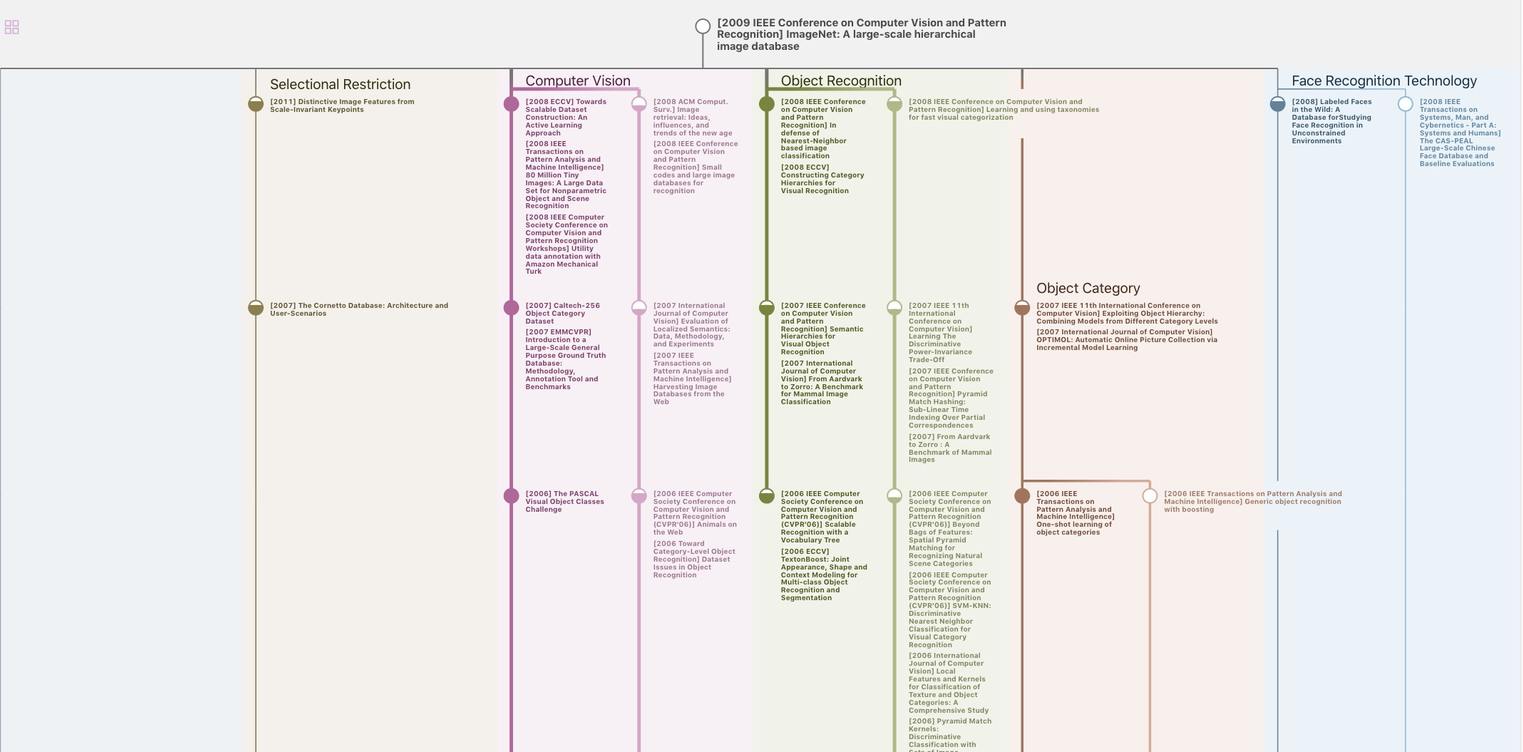
生成溯源树,研究论文发展脉络
Chat Paper
正在生成论文摘要