On the use of supervised machine learning for assessing schedulability: application to ethernet TSN
Proceedings of the 27th International Conference on Real-Time Networks and Systems(2019)
摘要
In this work, we ask if Machine Learning (ML) can provide a viable alternative to conventional schedulability analysis to determine whether a real-time Ethernet network meets a set of timing constraints. Otherwise said, can an algorithm learn what makes it difficult for a system to be feasible and predict whether a configuration will be feasible without executing a schedulability analysis? To get insights into this question, we apply a standard supervised ML technique, k-nearest neighbors (k-NN), and compare its accuracy and running times against precise and approximate schedulability analyses developed in Network-Calculus. The experiments consider different TSN scheduling solutions based on priority levels combined for one of them with traffic shaping. The results obtained on an automotive network topology suggest that k-NN is efficient at predicting the feasibility of realistic TSN networks, with an accuracy ranging from 91.8% to 95.9% depending on the exact TSN scheduling mechanism and a speedup of 190 over schedulability analysis for 106 configurations. Unlike schedulability analysis, ML leads however to a certain rate "false positives" (i.e., configurations deemed feasible while they are not). Nonetheless ML-based feasibility assessment techniques offer new trade-offs between accuracy and computation time that are especially interesting in contexts such as design-space exploration where false positives can be tolerated during the exploration process.
更多查看译文
关键词
machine learning, schedulability analysis, time sensitive networking (TSN), timing verification
AI 理解论文
溯源树
样例
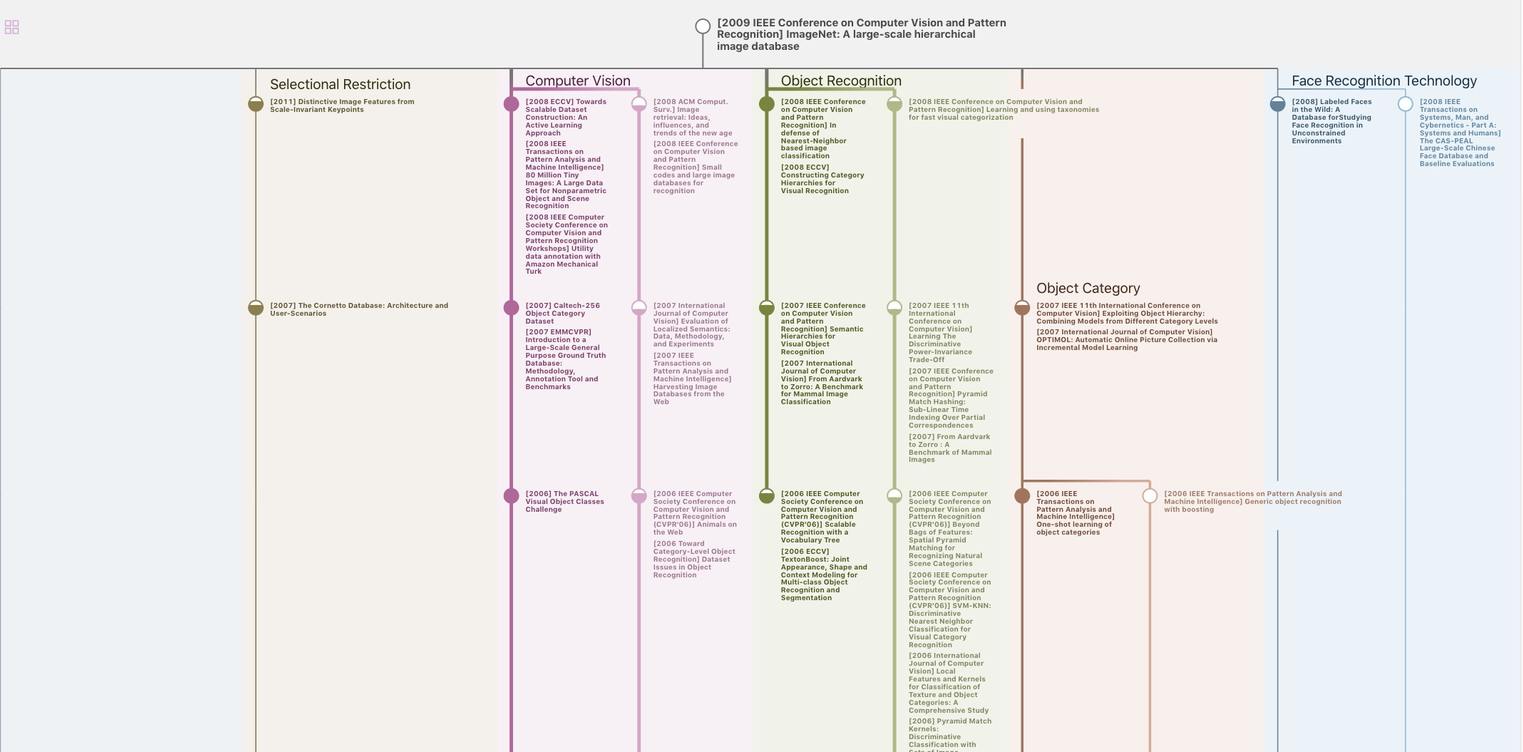
生成溯源树,研究论文发展脉络
Chat Paper
正在生成论文摘要