Penalized least squares and sign constraints with modified Newton-Raphson algorithms: application to EEG source imaging
arxiv(2021)
摘要
We propose a modified Newton-Raphson (MNR) algorithm to estimate multiple penalized least squares (MPLS) models, and its extension to perform efficient optimization over the active set of selected features (AMNR). MPLS models are a more flexible approach to find adaptive least squares solutions that can be simultaneously required to be sparse and smooth. This is particularly important when addressing real-life inverse problems where there is no ground truth available, such as electrophysiological source imaging. The proposed MNR technique can be interpreted as a generalization of the Majorize-Minimize (MM) algorithm to include combinations of constraints. The AMNR algorithm allows to extend some penalized least squares methods to the p much greater than n case, as well as considering sign constraints. We show that these algorithms provide solutions with acceptable reconstruction in simulated scenarios that do not cope with model assumptions, for low n/p ratios. We then use both algorithms for estimating known and new electroencephalography (EEG) inverse models with multiple penalties. Synthetic data were used for a preliminary comparison with the corresponding solutions using the least angle regression (LARS) algorithm according to well-known quality measures; while a visual event-related EEG was used to illustrate its usefulness in the analysis of real experimental data.
更多查看译文
AI 理解论文
溯源树
样例
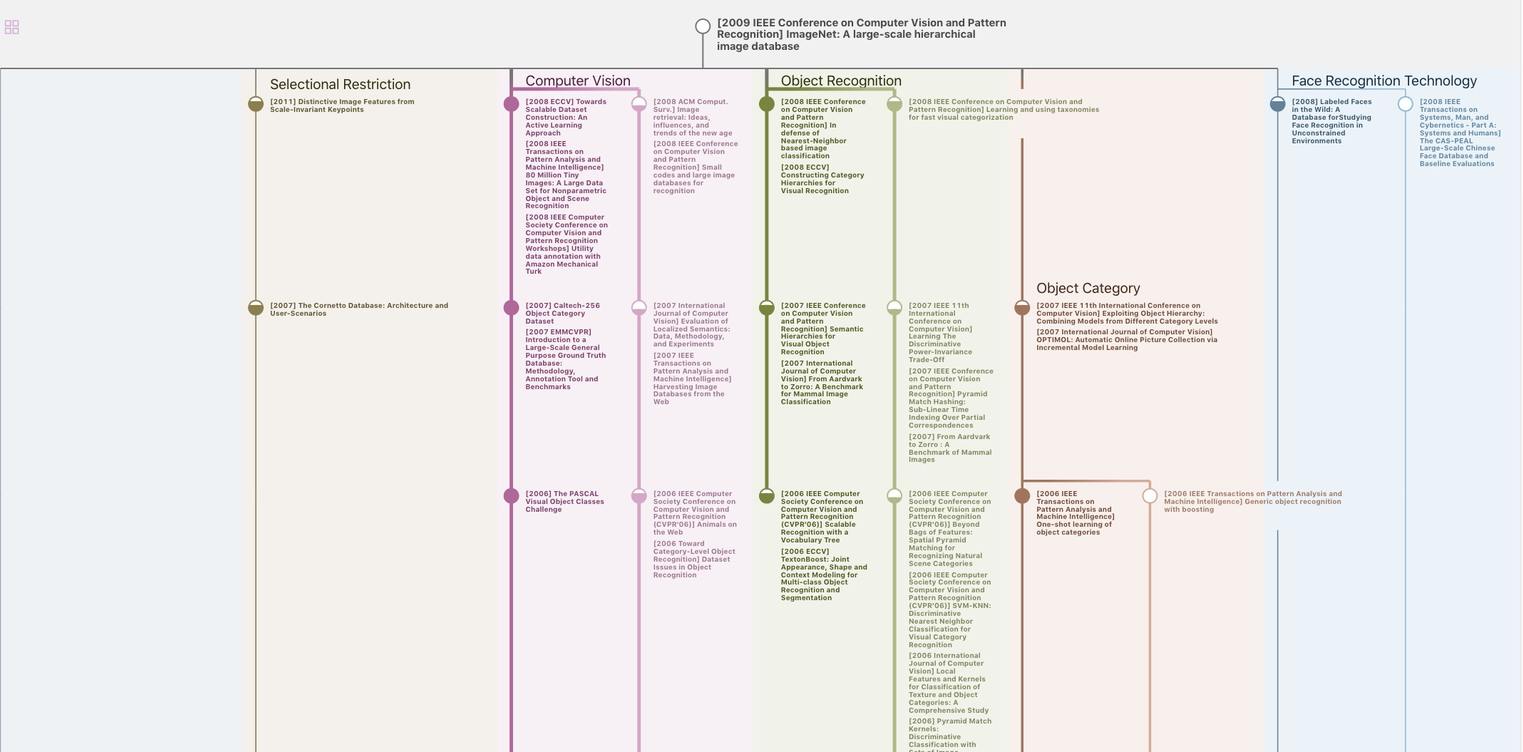
生成溯源树,研究论文发展脉络
Chat Paper
正在生成论文摘要