Prediction of p K a Using Machine Learning Methods with Rooted Topological Torsion Fingerprints: Application to Aliphatic Amines.
JOURNAL OF CHEMICAL INFORMATION AND MODELING(2019)
Abstract
The acid-base dissociation constant, pK(a), is a key parameter to define the ionization state of a compound and directly affects its biopharmaceutical profile. In this study, we developed a novel approach for pK(a) prediction using rooted topological torsion fingerprints in combination with five machine learning (ML) methods: random forest, partial least squares, extreme gradient boosting, lasso regression, and support vector regression. With a large and diverse set of 14 499 experimental pK(a) values, pK(a) models were developed for aliphatic amines. The models demonstrated consistently good prediction statistics and were able to generate accurate prospective predictions as validated with an external test set of 726 pK(a) values (RMSE 0.45, MAE 0.33, and R-2 0.84 by the top model). The factors that may affect prediction accuracy and model applicability were carefully assessed. The results demonstrated that rooted topological torsion fingerprints coupled with ML methods provide a promising approach for developing accurate pK(a) prediction models.
MoreTranslated text
Key words
rooted topological torsion fingerprints,aliphatic amines,machine learning methods,machine learning
AI Read Science
Must-Reading Tree
Example
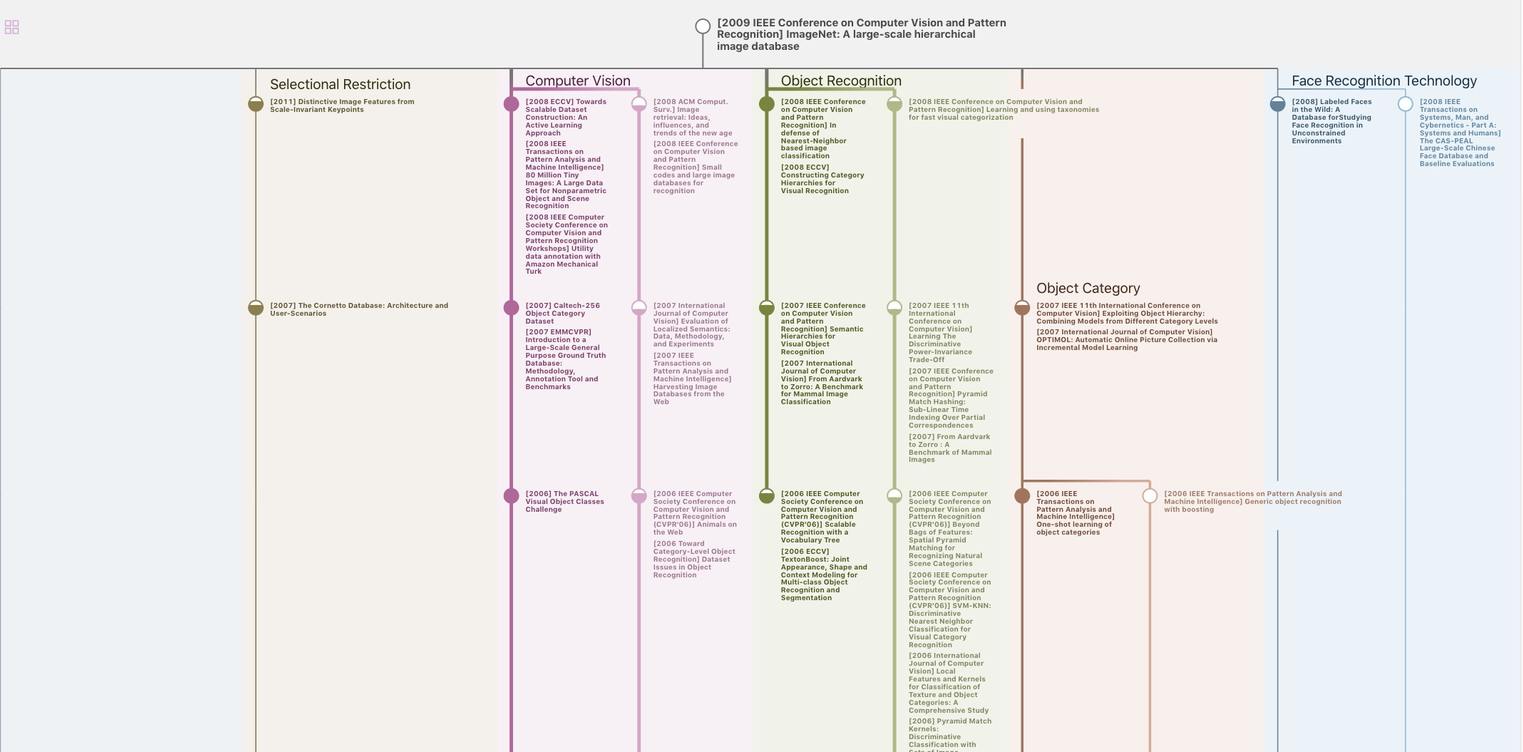
Generate MRT to find the research sequence of this paper
Chat Paper
Summary is being generated by the instructions you defined