Differential regulatory network-based quantification and prioritization of key genes underlying cancer drug resistance based on time-course RNA-seq data.
PLOS COMPUTATIONAL BIOLOGY(2019)
摘要
Drug resistance is a major cause for the failure of cancer chemotherapy or targeted therapy. However, the molecular regulatory mechanisms controlling the dynamic evolvement of drug resistance remain poorly understood. Thus, it is important to develop methods for identifying key gene regulatory mechanisms of the resistance to specific drugs. In this study, we developed a data-driven computational framework, DryNetMC, using a differential regulatory network-based modeling and characterization strategy to quantify and prioritize key genes underlying cancer drug resistance. The DryNetMC does not only infer gene regulatory networks (GRNs) via an integrated approach, but also characterizes and quantifies dynamical network properties for measuring node importance. We used time-course RNA-seq data from glioma cells treated with dbcAMP (a cAMP activator) as a realistic case to reconstruct the GRNs for sensitive and resistant cells. Based on a novel node importance index that comprehensively quantifies network topology, network entropy and expression dynamics, the top ranked genes were verified to be predictive of the drug sensitivities of different glioma cell lines, in comparison with other existing methods. The proposed method provides a quantitative approach to gain insights into the dynamic adaptation and regulatory mechanisms of cancer drug resistance and sheds light on the design of novel biomarkers or targets for predicting or overcoming drug resistance. Author summary Leveraging global gene expression patterns to study dynamical mechanisms of cancer drug resistance is an appealing yet challenging task for both experimental and computational biologists. In this study, we proposed a dynamic network-based computational method to prioritize the key genes responsible for cancer drug resistance, which is significantly innovative compared to the conventional differential expression method and co-expression network-based differential analysis. In addition, our method is verified to be more accurate compared with several state-of-the-art methods used for inferring GRNs. We applied our computational method to a set of time-course RNA-seq data of gliomas, and several novel predictions were verified by the additional gene expression/cellular response data or the clinical data. Our study provides a principled approach to gain insights into dynamic adaptation and regulatory network mechanisms of cancer drug resistance, and sheds lights on designing new biomarkers or targets for predicting or controlling drug resistance.
更多查看译文
AI 理解论文
溯源树
样例
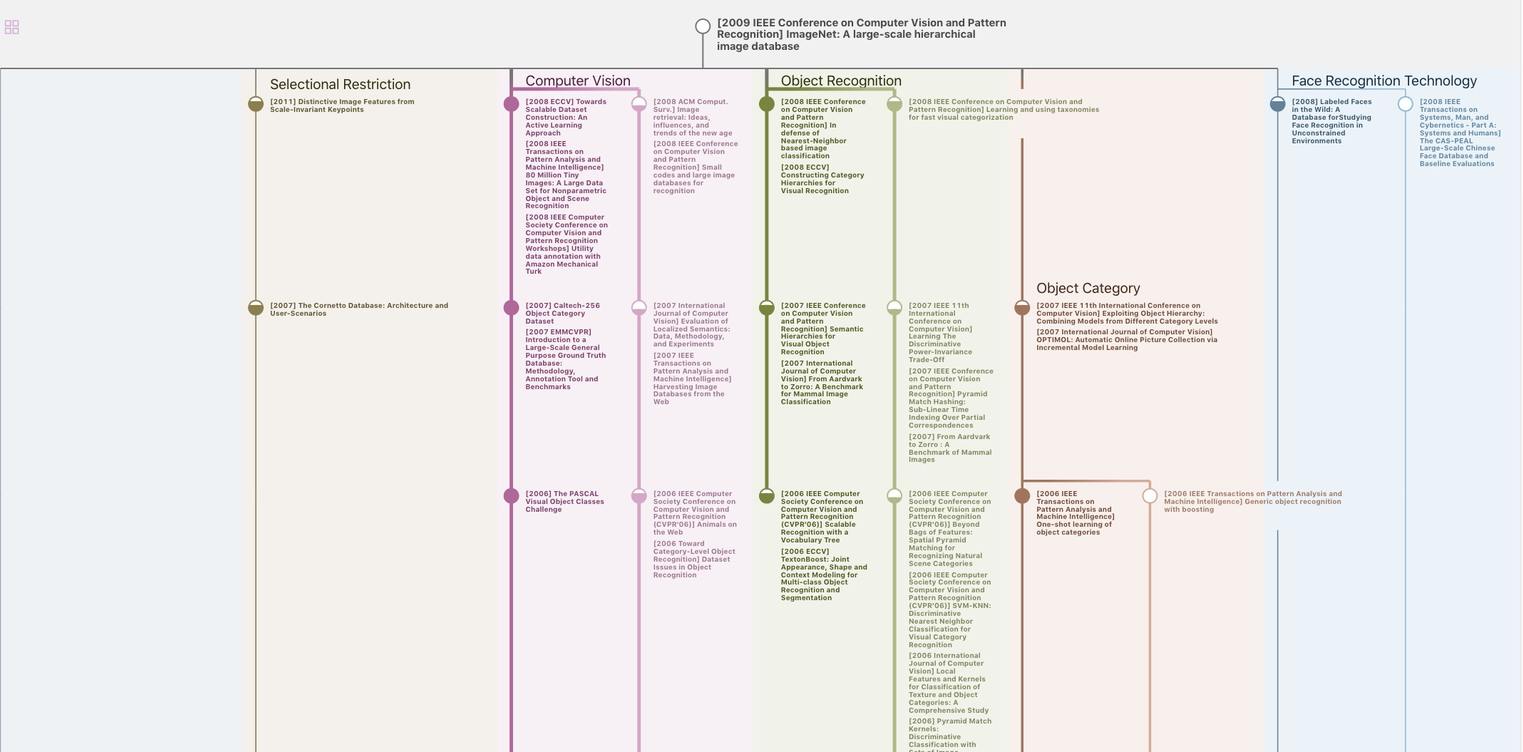
生成溯源树,研究论文发展脉络
Chat Paper
正在生成论文摘要