Resilient Load Restoration in Microgrids Considering Mobile Energy Storage Fleets: A Deep Reinforcement Learning Approach
2020 IEEE POWER & ENERGY SOCIETY GENERAL MEETING (PESGM)(2020)
摘要
Mobile energy storage systems (MESSs) provide mobility and flexibility to enhance distribution system resilience. The paper proposes a Markov decision process (MDP) formulation for an integrated service restoration strategy that coordinates the scheduling of MESSs and resource dispatching of microgrids. The uncertainties in load consumption are taken into account. The deep reinforcement learning (DRL) algorithm is utilized to solve the MDP for optimal scheduling. Specifically, the twin delayed deep deterministic policy gradient (TD3) is applied to train the deep Q-network and policy network, then the well trained policy can be deployed in on-line manner to perform multiple actions simultaneously. The proposed model is demonstrated on an integrated test system with three microgrids connected by Sioux Falls transportation network. The simulation results indicate that mobile and stationary energy resources can be well coordinated to improve system resilience.
更多查看译文
关键词
Microgrid,mobile energy storage,fleet management,deep reinforcement learning,scheduling,resilience
AI 理解论文
溯源树
样例
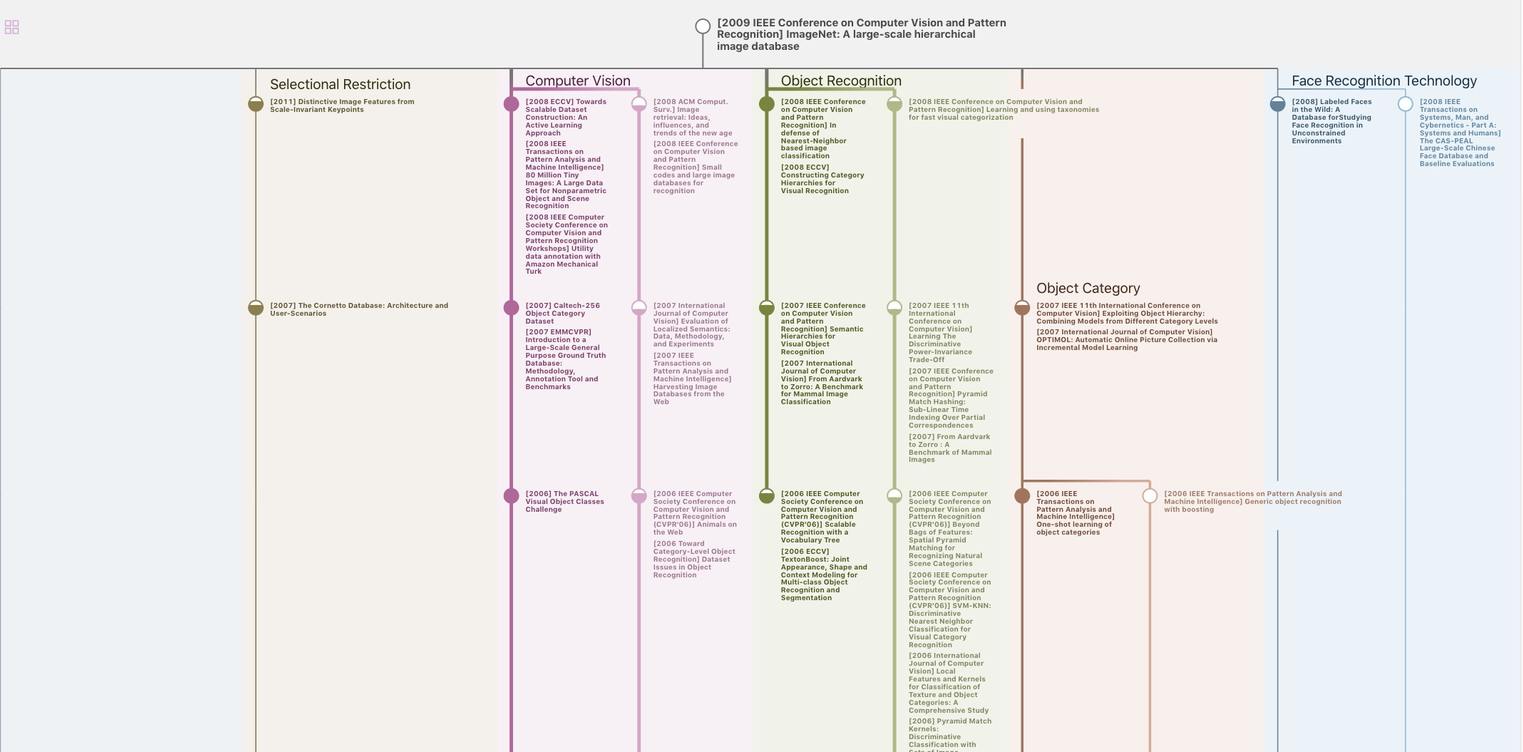
生成溯源树,研究论文发展脉络
Chat Paper
正在生成论文摘要