An efficient mobile-edge collaborative system for video photorealistic style transfer.
SEC(2019)
摘要
In the past decade, convolutional neural networks (CNNs) have achieved great practical success in image transformation tasks, including style transfer, semantic segmentation, etc. CNN-based style transfer, which denotes transforming an image into a desired output image according to a user-specified style image, is one of the most popular techniques in image transformation. It has led to to many successful industrial applications with significant commercial impacts, such as Prisma and DeepArt. Figure 1 shows the general workflow of the CNN-based style transfer. Given a content image and a user-specified style image, the content features and style features can be extracted using a pre-trained CNN, and then be merged to generate the stylized image. The CNN model is trained for generating a stylized image that has similar content features as the content image's and similar style features as the style image's. In this example, we can see the content image is captured at a lake in the daytime, while the style image is another similar scene captured at dusk. After performing style transfer, the content image is successfully transformed to the dusky scene while keeping the content unchanged as the content image.
更多查看译文
AI 理解论文
溯源树
样例
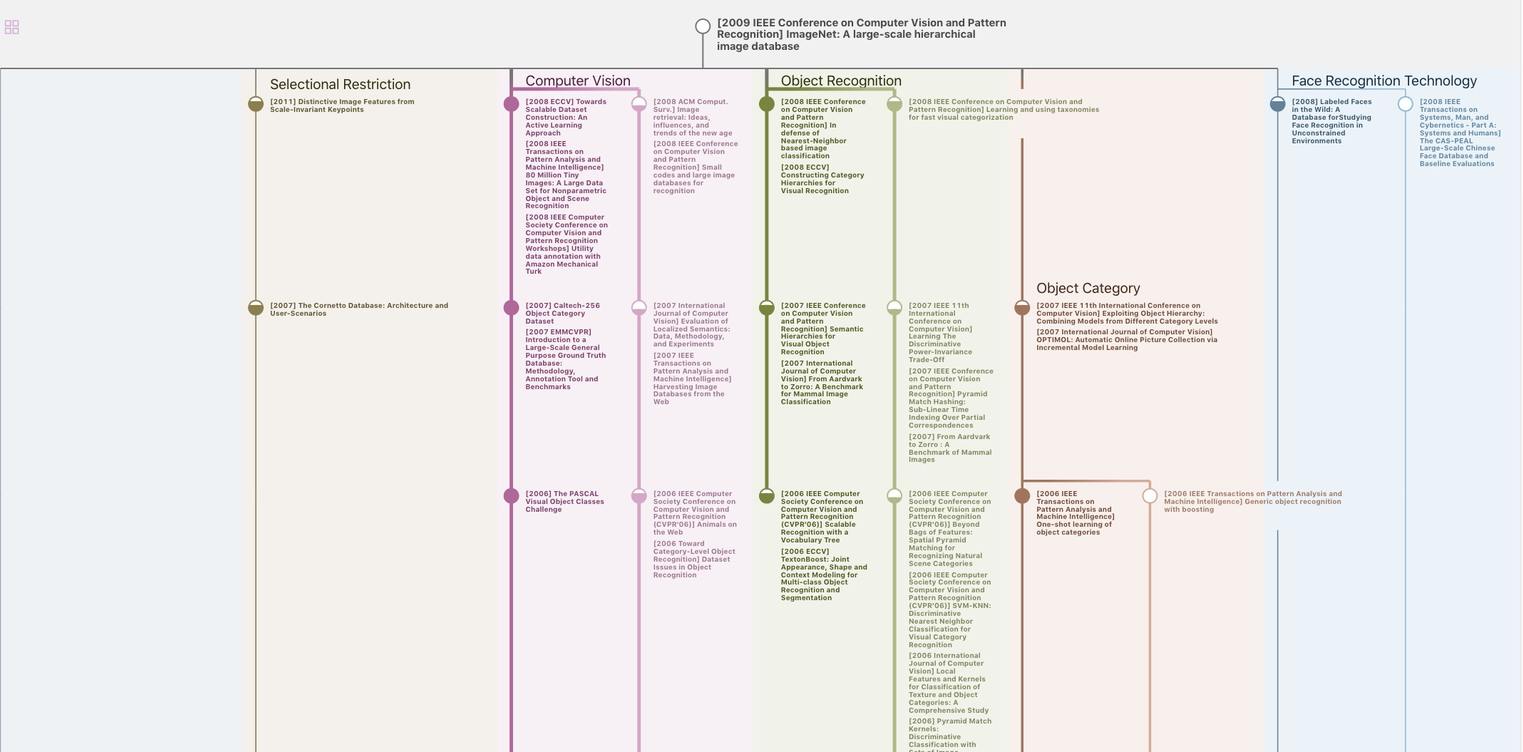
生成溯源树,研究论文发展脉络
Chat Paper
正在生成论文摘要