LASER: Linear Compression in Wireless Distributed Optimization
CoRR(2023)
摘要
Data-parallel SGD is the de facto algorithm for distributed optimization, especially for large scale machine learning. Despite its merits, communication bottleneck is one of its persistent issues. Most compression schemes to alleviate this either assume noiseless communication links, or fail to achieve good performance on practical tasks. In this paper, we close this gap and introduce LASER: LineAr CompreSsion in WirEless DistRibuted Optimization. LASER capitalizes on the inherent low-rank structure of gradients and transmits them efficiently over the noisy channels. Whilst enjoying theoretical guarantees similar to those of the classical SGD, LASER shows consistent gains over baselines on a variety of practical benchmarks. In particular, it outperforms the state-of-the-art compression schemes on challenging computer vision and GPT language modeling tasks. On the latter, we obtain $50$-$64 \%$ improvement in perplexity over our baselines for noisy channels.
更多查看译文
关键词
linear compression,optimization,distributed,laser
AI 理解论文
溯源树
样例
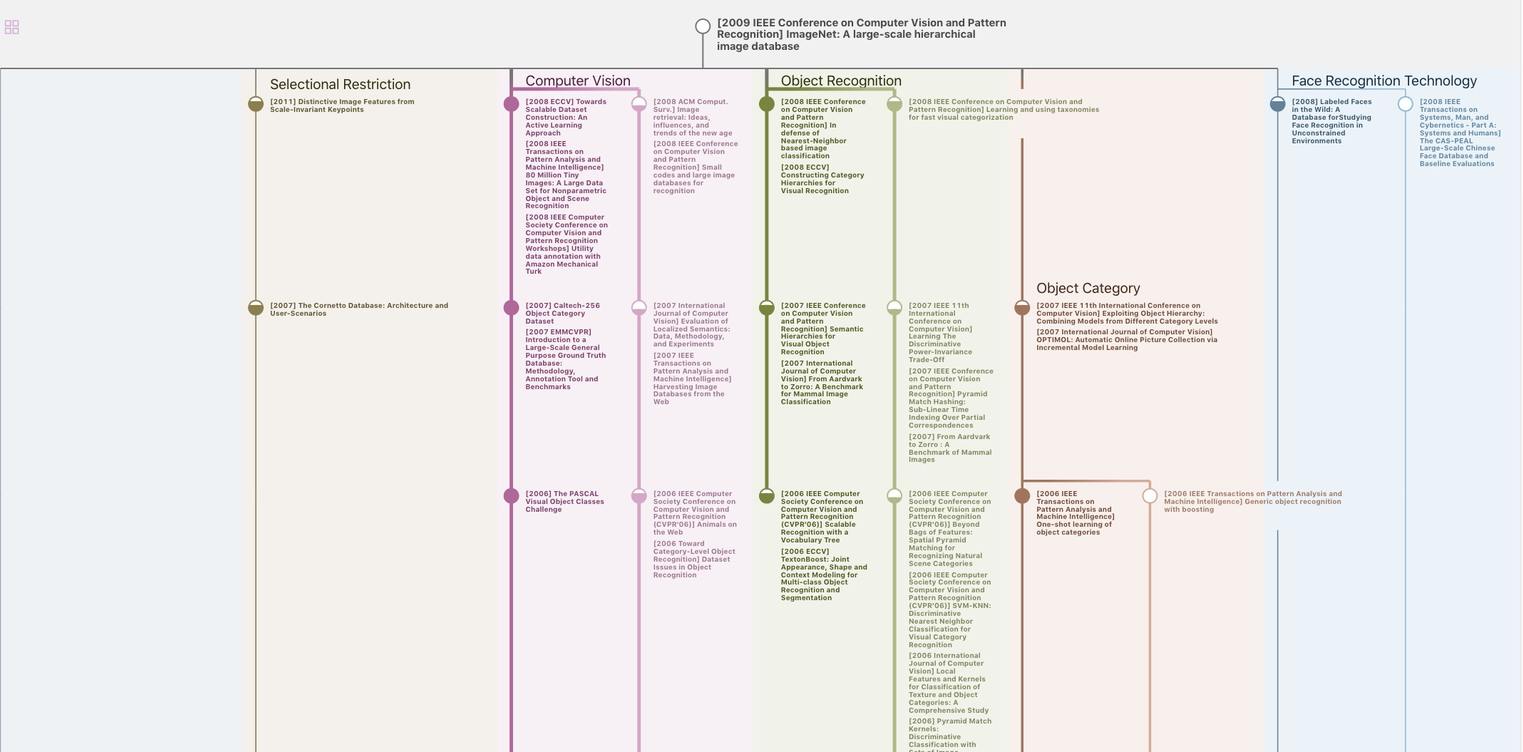
生成溯源树,研究论文发展脉络
Chat Paper
正在生成论文摘要