Edge-Assisted Adaptive Video Streaming With Deep Learning In Mobile Edge Networks
2019 IEEE WIRELESS COMMUNICATIONS AND NETWORKING CONFERENCE (WCNC)(2019)
摘要
Most HTTP Adaptive Streaming (HAS) video content delivery solutions today are governed by purely client-based logics. For lack of coordination among clients and awareness of the dynamic Radio Access Network (RAN) conditions, these approaches may lead to suboptimal user experience and underutilization of network resources. Recently, Mobile Edge Computing (MEC) has been studied as a new networking paradigm to play a part in the adaptive video streaming process with lower endto-end latency and better network awareness. In this paper, we present an edge-assisted adaptive video streaming scheme with deep Q-learning techniques and bandwidth sharing policies, which performs video adaptation according to the real-time radio and network information collected at the network edge. The adaptation scheme is implemented as an edge application hosted on our own deployed MEC server in a real LTE network testbed for experimental evaluation against three popular client-based solutions, namely Buffer-Based Adaptation (BBA), Rate-Based Adaptation (RBA) and adaptation performed by dash.js with its default adaptation logic. To the best of our knowledge, it is one of the few deep Q-learning adaptive video streaming solutions that have been deployed in the MEC framework at network edge in practice. Experiment results show that our proposed scheme outperforms the other three client-based solutions with higher Quality of Experience (QoE) and fairness in the evaluated network environments.
更多查看译文
关键词
HAS, MEC, deep Q-learning
AI 理解论文
溯源树
样例
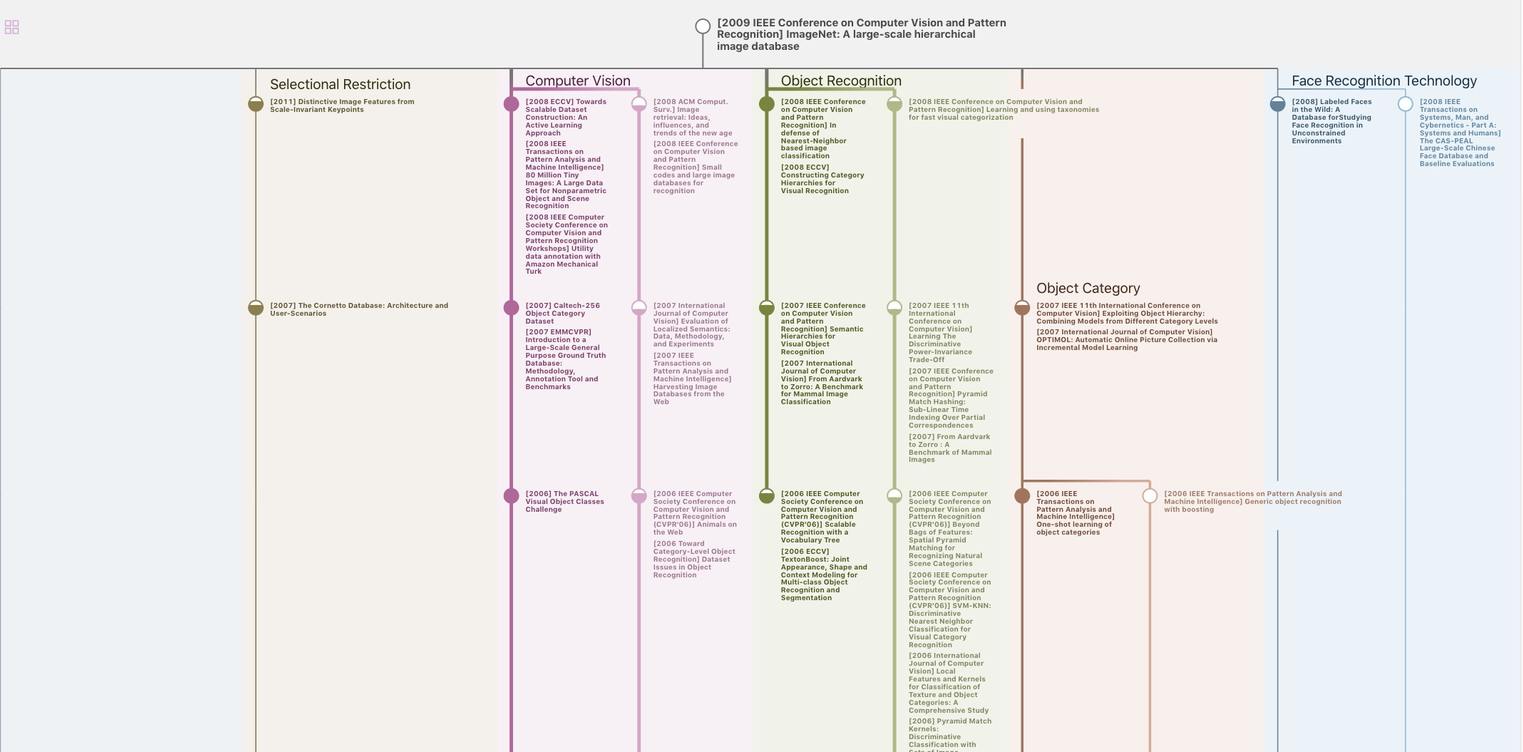
生成溯源树,研究论文发展脉络
Chat Paper
正在生成论文摘要