Multi-Objective Genetic Programming Based Automatic Modulation Classification
2019 IEEE WIRELESS COMMUNICATIONS AND NETWORKING CONFERENCE (WCNC)(2019)
摘要
Automatic modulation classification (AMC) plays a crucial role in the cognitive radio networks, to which feature-based (FB) methods are the dominating solutions. However, the original features in FB methods are redundant, leading to the ambiguity of classification. To tackle this problem, this paper proposes a novel multi-objective modulation classification (MOMC) method. To reduce the redundant features, the original multi-features are recombined into a single feature by multi-objective genetic programming (MOGP) algorithm. Two quantitative objectives, the classification error rate and the variance for robustness, are then presented to jointly optimize the algorithm as two fitness functions. Furthermore, the single feature generated by MOGP is classified by logistic regression (LR) with low computational complexity. Simulation results verify the enhanced robustness and classification accuracy performance yielded by our proposed MOMC method compared to the existing classification methods.
更多查看译文
关键词
automatic modulation classification,cognitive radio networks,feature-based methods,FB methods,multiobjective genetic programming algorithm,quantitative objectives,classification error rate,classification accuracy performance,MOMC method,multiobjective modulation classification method,fitness functions,logistic regression,computational complexity
AI 理解论文
溯源树
样例
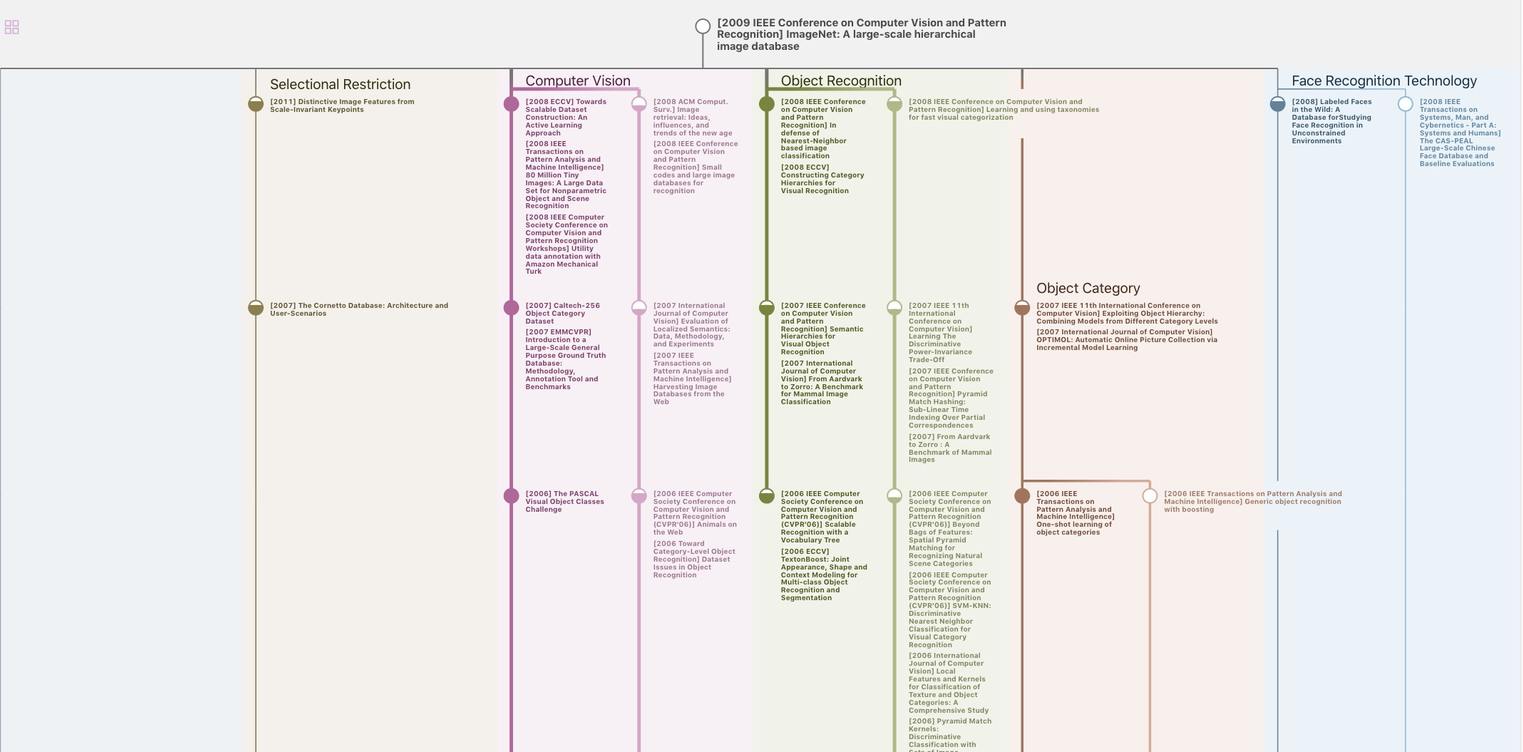
生成溯源树,研究论文发展脉络
Chat Paper
正在生成论文摘要