SWaP - Probabilistic Graphical and Deep Learning Models for Water Consumption Prediction.
BuildSys '19: The 6th ACM International Conference on Systems for Energy-Efficient Buildings, Cities, and Transportation New York NY USA November, 2019(2019)
摘要
Accurately predicting water consumption in residential and commercial buildings is essential for identifying possible leaks, minimizing water wastage, and for paving the way for a sustainable future. In this paper, we present SWaP, a Smart Water Prediction system that predicts future hourly water consumption based on historical data. To perform this prediction task, in SWaP, we design discriminative probabilistic graphical and deep learning models, in particular, sparse Gaussian Conditional Random Fields (GCRFs) and Long Short Term Memory (LSTM) based deep Recurrent Neural Network (RNN) models, to successfully encode dependencies in the water consumption data. We evaluate our system on water consumption data collected from multiple buildings in a university campus and demonstrate that both the GCRF and LSTM based deep models are able to accurately predict future hourly water consumption in advance using just the last 24 hours of data at test time. SWaP achieves superior prediction performance for all buildings in comparison to the linear regression and ARIMA baselines in terms of Root Mean Squared Error (RMSE) and Mean Absolute Error (MAE), with the GCRF and LSTM models providing 50% and 44% improvements on average, respectively. We also demonstrate that augmenting our models with temporal features such as time of the day and day of the week can improve the overall average prediction performance. Additionally, based on our evaluation, we observe that the GCRF model outperforms the LSTM based deep learning model, while simultaneously being faster to train and execute at test time. The computationally efficient and interpretable nature of GCRF models in SWaP make them an ideal choice for practical deployment.
更多查看译文
关键词
RNN,Sparse GCRF,Time series modeling
AI 理解论文
溯源树
样例
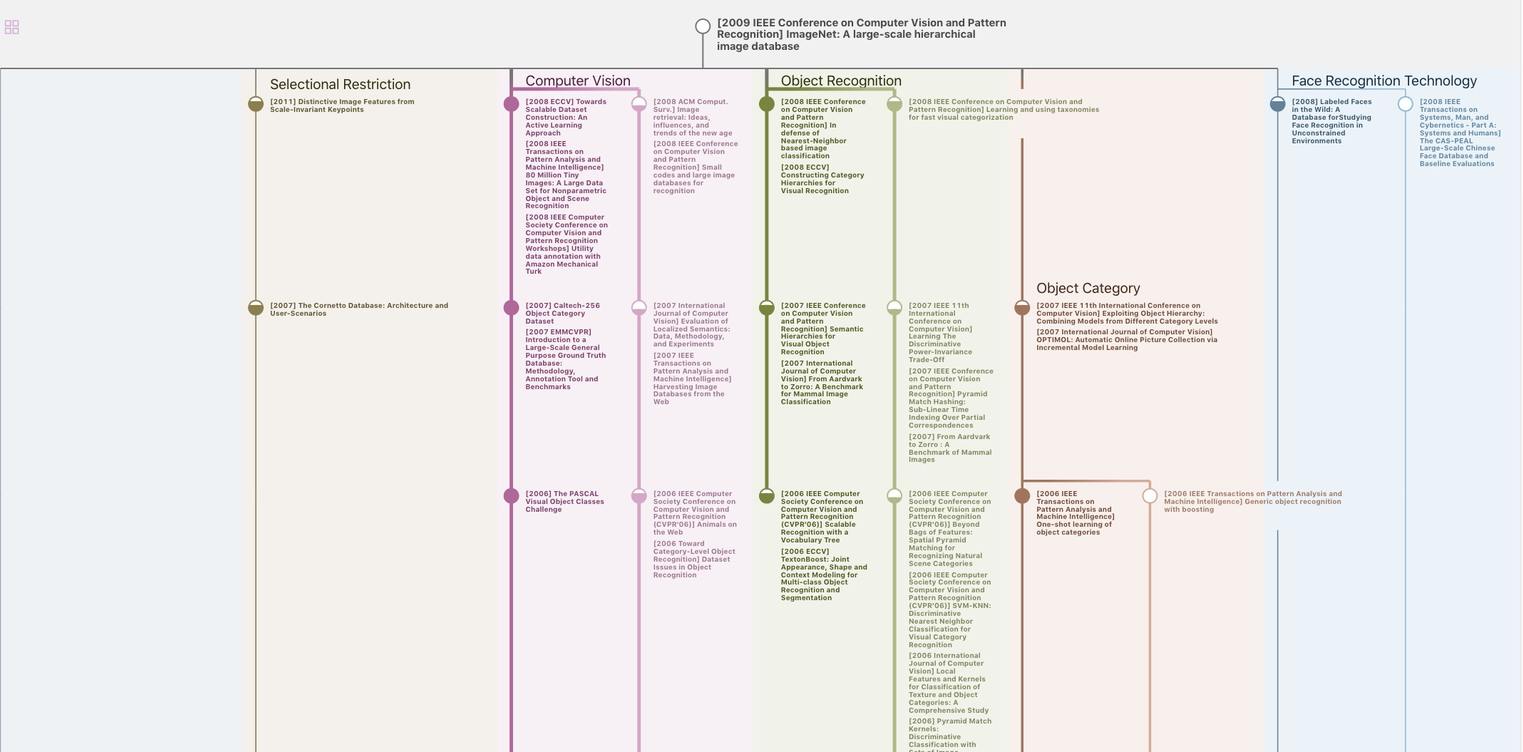
生成溯源树,研究论文发展脉络
Chat Paper
正在生成论文摘要