Learning from Correlated Events for Equipment Relation Inference in Buildings.
BuildSys '19: The 6th ACM International Conference on Systems for Energy-Efficient Buildings, Cities, and Transportation New York NY USA November, 2019(2019)
摘要
Modern buildings produce thousands of data streams, and the ability to automatically infer the physical context of such data is the key to enabling building analytics at scale. As acquiring this contextual information is currently a time-consuming and error-prone manual process, in this study we make the first attempt at automatically inferring one important contextual aspect of the equipment in buildings --- how each equipment is functionally connected with another. The main insight behind our solution is that functionally connected equipment is exposed to the same events in the physical world, creating correlated changes in the time series data of both equipment. Because events are of indeterminate length in time series, however, identifying them requires solving a non-polynomial combinatorial data segmentation problem. We present a solution that first extracts latent events from the sensory time series data, and then sifts out coincident events with a customized correlation procedure to identify the relationship between equipment. We evaluated our approach on data collected from over 1,000 pieces of equipment from 5 commercial buildings of various sizes located in different geographical regions in the US. Results show that this approach achieves 94.38% accuracy in relation inference, compared to 85.49% by the best baseline.
更多查看译文
关键词
Relation inference,smart buildings,time series analysis,event detection,probabilistic modeling
AI 理解论文
溯源树
样例
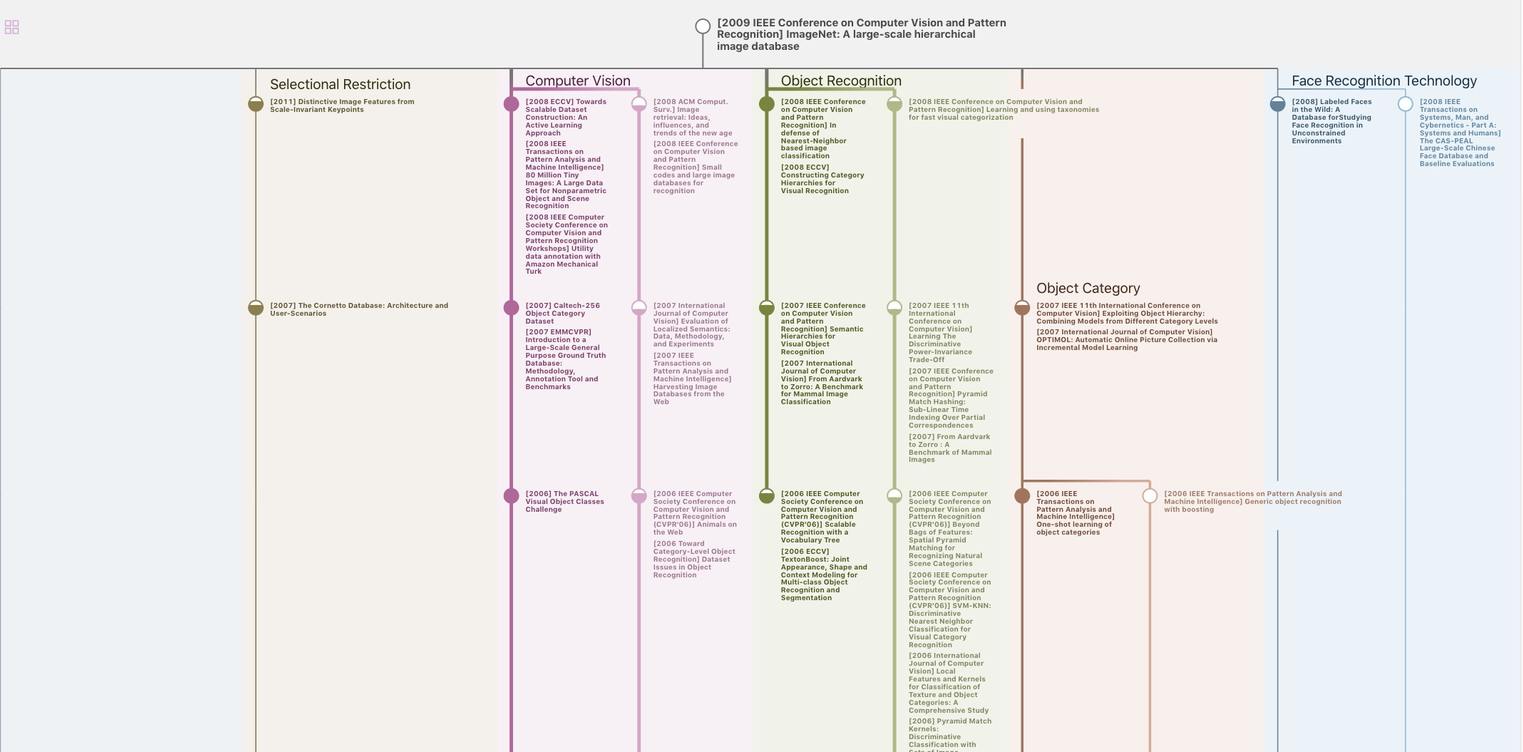
生成溯源树,研究论文发展脉络
Chat Paper
正在生成论文摘要