Meta-Deep Q-Learning for Eco-Routing
2019 IEEE 2nd Connected and Automated Vehicles Symposium (CAVS)(2019)
摘要
In this paper, a multi-objective deep Q-learning (MOM-DQL) method is developed for solving eco- routing problem in a signalized traffic network. The problem is formulated as dynamic multi- objective Markov decision processes (MOMDPs). MOM- DQL can explore the optimal eco-routes with respect to drivers’ different preferences on saving travel time and fuel. The MOM-DQL agent is trained under a series of learning environments that are based on historical vehicle trajectories, fuel consumption, and traffic signal status in the remote data center. The model that represents the action value function of the historical dynamic driving conditions can be downloaded to the vehicle requesting eco-routing service. The model can quickly adapt to the most recent driving condition through online one-shot learning and predict the optimal eco-routes for the subsequent unseen driving condition of the signalized traffic network. Simulation shows that the MOM-DQL method can discover the optimal eco-routes, and saves 52% travel time and 33% fuel, compared to the shortest-path strategy that is widely used in navigation systems.
更多查看译文
关键词
meta-deep Q-learning,multiobjective deep Q-learning method,signalized traffic network,multiobjective Markov decision processes,MOM- DQL,travel time,MOM-DQL agent,historical vehicle trajectories,traffic signal status,historical dynamic driving conditions,eco-routing service,one-shot learning,subsequent unseen driving condition,MOM-DQL method,action value function,eco- routing problem
AI 理解论文
溯源树
样例
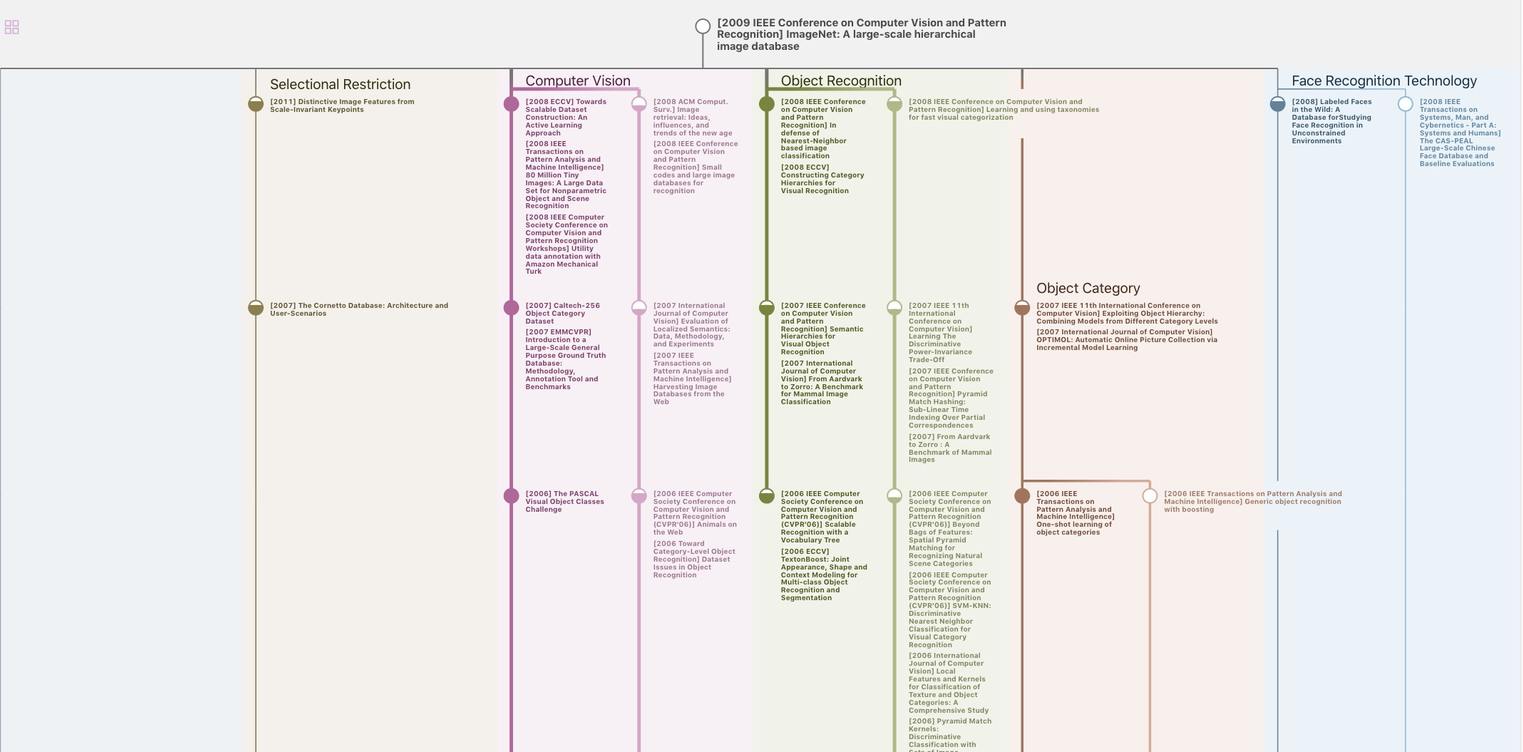
生成溯源树,研究论文发展脉络
Chat Paper
正在生成论文摘要