Self-learning of multivariate time series using perceptually important points
IEEE/CAA Journal of Automatica Sinica(2019)
摘要
In machine learning, positive-unlabelled ( PU ) learning is a special case within semi-supervised learning. In positive-unlabelled learning, the training set contains some positive examples and a set of unlabelled examples from both the positive and negative classes. Positive-unlabelled learning has gained attention in many domains, especially in time-series data, in which the obtainment of labelled data is challenging. Examples which originate from the negative class are especially difficult to acquire. Self-learning is a semi-supervised method capable of PU learning in time-series data. In the self-learning approach, observations are individually added from the unlabelled data into the positive class until a stopping criterion is reached. The model is retrained after each addition with the existent labels. The main problem in self-learning is to know when to stop the learning. There are multiple, different stopping criteria in the literature, but they tend to be inaccurate or challenging to apply. This publication proposes a novel stopping criterion, which is called Peak evaluation using perceptually important points, to address this problem for time-series data. Peak evaluation using perceptually important points is exceptional, as it does not have tunable hyperparameters, which makes it easily applicable to an unsupervised setting. Simultaneously, it is flexible as it does not make any assumptions on the balance of the dataset between the positive and the negative class.
更多查看译文
关键词
Positive-unlabelled (PU) learning,self-learning,stopping criterion,time series
AI 理解论文
溯源树
样例
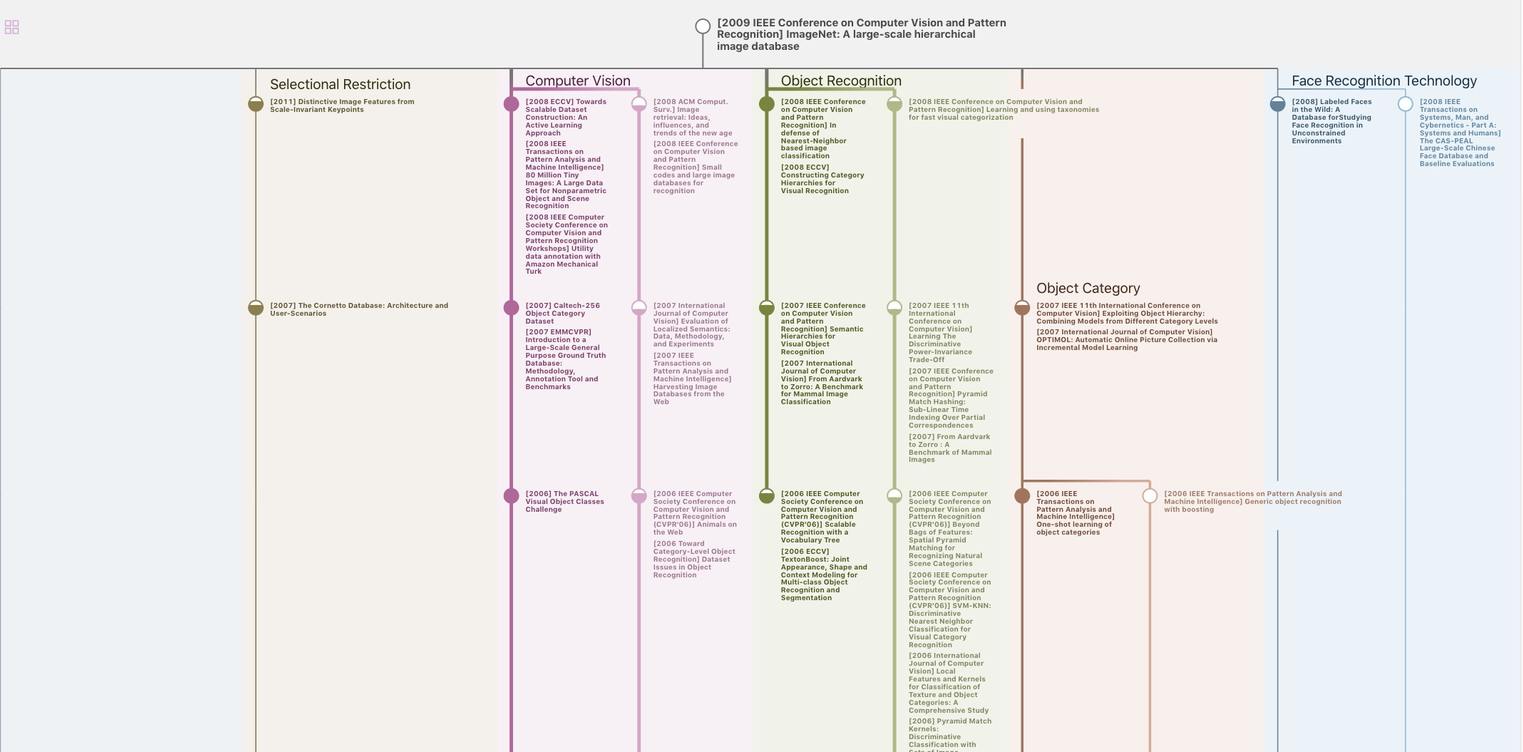
生成溯源树,研究论文发展脉络
Chat Paper
正在生成论文摘要