Deep learning analyses of synthetic spectral libraries with an application to the Gaia-ESO database
arxiv(2019)
摘要
In the era of stellar spectroscopic surveys, synthetic spectral libraries will form the basis for the derivation of the stellar parameters and chemical abundances. In this paper, four popular synthetic grids (INTRIGOSS, FERRE, AMBRE, and PHOENIX) are used in our deep learning prediction framework (StarNet), and compared in an application to optical spectra from the Gaia-ESO survey. The stellar parameters for temperature, surface gravity, metallicity, radial velocity, rotational velocity, and [{\alpha}/Fe] are determined simultaneously for FGK type dwarfs and giants. StarNet was modified to mitigate the differences in the sampling between the synthetic grids and the observed spectra, by augmenting the grids with realistic observational signatures, in an attempt to incorporate both modelling and statistical uncertainties as part of the training. When applied to spectra from the Gaia-ESO spectroscopic survey and the Gaia-ESO benchmark stars, the INTRIGOSS-trained StarNet showed the best results with the least scatter. Training with the FERRE synthetic grid produces similarly accurate predictions (followed closely by the AMBRE grid), but over a wider range in stellar parameters and spectroscopic wavelengths . In the future, improvements in the underlying physics that generates these synthetic grids will be necessary for consistent high precision stellar parameters and chemical abundances from machine learning and other sophisticated data analysis tools.
更多查看译文
AI 理解论文
溯源树
样例
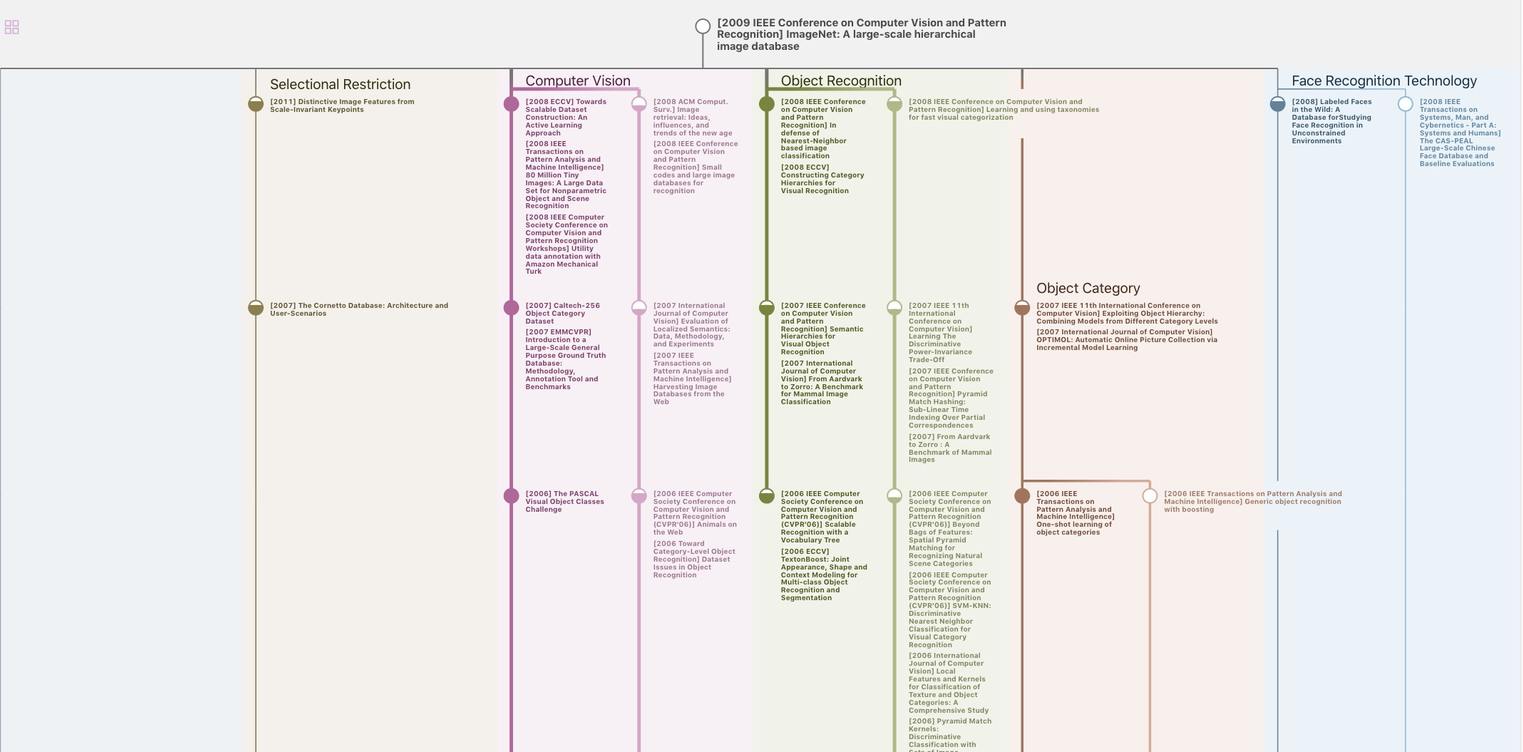
生成溯源树,研究论文发展脉络
Chat Paper
正在生成论文摘要