MERIT: Tensor Transform for Memory-Efficient Vision Processing on Parallel Architectures
IEEE Transactions on Very Large Scale Integration (VLSI) Systems(2020)
摘要
Computationally intensive deep neural networks (DNNs) are well- suited to run on GPUs, but newly developed algorithms usually require the heavily optimized DNN routines to work efficiently, and this problem could be even more difficult for specialized DNN architectures. In this article, we propose a mathematical formulation that can be useful for transferring the algorithm optimization knowledge across computing platforms. We discover that data movement and storage inside parallel processor architectures can be viewed as tensor transforms across memory hierarchies, making it possible to describe many memory optimization techniques mathematically. Such transform, which we call memory-efficient ranged inner-product tensor (MERIT) transform, can be applied to not only DNN tasks but also many traditional machine learning and computer vision computations. Moreover, the tensor transforms can be readily mapped to existing vector processor architectures. In this article, we demonstrate that many popular applications can be converted to a succinct MERIT notation on GPUs, speeding up GPU kernels up to 20 times while using only half as many code tokens. We also use the principle of the proposed transform to design a specialized hardware unit called MERIT-z processor. This processor can be applied to a variety of DNN tasks as well as other computer vision tasks while providing comparable area and power efficiency to dedicated DNN application-specific integrated circuits (ASICs).
更多查看译文
关键词
Transforms,Magneto electrical resistivity imaging technique,Computer architecture,Tensile stress,Task analysis,Graphics processing units,Optimization
AI 理解论文
溯源树
样例
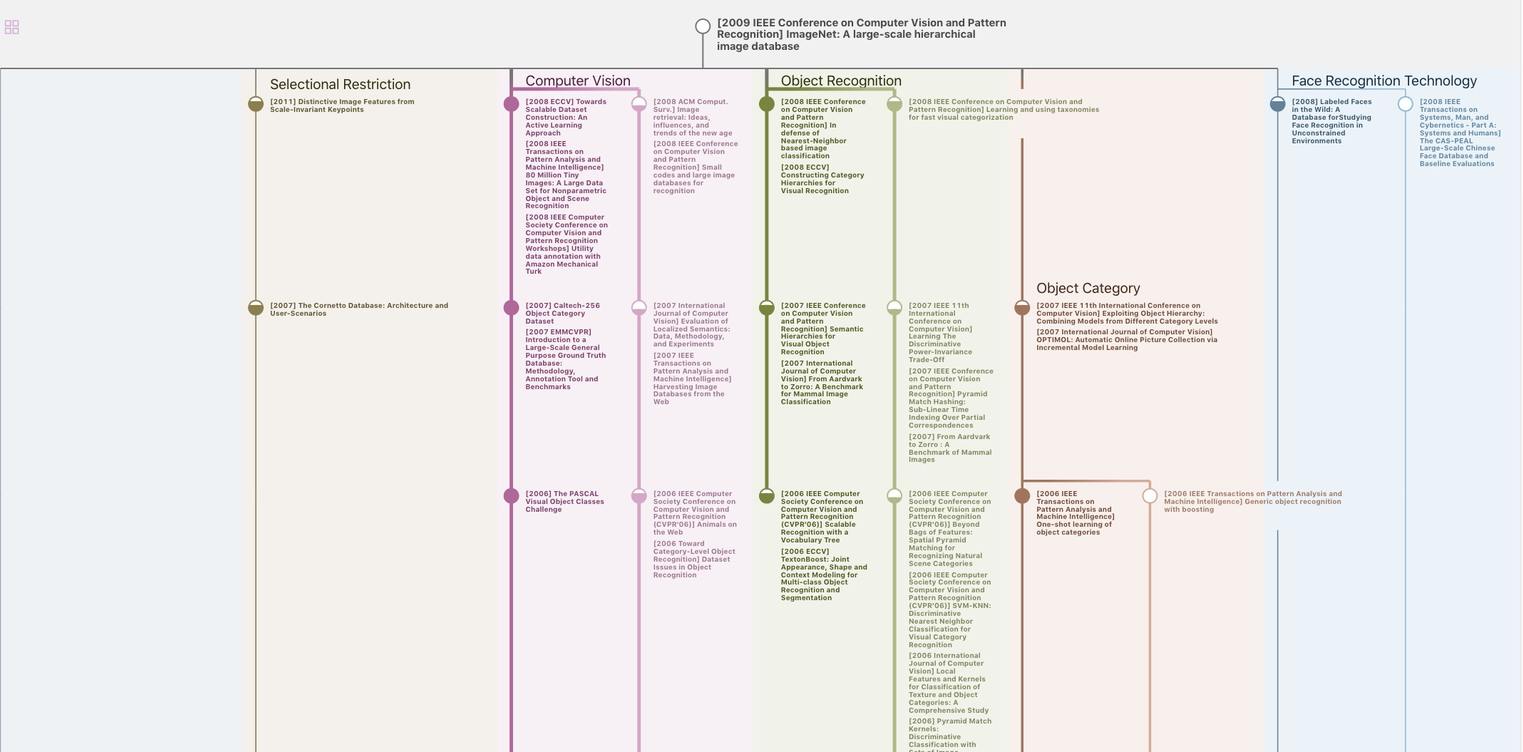
生成溯源树,研究论文发展脉络
Chat Paper
正在生成论文摘要