A Modular Deep Learning Pipeline for Galaxy-Scale Strong Gravitational Lens Detection and Modeling
CoRR(2022)
摘要
Upcoming large astronomical surveys are expected to capture an unprecedented number of strong gravitational lensing systems. Deep learning is emerging as a promising practical tool for the detection and quantification of these galaxy-scale image distortions. The absence of large quantities of representative data from current astronomical surveys motivates the development of a robust forward-modeling approach using synthetic lensing images. Using a mock sample of strong lenses created upon a state-of-the-art extragalactic catalogs, we train a modular deep learning pipeline for uncertainty-quantified detection and modeling with intermediate image processing components for denoising and deblending the lensing systems. We demonstrate a high degree of interpretability and controlled systematics due to domain-specific task modules trained with different stages of synthetic image generation. For lens detection and modeling, we obtain semantically meaningful latent spaces that separate classes of strong lens images and yield uncertainty estimates that explain the origin of misclassified images and provide probabilistic predictions for the lens parameters. Validation of the inference pipeline has been carried out using images from the Subaru telescope's Hyper Suprime-Cam camera, and LSST DESC simulated DC2 sky survey catalogues.
更多查看译文
AI 理解论文
溯源树
样例
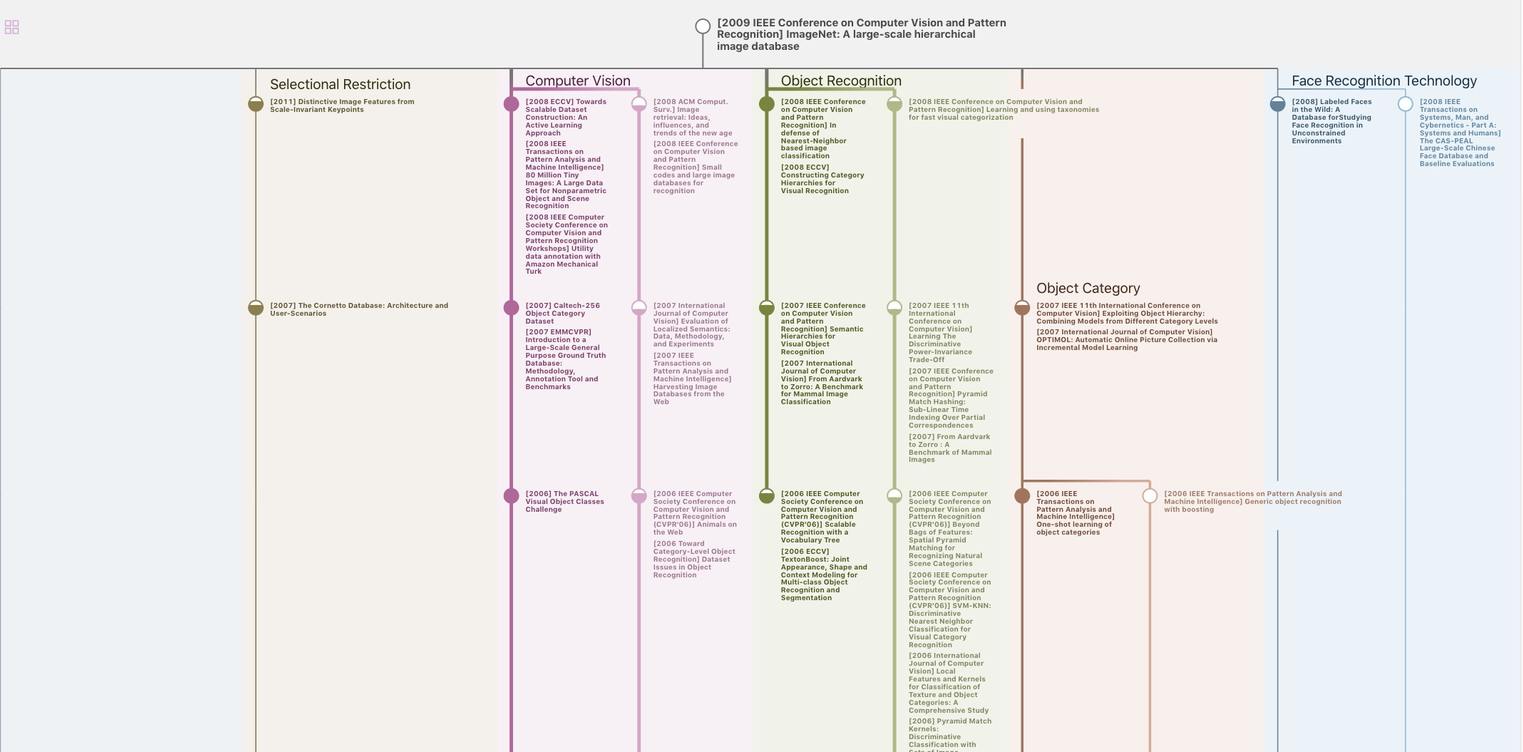
生成溯源树,研究论文发展脉络
Chat Paper
正在生成论文摘要