Fair Adversarial Gradient Tree Boosting
2019 19TH IEEE INTERNATIONAL CONFERENCE ON DATA MINING (ICDM 2019)(2019)
摘要
Fair classification has become an important topic in machine learning research. While most bias mitigation strategies focus on neural networks, we noticed a lack of work on fair classifiers based on decision trees even though they have proven very efficient. In an up-to-date comparison of state-of-the-art classification algorithms in tabular data, tree boosting outperforms deep learning [1]. For this reason, we have developed a novel approach of adversarial gradient tree boosting. The objective of the algorithm is to predict the output Y with gradient tree boosting while minimizing the ability of an adversarial neural network to predict the sensitive attribute S. The approach incorporates at each iteration the gradient of the neural network directly in the gradient tree boosting. We empirically assess our approach on 4 popular data sets and compare against state-of-the-art algorithms. The results show that our algorithm achieves a higher accuracy while obtaining the same level of fairness, as measured using a set of different common fairness definitions.
更多查看译文
关键词
Fair machine learning, Adversarial learning, Gradient Tree Boosting
AI 理解论文
溯源树
样例
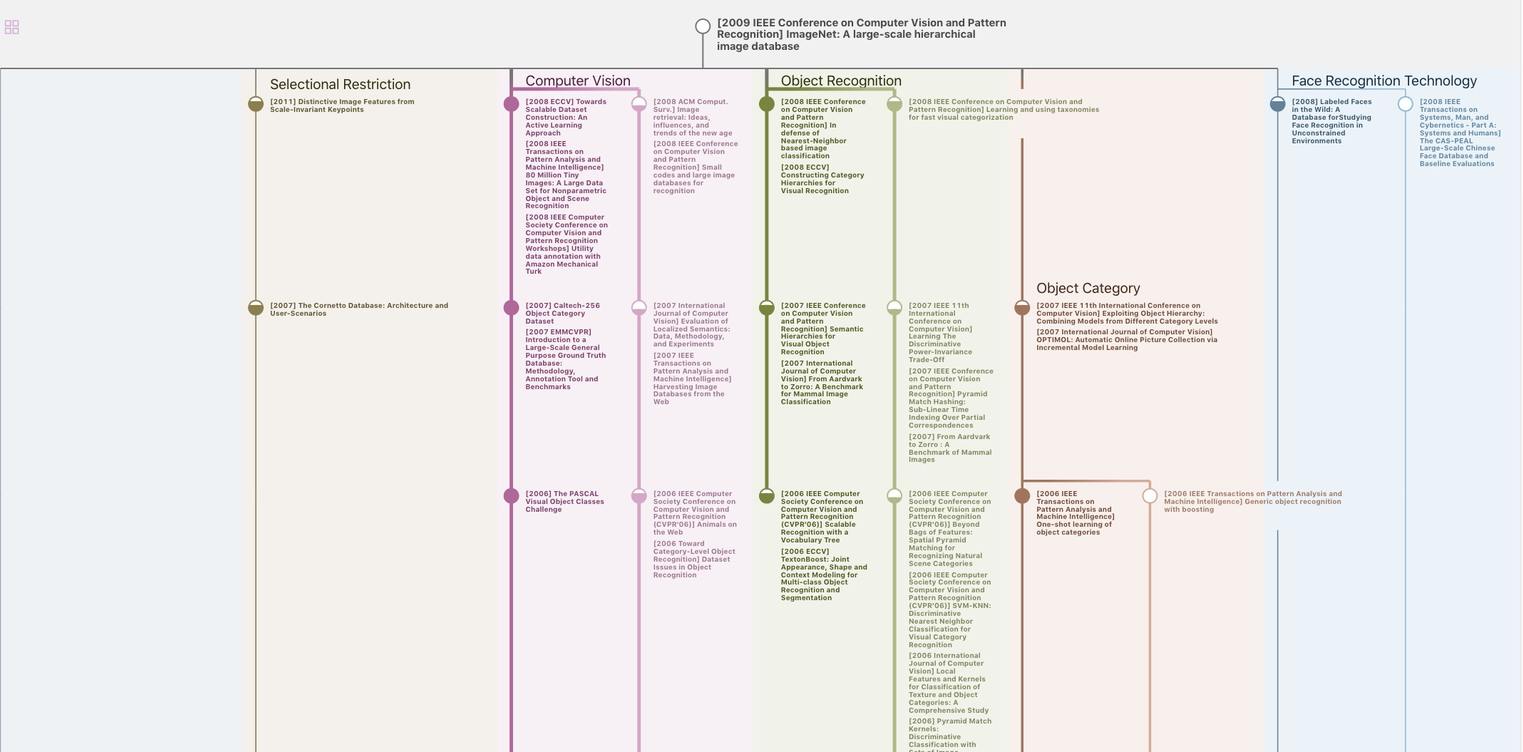
生成溯源树,研究论文发展脉络
Chat Paper
正在生成论文摘要