Squeezing the Last MHz for CNN Acceleration on FPGAs
2019 IEEE International Test Conference in Asia (ITC-Asia)(2019)
摘要
Neural networks especially the convolution neural networks (CNN) have become prevalent and numerous CNN accelerators have been developed to achieve higher performance. While clock frequency determines the operation speed and has direct influence on the performance of the accelerators, we propose to apply overclocking, a circuit optimization approach that enables higher clock frequency, on general CNN accelerators. This technique brings significant performance improvement, but it leads to moderate timing errors, wrong computing results and low prediction accuracy. By taking advantage of the inherent fault tolerance of neural networks, we opt to learn the computing errors together with the application data with additional on-accelerator training. In this case, the resulting models can be resilient to the errors and do not necessarily suffer considerable prediction accuracy loss. In addition, we also take the worst case of overclocking into consideration with a series of approaches ranging from fault detection to fault recovery in case of hardware crash. Finally, we demonstrate the use of overclocking on a CNN accelerator implemented on Xilinx KCU1500 with comprehensive experiments. The experiments show that overclocking in combination with the on-accelerator neural network training improves both the neural network performance and energy efficiency with small prediction accuracy loss.
更多查看译文
关键词
Overclocking,On accelerator training,CNN acceleration,Energy efficient
AI 理解论文
溯源树
样例
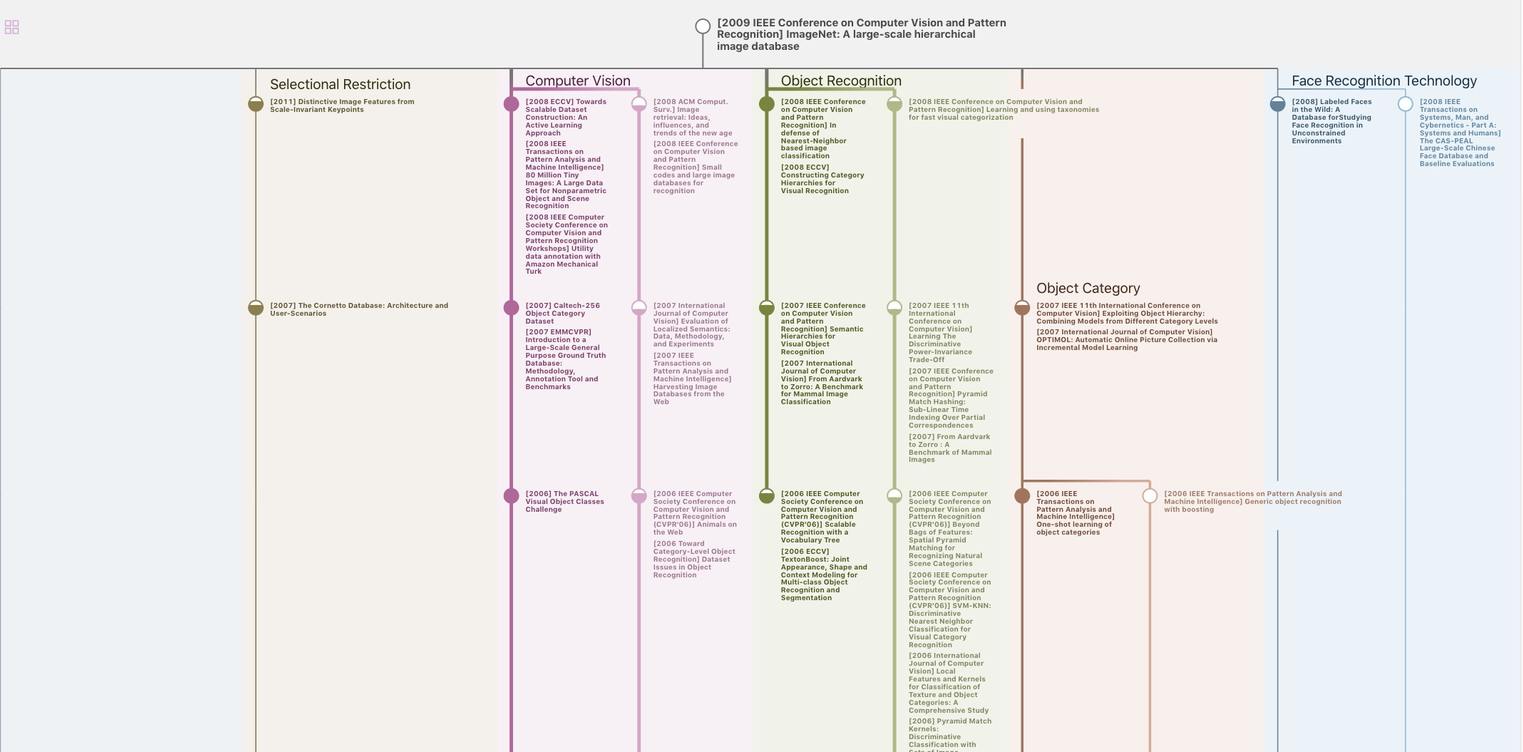
生成溯源树,研究论文发展脉络
Chat Paper
正在生成论文摘要