Maximum Correntropy Criterion-Based Robust Semisupervised Concept Factorization for Image Representation
IEEE Transactions on Neural Networks and Learning Systems(2020)
摘要
Concept factorization (CF) has shown its great advantage for both clustering and data representation and is particularly useful for image representation. Compared with nonnegative matrix factorization (NMF), CF can be applied to data containing negative values. However, the performance of CF method and its extensions will degenerate a lot due to the negative effects of outliers, and CF is an unsupervised method that cannot incorporate label information. In this article, we propose a novel CF method, with a novel model built based on the maximum correntropy criterion (MCC). In order to capture the local geometry information of data, our method integrates the robust adaptive embedding and CF into a unified framework. The label information is utilized in the adaptive learning process. Furthermore, an iterative strategy based on the accelerated block coordinate update is proposed. The convergence property of the proposed method is analyzed to ensure that the algorithm converges to a reliable solution. The experimental results on four real-world image data sets show that the new method can almost always filter out the negative effects of the outliers and outperform several state-of-the-art image representation methods.
更多查看译文
关键词
Data models,Image representation,Convergence,Task analysis,Principal component analysis,Analytical models,Learning systems
AI 理解论文
溯源树
样例
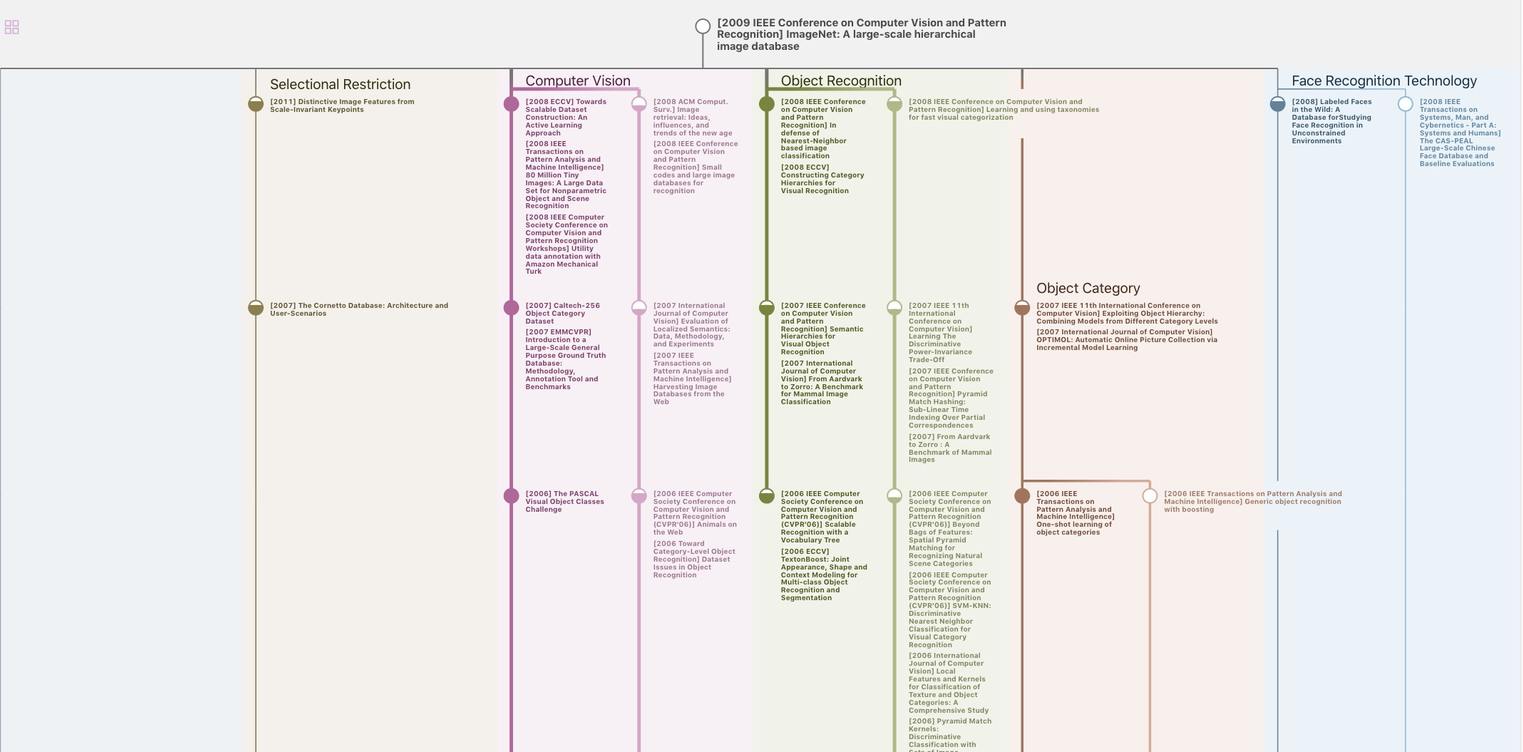
生成溯源树,研究论文发展脉络
Chat Paper
正在生成论文摘要