Forecasting Ontario Oncology Drug Expenditures: A Hybrid Approach to Improving Accuracy
Applied Health Economics and Health Policy(2019)
摘要
Background The Provincial Drug Reimbursement Program (PDRP) at Cancer Care Ontario (CCO) is responsible for monitoring actual and projected outpatient intravenous cancer drug spending in the province. We developed a hybrid forecasting approach combining automated time-series forecasting with expert-customizable input. Objective Our objectives were to provide a flexible tool in which to incorporate multiple forecasts and to improve the accuracy of the resulting forecast. Methods The approach employed linear and non-linear time-series techniques and a combined hybrid model incorporating both approaches. We developed an interactive tool that incorporated the statistical models and identified the best performing forecast according to standard goodness-of-fit measures. Model selection procedures considered both the amount of historical expenditure data available per drug policy and the individual policy contributions to the overall budget. The user was allowed to customize forecasts based on knowledge of external factors related to policy or price changes and new drugs that come to market Results A comparison of 2016/17 fiscal year expenditures showed that all policies with a significant contribution to the overall budget were forecast with < 4% error. Forecasting error was reduced by at least $Can5 million for the nine most expensive policies compared with expert opinion. This approach to drug budget forecasting was implemented in Ontario for the first time in the 2017/18 fiscal year, where 1% error was observed for the overall budget, corresponding to an overestimate of expenditures by $Can3.0 million. Conclusion We introduced a pragmatic approach for regular forecasting by budget holders in Ontario. Our approach to isolating ‘big budget’ from ‘small budget’ drugs using an 80-20 rule and providing multiple forecasts depending on the length of the drug expenditure histories is transferable to other jurisdictions.
更多查看译文
AI 理解论文
溯源树
样例
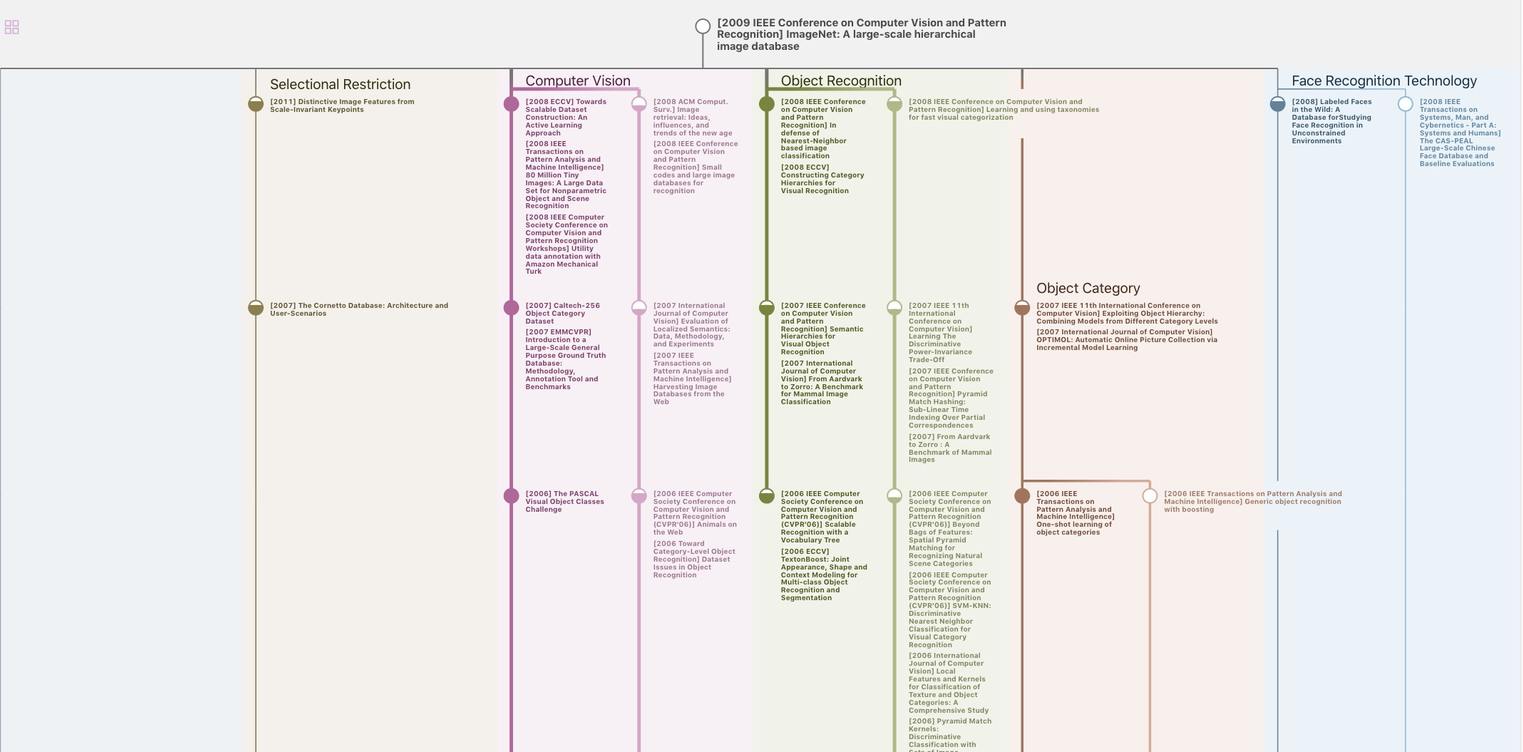
生成溯源树,研究论文发展脉络
Chat Paper
正在生成论文摘要