Nonpeaked Discriminant Analysis for Data Representation.
IEEE transactions on neural networks and learning systems(2019)
摘要
Of late, there are many studies on the robust discriminant analysis, which adopt L
1
-norm as the distance metric, but their results are not robust enough to gain universal acceptance. To overcome this problem, the authors of this article present a nonpeaked discriminant analysis (NPDA) technique, in which cutting L
1
-norm is adopted as the distance metric. As this kind of norm can better eliminate heavy outliers in learning models, the proposed algorithm is expected to be stronger in performing feature extraction tasks for data representation than the existing robust discriminant analysis techniques, which are based on the L
1
-norm distance metric. The authors also present a comprehensive analysis to show that cutting L
1
-norm distance can be computed equally well, using the difference between two special convex functions. Against this background, an efficient iterative algorithm is designed for the optimization of the proposed objective. Theoretical proofs on the convergence of the algorithm are also presented. Theoretical insights and effectiveness of the proposed method are validated by experimental tests on several real data sets.
更多查看译文
关键词
Cutting L₁-norm distance,data classification,discriminant analysis,robustness
AI 理解论文
溯源树
样例
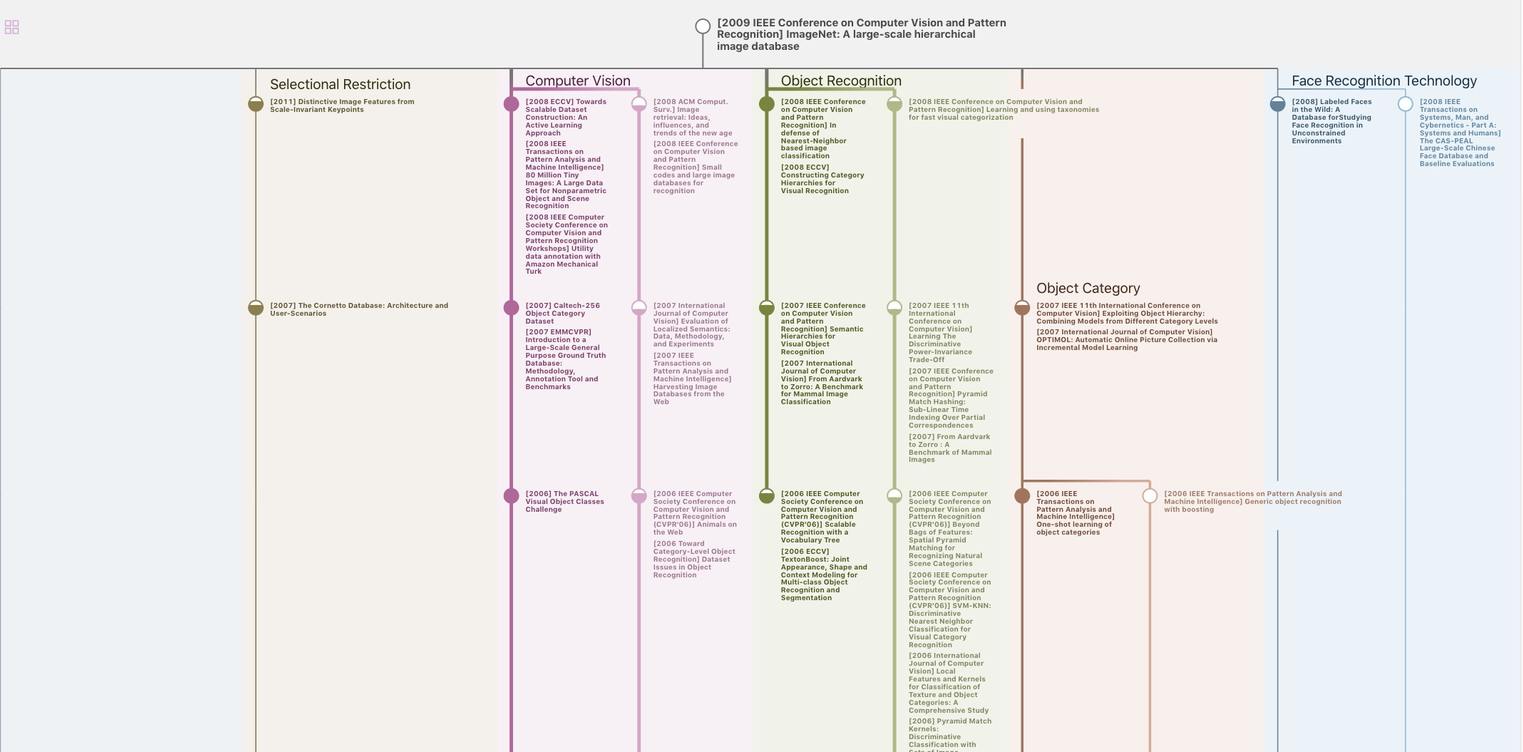
生成溯源树,研究论文发展脉络
Chat Paper
正在生成论文摘要