Compressing Deep Neural Networks With Sparse Matrix Factorization
IEEE Transactions on Neural Networks and Learning Systems(2020)
摘要
Modern deep neural networks (DNNs) are usually overparameterized and composed of a large number of learnable parameters. One of a few effective solutions attempts to compress DNN models via learning sparse weights and connections. In this article, we follow this line of research and present an alternative framework of learning sparse DNNs, with the assistance of matrix factorization. We provide an underlying principle for substituting the original parameter matrices with the multiplications of highly sparse ones, which constitutes the theoretical basis of our method. Experimental results demonstrate that our method substantially outperforms previous states of the arts for compressing various DNNs, giving rich empirical evidence in support of its effectiveness. It is also worth mentioning that, unlike many other works that focus on feedforward networks like multi-layer perceptrons and convolutional neural networks only, we also evaluate our method on a series of recurrent networks in practice.
更多查看译文
关键词
Sparse matrices,Matrices,Indexes,Neural networks,Task analysis,Bayes methods,Learning systems
AI 理解论文
溯源树
样例
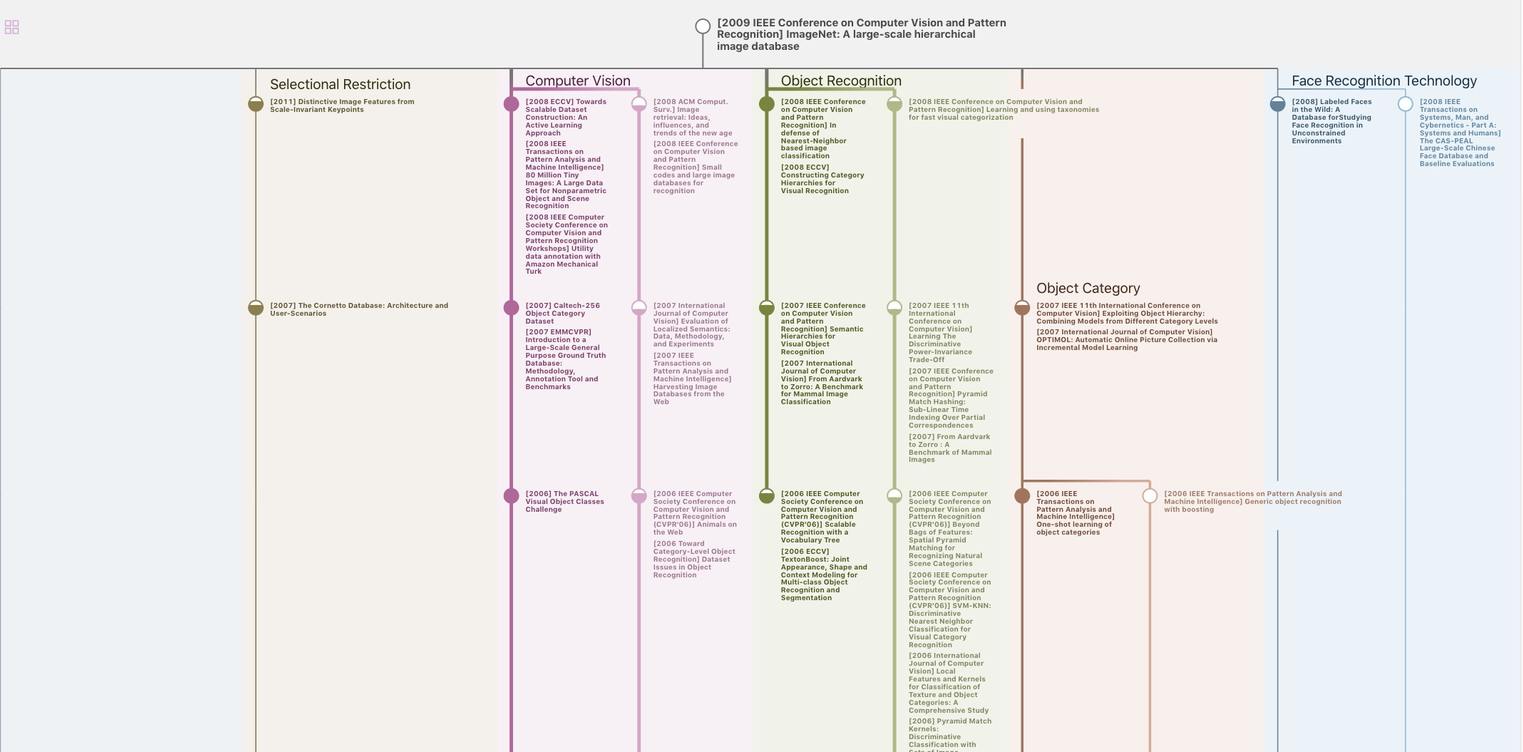
生成溯源树,研究论文发展脉络
Chat Paper
正在生成论文摘要