Learning to rank hotels for search and recommendation from session-based interaction logs and meta data
Proceedings of the Workshop on ACM Recommender Systems Challenge(2019)
摘要
Being able to provide high quality search and recommendation services can be a decisive success factor for online applications, e.g., in today's competitive e-commerce environments. Context-adaptive and personalized item suggestions can help to both improve the user experience and the provider's short-term and long-term revenue. However, automating this form of adaptation can be challenging, when no long-term preference profiles are available. In these situations, the user's preferences and short-term intent must be derived from the last few observed interactions.
In this work, we present a hybrid approach to rank hotels based on the user's most recent interactions and meta data about the available items. The developed recommendation approach can be used both for personalized search and session-based recommendation. Technically, we employed a combination of a gradient-boosted learning-to-rank model, Bayesian Personalized Ranking and an embedding model using Doc2Vec. The approach was successfully evaluated in the context of the ACM RecSys 2019 challenge, where it led our team letoh govatri to the fifth place on the leaderboard, with a ranking accuracy only 0.53% below the winning approach.
更多查看译文
关键词
cold start, hotels, recommendation, search personalization, session-based recommendation, travel and tourism
AI 理解论文
溯源树
样例
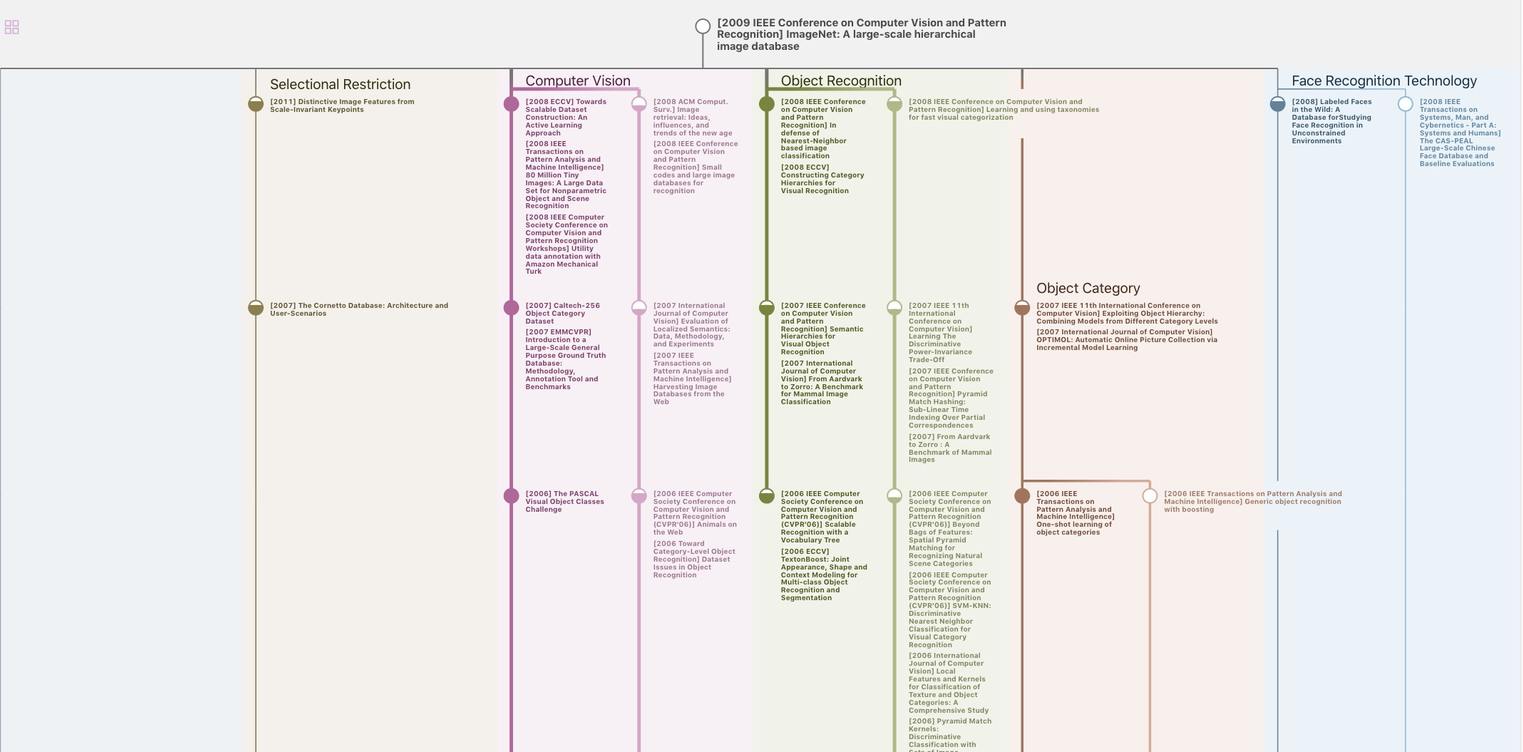
生成溯源树,研究论文发展脉络
Chat Paper
正在生成论文摘要