Molecular Graph Generation with Deep Reinforced Multitask Network and Adversarial Imitation Learning
2019 IEEE International Conference on Bioinformatics and Biomedicine (BIBM)(2019)
摘要
Molecular graph generation aims to design molecules with desired biochemical properties, which is promising in drug discovery. Existing methods typically combine deep reinforcement models with adversarial training. However, the reinforced rewards in molecule generation are delayed and sparse while adversarial training suffers mode collapse issue. Moreover, they optimize multiple properties with a linear combination manner, where the models get distracted by potentially conflicting objectives. To tackle the above challenges, we propose a Deep Reinforced framework with Adversarial Imitation and Multitask learning (DR-AIM). First, the reinforced agent generates discrete molecular graphs via deep Q-learning, where the trajectories of high rewards are cached as experts. Then policies are extracted directly from expert trajectories by adversarial imitation learning, in which the discriminator delivers behavior distribution signals to the agent as dense rewards. Second, we propose to realize multi-goal molecule generation as a multitask learning process, where different property optimizations are treated as different tasks to be trained jointly. Extensive experiments demonstrate the effectiveness of DR-AIM in molecule generation.
更多查看译文
关键词
molecule generation,deep reinforced networks,adversarial imitation learning,multitask optimization
AI 理解论文
溯源树
样例
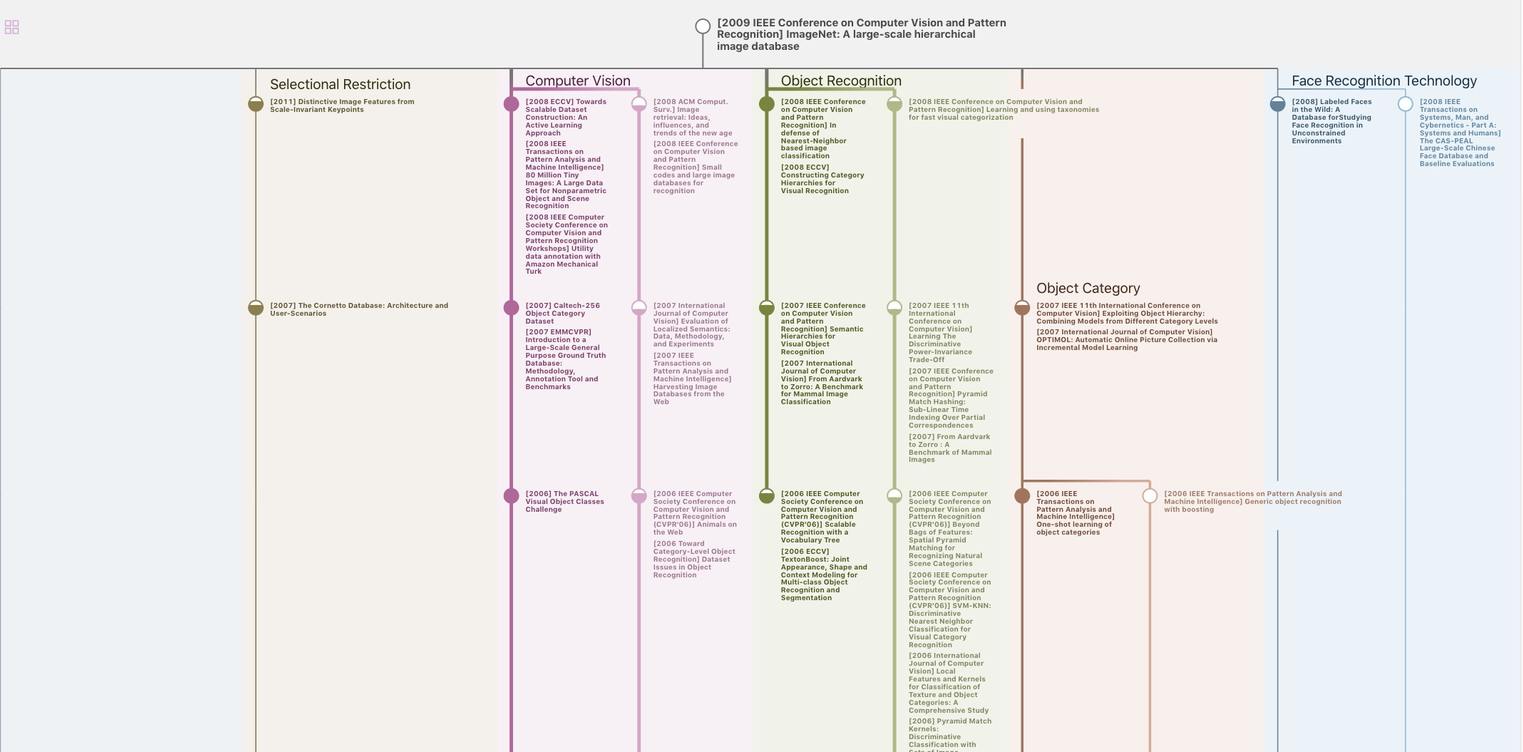
生成溯源树,研究论文发展脉络
Chat Paper
正在生成论文摘要