Improving DWT-RNN Model Via B-spline Wavelet Multiresolution to Forecast a High-Frequency Time Series.
Expert systems with applications(2019)
Abstract
This paper presents a recurrent neural network (RNN) which is improved by using an efficient discrete wavelet transform (DWT) for predicting a high-frequency time series. In the combined DWT-RNN model, first, a multiresolution based on B-spline wavelet of high order d (BSd) is used to decompose the time series into several smooth data sets. Therefore, an approximation data set (with low-frequency) and several detail data sets (with high-frequency), with small wave amplitude, are obtained. Then, all decomposed components are used as RNN inputs. The proposed BSd-RNN model can approximate smooth patterns with satisfactory accuracy, and because of the local properties, BSd is a better choice than other common DWT such as Haar and Daubechies of order n (dbn), for preprocessing the high-frequency time series. According to results of performance metrics for predicting four different stock indices, the BSd-RNN model outperforms other common DWT-RNN model such as Haar-RNN and dbn-RNN. Also, the results show the BSd-RNN model outperforms other common artificial neural network (ANN) model such as multilayer feed-forward neural network (FFNN). Finally, The results show that BS3-RNN predicting model has better predictive ability than other compared models which use other wavelets or other ANNs. (C) 2019 Elsevier Ltd. All rights reserved.
MoreTranslated text
Key words
Discrete wavelet transform,B-spline wavelets multiresolution,Artificial neural networks,Return volatility,Financial time series forecasting
AI Read Science
Must-Reading Tree
Example
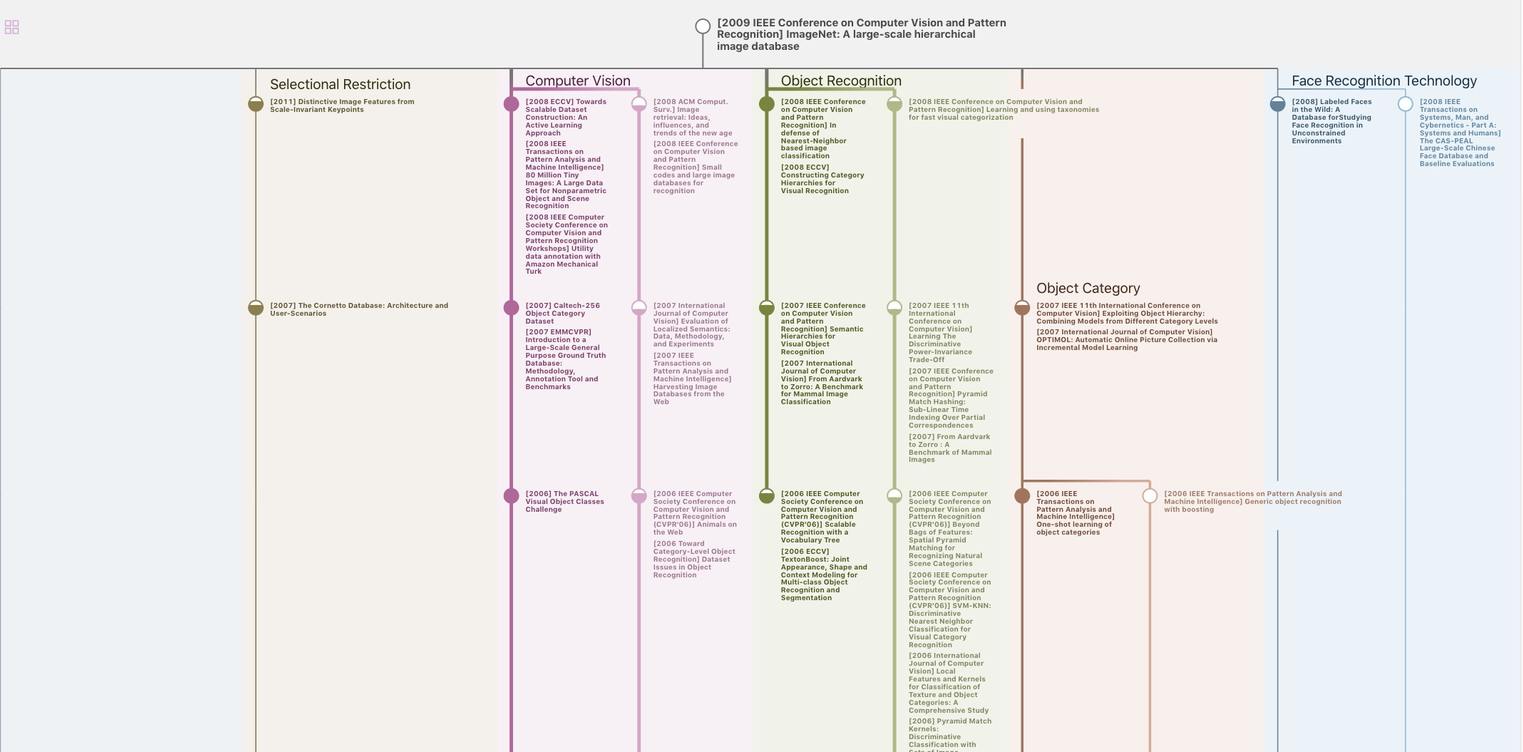
Generate MRT to find the research sequence of this paper
Chat Paper
Summary is being generated by the instructions you defined