DNNRE: A Dynamic Neural Network for Distant Supervised Relation Extraction
arxiv(2019)
摘要
Distant Supervised Relation Extraction (DSRE) is usually formulated as a problem of classifying a bag of sentences that contain two query entities, into the predefined relation classes. Most existing methods consider those relation classes as distinct semantic categories while ignoring their potential connections to each other and query entities. In this paper, we propose to leverage those connections to improve the relation extraction accuracy. Our key ideas are twofold: (1) For sentences belonging to the same relation class, the expression style, i.e. words choice, can vary according to the query entities. To account for this style shift, the model should adjust its parameters in accordance with entity types. (2) Some relation classes are semantically similar, and the mutual relationship of classes can be adopted to enhance the relation predictor. This is especially beneficial for those classes with few samples, i.e., long-tail classes. To unify these two ideas, we developed a novel Dynamic Neural Network for Relation Extraction (DNNRE). The network adopts a novel dynamic parameter generator which dynamically generates the network parameters according to the query entity types, relation classes, and the class similarity matrix. By using this mechanism, the network can simultaneously handle the style shift problem and leverage mutual class relationships to enhance the prediction accuracy for long-tail classes. Through our experimental study, we demonstrate the effectiveness of the proposed method and show that it can achieve superior performance over the state-of-the-art methods.
更多查看译文
AI 理解论文
溯源树
样例
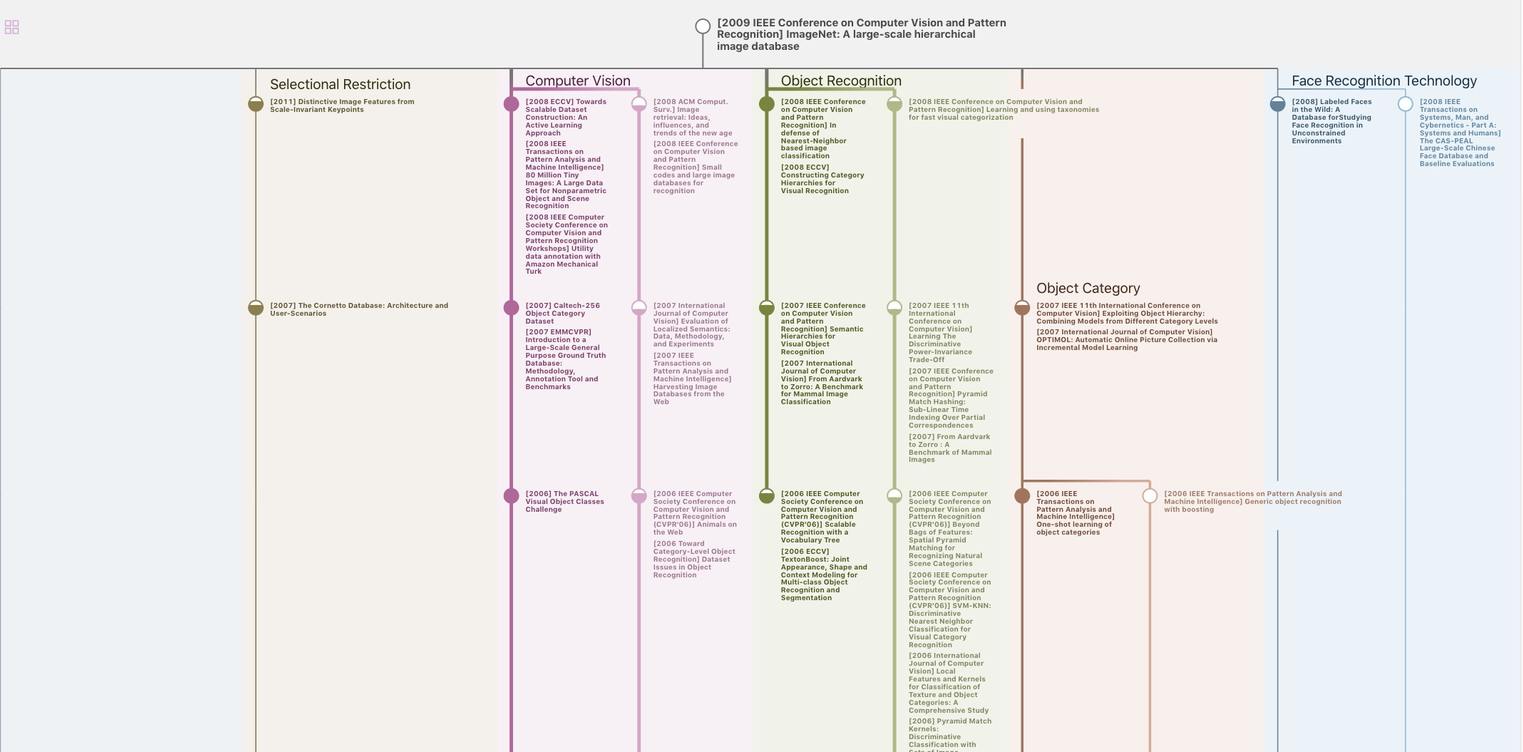
生成溯源树,研究论文发展脉络
Chat Paper
正在生成论文摘要