Clustering of solutions in the symmetric binary perceptron
JOURNAL OF STATISTICAL MECHANICS-THEORY AND EXPERIMENT(2020)
摘要
The geometrical features of the (non-convex) loss landscape of neural network models are crucial in ensuring successful optimization and, most importantly, the capability to generalize well. While minimizers' flatness consistently correlates with good generalization, there has been little rigorous work in exploring the condition of existence of such minimizers, even in toy models. Here we consider a simple neural network model, the symmetric perceptron, with binary weights. Phrasing the learning problem as a constraint satisfaction problem, the analogous of a flat minimizer becomes a large and dense cluster of solutions, while the narrowest minimizers are isolated solutions. We perform the first steps toward the rigorous proof of the existence of a dense cluster in certain regimes of the parameters, by computing the first and second moment upper bounds for the existence of pairs of arbitrarily close solutions. Moreover, we present a non rigorous derivation of the same bounds for sets ofysolutions at fixed pairwise distances.
更多查看译文
关键词
cavity and replica method,energy landscapes,first and second moment method,neural networks
AI 理解论文
溯源树
样例
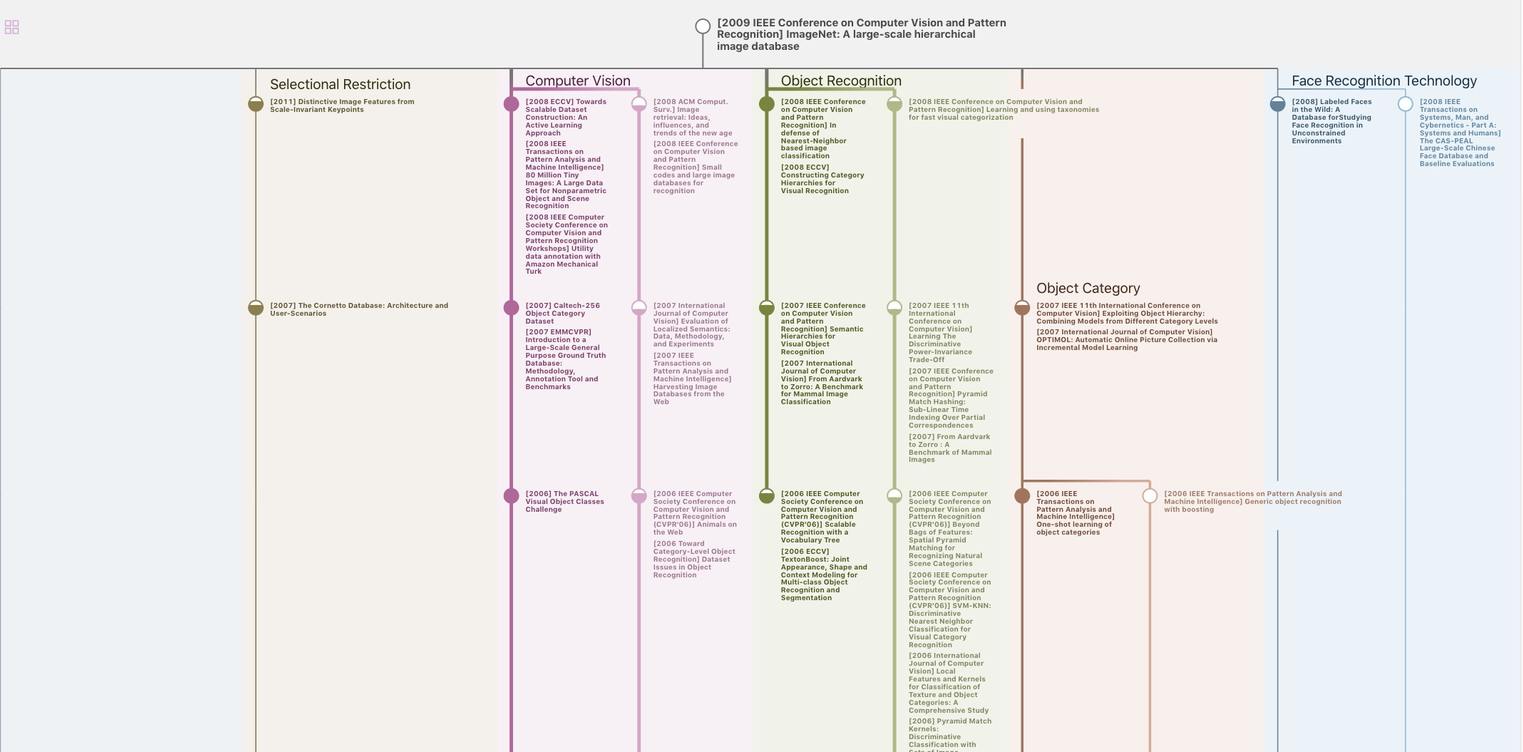
生成溯源树,研究论文发展脉络
Chat Paper
正在生成论文摘要