Search to Distill: Pearls are Everywhere but not the Eyes
CVPR(2020)
摘要
Standard Knowledge Distillation (KD) approaches distill the knowledge of a cumbersome teacher model into the parameters of a student model with a pre-defined architecture. However, the knowledge of a neural network, which is represented by the network's output distribution conditioned on its input, depends not only on its parameters but also on its architecture. Hence, a more generalized approach for KD is to distill the teacher's knowledge into both the parameters and architecture of the student. To achieve this, we present a new Architecture-aware Knowledge Distillation (AKD) approach that finds student models (pearls for the teacher) that are best for distilling the given teacher model. In particular, we leverage Neural Architecture Search (NAS), equipped with our KD-guided reward, to search for the best student architectures for a given teacher. Experimental results show our proposed AKD consistently outperforms the conventional NAS plus KD approach, and achieves state-of-the-art results on the ImageNet classification task under various latency settings. Furthermore, the best AKD student architecture for the ImageNet classification task also transfers well to other tasks such as million level face recognition and ensemble learning.
更多查看译文
关键词
pearls,distill,search
AI 理解论文
溯源树
样例
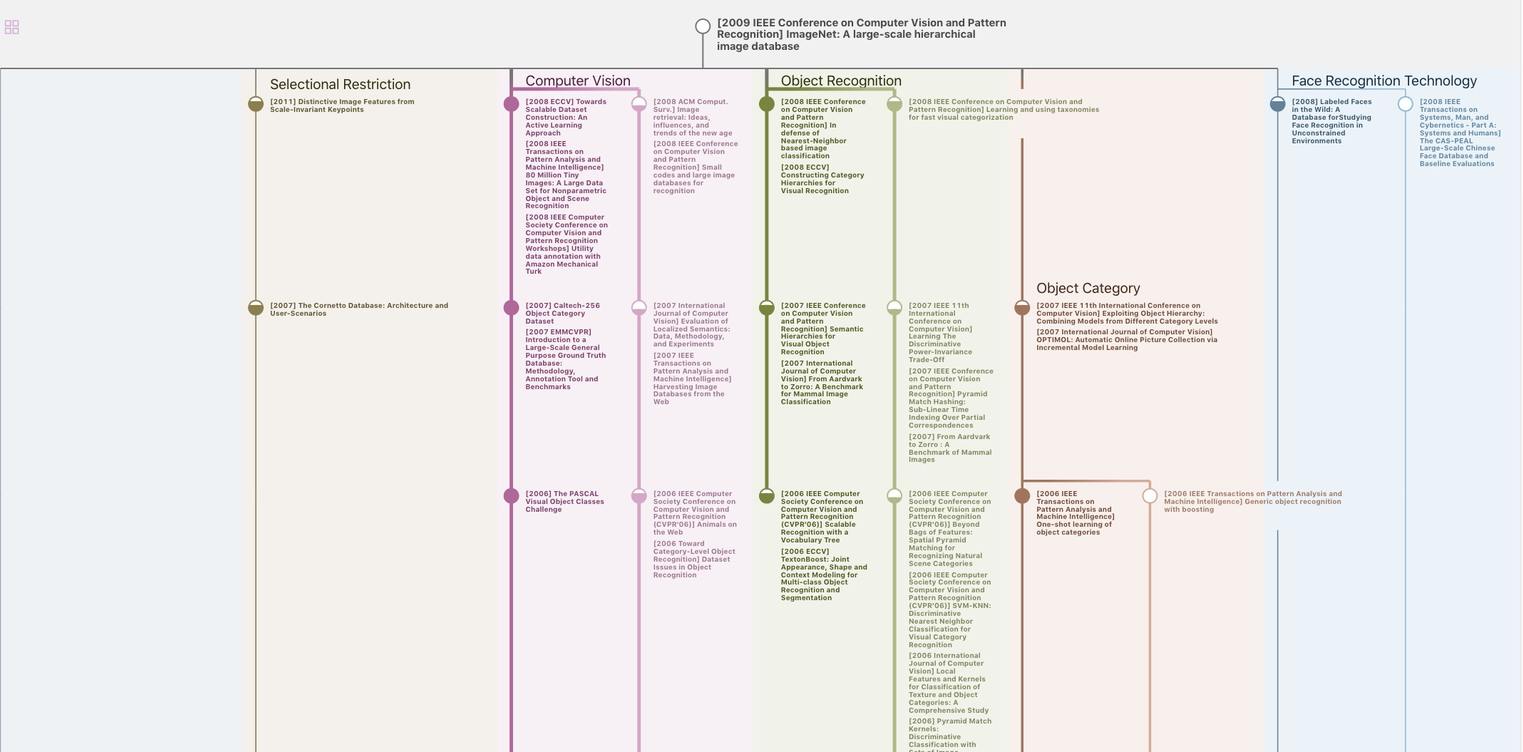
生成溯源树,研究论文发展脉络
Chat Paper
正在生成论文摘要