A Compact Recursive Dense Convolutional Network for image classification.
Neurocomputing(2020)
摘要
In general, the deeper the convolutional network is, the higher the accuracy will be. However, when the depth of the network increases, the number of parameters (weights and bias) will increase quickly, which requires a lot of storage space when it is applied in real life. Considering the parameters’ efficiency for the deep network, we propose an improved deep convolutional network, named Recursive Dense Convolutional Network (RDenseNet), inspired by the recursive idea. The proposed RDenseNet still utilizes the dense block in Dense Convolutional Network (DenseNet) as a basic structure, but different from the cascade structure of DenseNet, our proposed RDenseNet uses the output of the dense block as input for the current block again. That is, our proposed RDenseNet turns the cascade structure in DenseNet into a recursive structure, which can reduce the number of parameters that actually need to be stored. Experiments illustrate that our proposed RDenseNet can still keep or exceed the accuracy of some state-of-the-art deep networks with much less parameters.
更多查看译文
关键词
Classification,Convolutional network,Recursion,Dense block
AI 理解论文
溯源树
样例
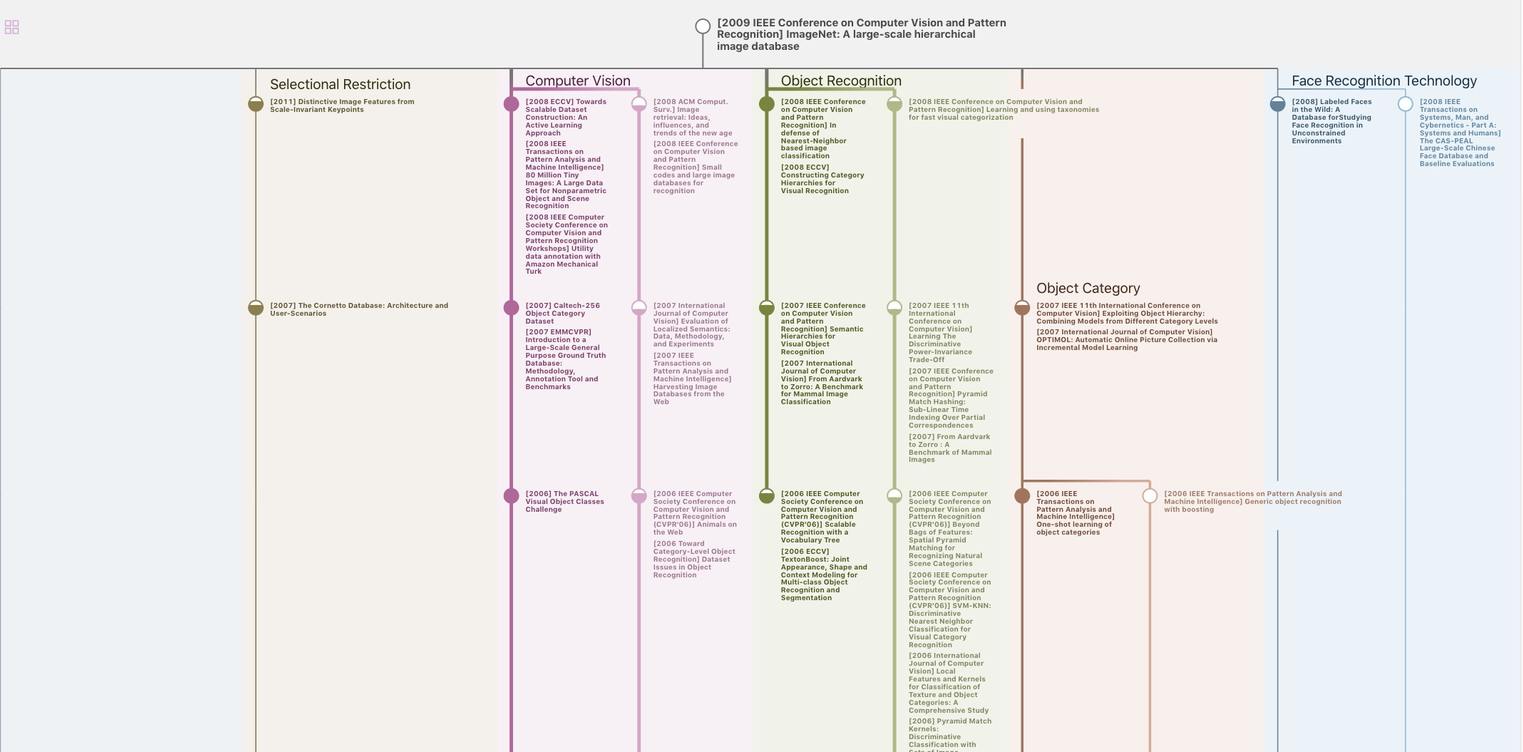
生成溯源树,研究论文发展脉络
Chat Paper
正在生成论文摘要