Improving Hyperspectral Image Classification By Combining Spectral And Multiband Compact Texture Features
2019 IEEE INTERNATIONAL GEOSCIENCE AND REMOTE SENSING SYMPOSIUM (IGARSS 2019)(2019)
摘要
Several studies have demonstrated the efficiency of using spatial information in representation of hyperspectral (HS) images. Texture features are known as one of the most important categories of spatial information in various applications of image processing. This study evaluates the capability of recently proposed descriptors named multiband compact texture unit. This method extracts texture by characterizing simultaneously spatial relationship in the same band and across the different bands. The proposed evaluation is performed in the context of patch-based classification paradigm using two HS datasets. For that, objects were generated through superpixel segmentation. The classification in the object-feature space is performed using a random forest algorithm. The proposed approach is compared to various other color-texture analysis methods, including: Integrative gray-level co-occurrence matrix, Opponent Gabor features and Opponent local binary patterns. Experimental results show that multiband compact texture unit method produces the best results.
更多查看译文
关键词
Hyperspectral imagery, multiband compact texture analysis, superpixel segmentation, random forest classification
AI 理解论文
溯源树
样例
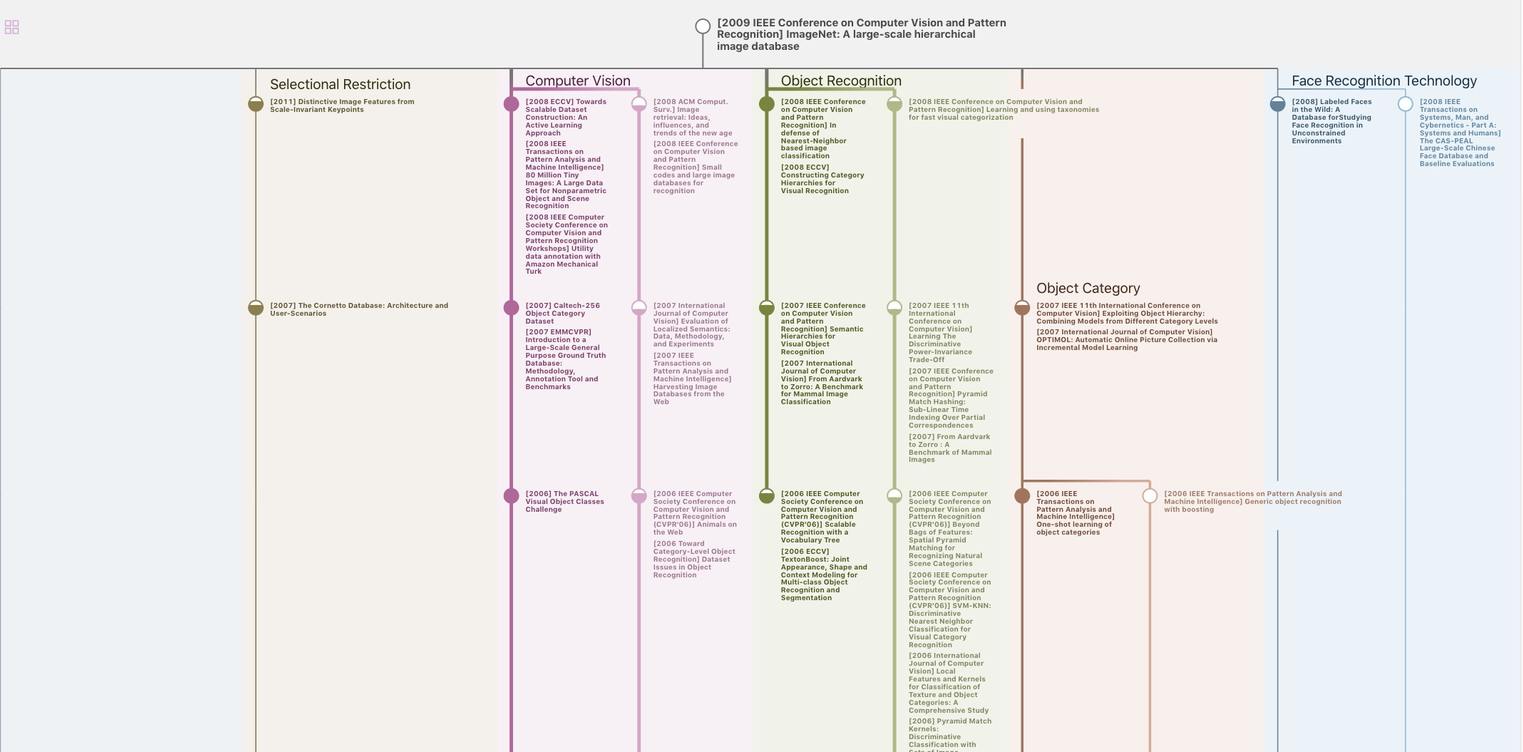
生成溯源树,研究论文发展脉络
Chat Paper
正在生成论文摘要