Comparison Of Deep Learning Model Performance Between Meta-Dataset Training Versus Deep Neural Ensembles
2019 IEEE INTERNATIONAL GEOSCIENCE AND REMOTE SENSING SYMPOSIUM (IGARSS 2019)(2019)
摘要
Recently, many high-resolution remote sensing imagery (HR-RSI) datasets have been released that have diverse characteristics, such as high inter-class and low intra-class variation. Additionally, a benchmark meta-dataset (MDS) was created by agglomerating object classes from multiple HR-RSI datasets. Previous work has shown that deep convolutional neural networks (DCNN) trained on the MDS perform on par with DCNN trained on constituent benchmark datasets in cross-validation experiments. Here we train a model ensemble on four datasets and compare it with a single robust DCNN trained on the MDS. The goal is to better understand under what conditions an ensemble of models, each trained with distinct datasets, is advantaged or disadvantaged compared to a single model trained with the agglomerated MDS for classification performance.
更多查看译文
关键词
Deep Learning, Fusion, Ensemble
AI 理解论文
溯源树
样例
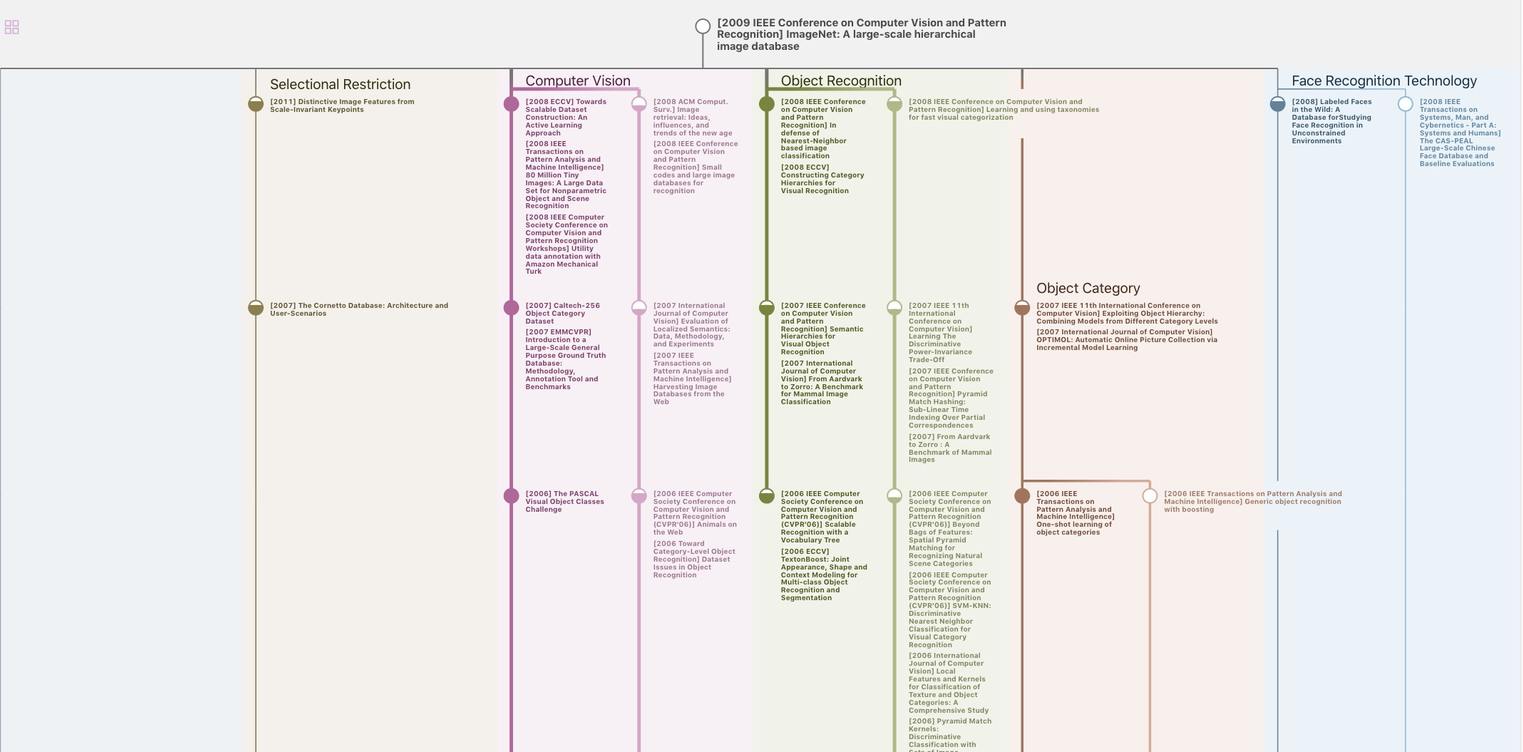
生成溯源树,研究论文发展脉络
Chat Paper
正在生成论文摘要