Simultaneous Segmentation And Edge Detection For Hyperspectral Image Via A Deep Supervised And Boundary-Constrained Network
2019 IEEE INTERNATIONAL GEOSCIENCE AND REMOTE SENSING SYMPOSIUM (IGARSS 2019)(2019)
摘要
Recent research has shown the great potential of convolutional neural networks (CNNs) in hyperspectral image (HSI) classification. Nevertheless, CNN based approaches may lead to over-smoothing effect due to the spatial information loss during the convolution and pooling operations. To address this problem, in this paper, we propose a deep supervised and boundary-constrained network (DSBC-Net) which takes the boundary information into consideration. With the well-designed architecture, DSBC-Net can achieve segmentation and edge detection for HSI simultaneously. Besides, a novel deep supervision strategy is proposed to improve the training of the deep neural network. Experimental results on two benchmark HSI datasets demonstrate that the DSBC-Net can better maintain the boundaries of different objects with higher classification accuracy compared with previous methods.
更多查看译文
关键词
Hyperspectral image classification, edge detection, convolutional neural network (CNN), deep learning
AI 理解论文
溯源树
样例
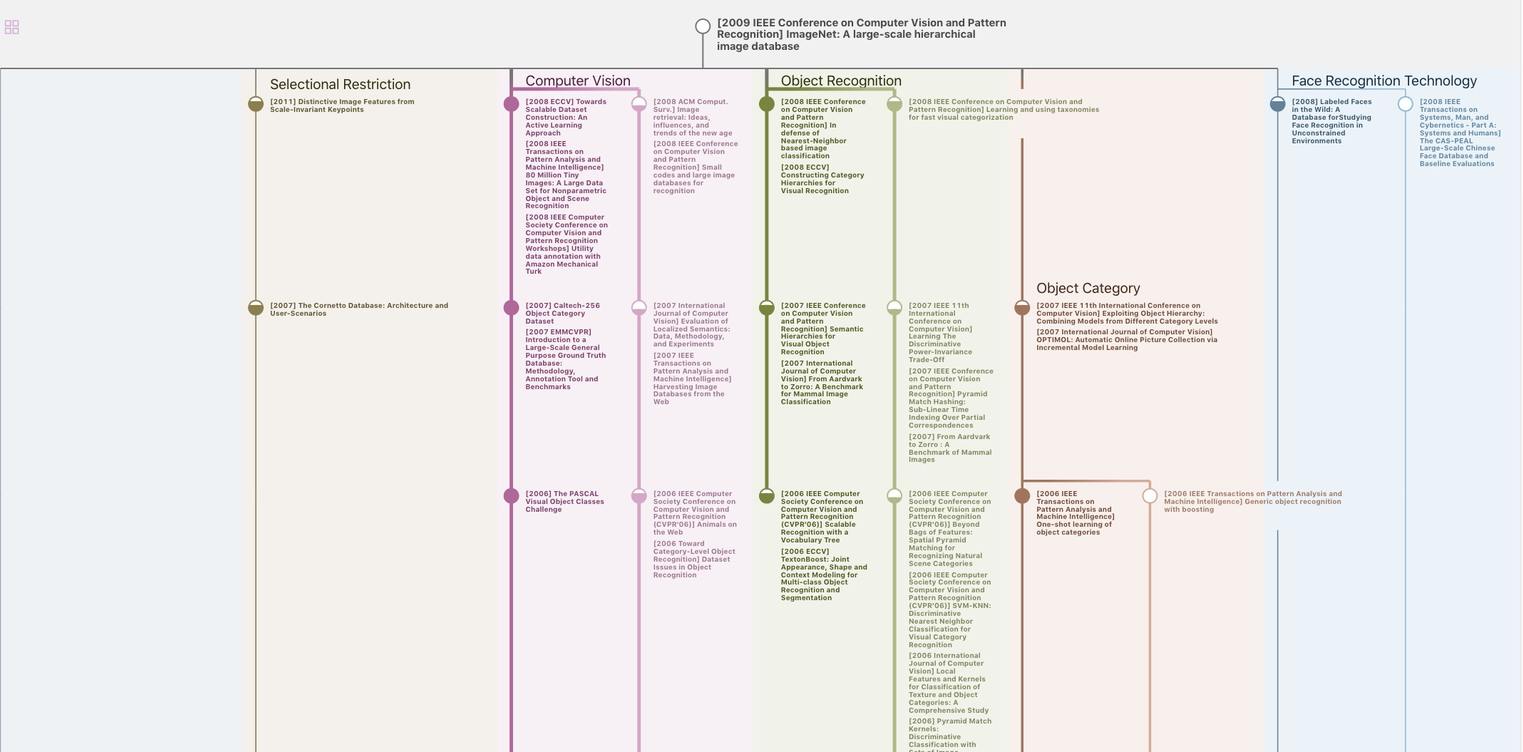
生成溯源树,研究论文发展脉络
Chat Paper
正在生成论文摘要