Hyperspectral Image Classification Based on Generative Adversarial Networks with Feature Fusing and Dynamic Neighborhood Voting Mechanism
IGARSS(2019)
摘要
Classifying Hyperspectral images with few training samples is a challenging problem. The generative adversarial networks (GAN) are promising techniques to address the problems. GAN constructs an adversarial game between a discriminator and a generator. The generator generates samples that are not distinguishable by the discriminator, and the discriminator determines whether or not a sample is composed of real data. In this paper, by introducing multilayer features fusion in GAN and a dynamic neighborhood voting mechanism, a novel algorithm for HSIs classification based on 1-D GAN was proposed. Extracting and fusing multiple layers features in discriminator, and using a little labeled samples, we fine-tuned a new sample 1-D CNN spectral classifier for HSIs. In order to improve the accuracy of the classification, we proposed a dynamic neighborhood voting mechanism to classify the HSIs with spatial features. The obtained results show that the proposed models provide competitive results compared to the state-of-the-art methods.
更多查看译文
关键词
Hyperspectral images classification,semi-supervised learning (SSL),generative adversarial networks (GAN),deep learning,spectral-spatial classification
AI 理解论文
溯源树
样例
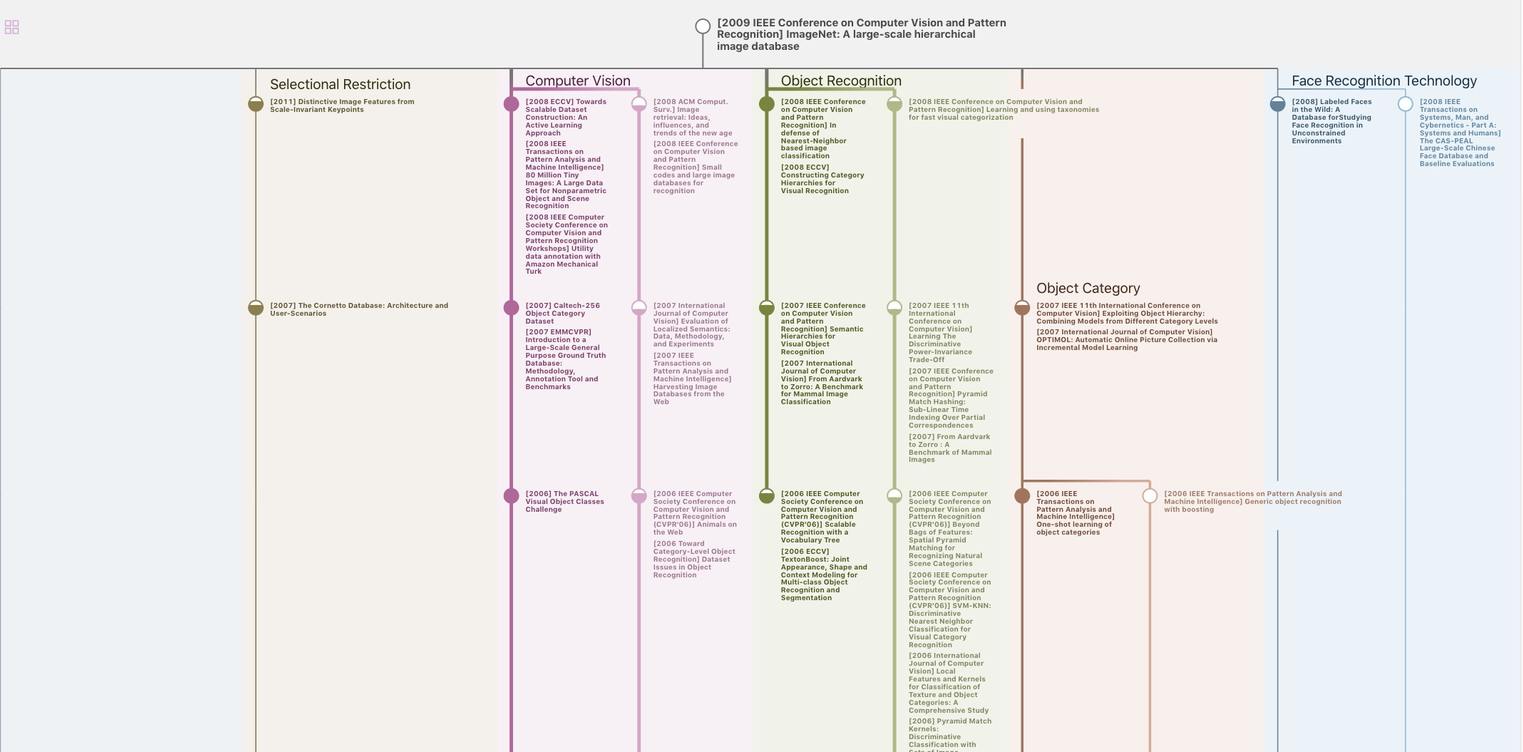
生成溯源树,研究论文发展脉络
Chat Paper
正在生成论文摘要