A Deep Learning Approach Using DeepGBM for Credit Assessment
Proceedings of the 2019 International Conference on Robotics, Intelligent Control and Artificial Intelligence(2019)
摘要
In the loan business, the bank needs to conduct credit assessment on customers to reduce the loan risk. How to assess personal credit has become a problem which is worth studying. In the traditional credit assessment methods, logistic regression, decision tree, random forest, and other methods were often used to conduct credit assessment for individuals. In recent years, a new machine learning method, LightGBM [1] has also been used in credit assessment and achieved good results. In the models mentioned above, the problem of sparse categorical features and dense numerical features of the credit assessment data set is not solved yet. DeepGBM[2] proposed by Guolin Ke, Zhenhui Xu* and Jia Zhang can solve the problem of credit assessment data set very well. Therefore, our research adopted the latest deep learning framework DeepGBM. The deep learning framework of DeepGBM consists of two parts, CatNN, and GBDT2NN, which are used to deal with sparse categorical features and dense numerical features, respectively. This paper used a data set from Kaggle: Home Credit Default Risk. We had conducted several different experimental methods on this data set. The final results of these experiments demonstrate that the performance of DeepGBM is better than other models.
更多查看译文
关键词
Credit assessment, DeepGBM, GBDT, Machine learning
AI 理解论文
溯源树
样例
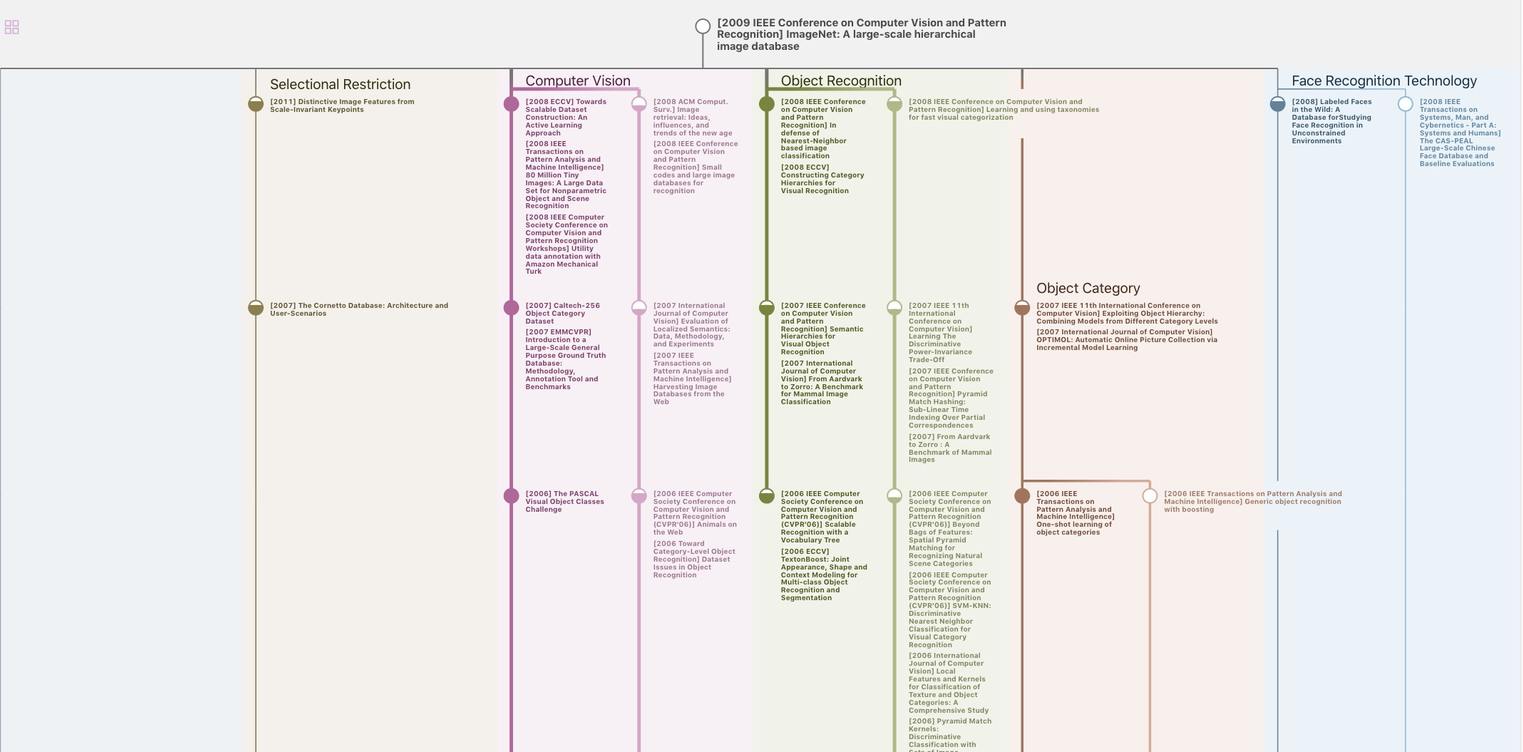
生成溯源树,研究论文发展脉络
Chat Paper
正在生成论文摘要