Visual Question Answering with Dynamic Parameter Prediction using Functional Hashing
Proceedings of the 2019 International Conference on Robotics, Intelligent Control and Artificial Intelligence(2019)
摘要
The dynamic parameter prediction model (DPPnet) established a mapping between the original space and the compression space by HashedNet for Visual Question Answering (VQA). HashedNet is a single-seeded random hash function, so the collision rate is linear to the compression ratio. In order to relieve the interference of the conflict rate to VQA and improve the stability and accuracy of the model, we introduce a multi-seed random hashing compression named functional hashing to be used as the fusion mechanism in this paper. Multiple pairs of independent random hash function are active and the variable parameters among them are trained by a lightweight reconfigurable neural network, making the compression mechanism more flexible. In addition, we use Bi-GRU to extract the historical and future context information from question sentences. At the same time, we propose gated convolution to selectively learn the feature from images questioned dynamically. Experimental results show that our proposed method achieves higher accuracy compared with existing models.
更多查看译文
关键词
Bi-GRU, Fusion mechanism, Gated convolution, Visual Question Answering (VQA)
AI 理解论文
溯源树
样例
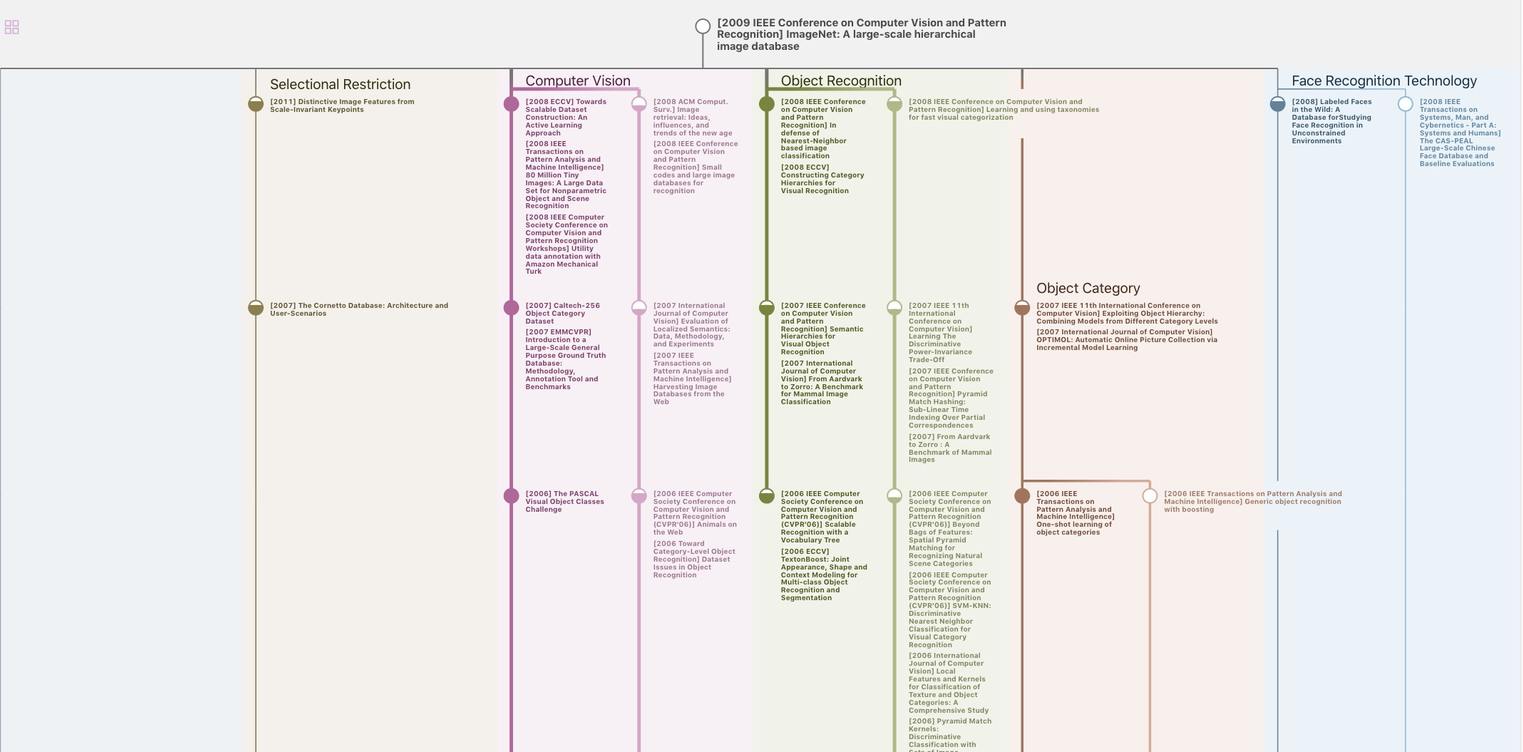
生成溯源树,研究论文发展脉络
Chat Paper
正在生成论文摘要