Hyperspectral Image Classification Based on Two-Phase Relation Learning Network
IEEE Transactions on Geoscience and Remote Sensing(2019)
摘要
Deep learning-based classification methods are competent to achieve an excellent performance under one necessary condition, i.e., there are sufficient labeled samples in each class, which is extremely impractical in most of the remote sensing tasks. To improve the performance with small training sets, we resort to other hyperspectral images and design a two-phase relation learning network that can be transferred between different images for general information sharing and fine-trained on a specific hyperspectral image for individual information learning. Specifically, we use a relation learning method to compare samples and deal with the task inconsistency between different data sets, and we adopt an episode-based training strategy to mimic the testing setup and learn the transferable comparison ability. Benefited from these two strategies, the proposed network takes the advantage of extra knowledge for information supplement and learns to compare rather than to classify for information exploration, which guarantees a reasonable performance even with small training sets. Extensive experiments and analysis on three benchmarks demonstrate that the proposed method can provide an effective solution for hyperspectral image classification with small training sets, which makes it possible to work on large-scale applications of earth observation with less effort on field investigation.
更多查看译文
关键词
Hyperspectral imaging,Training,Measurement,Deep learning,Task analysis
AI 理解论文
溯源树
样例
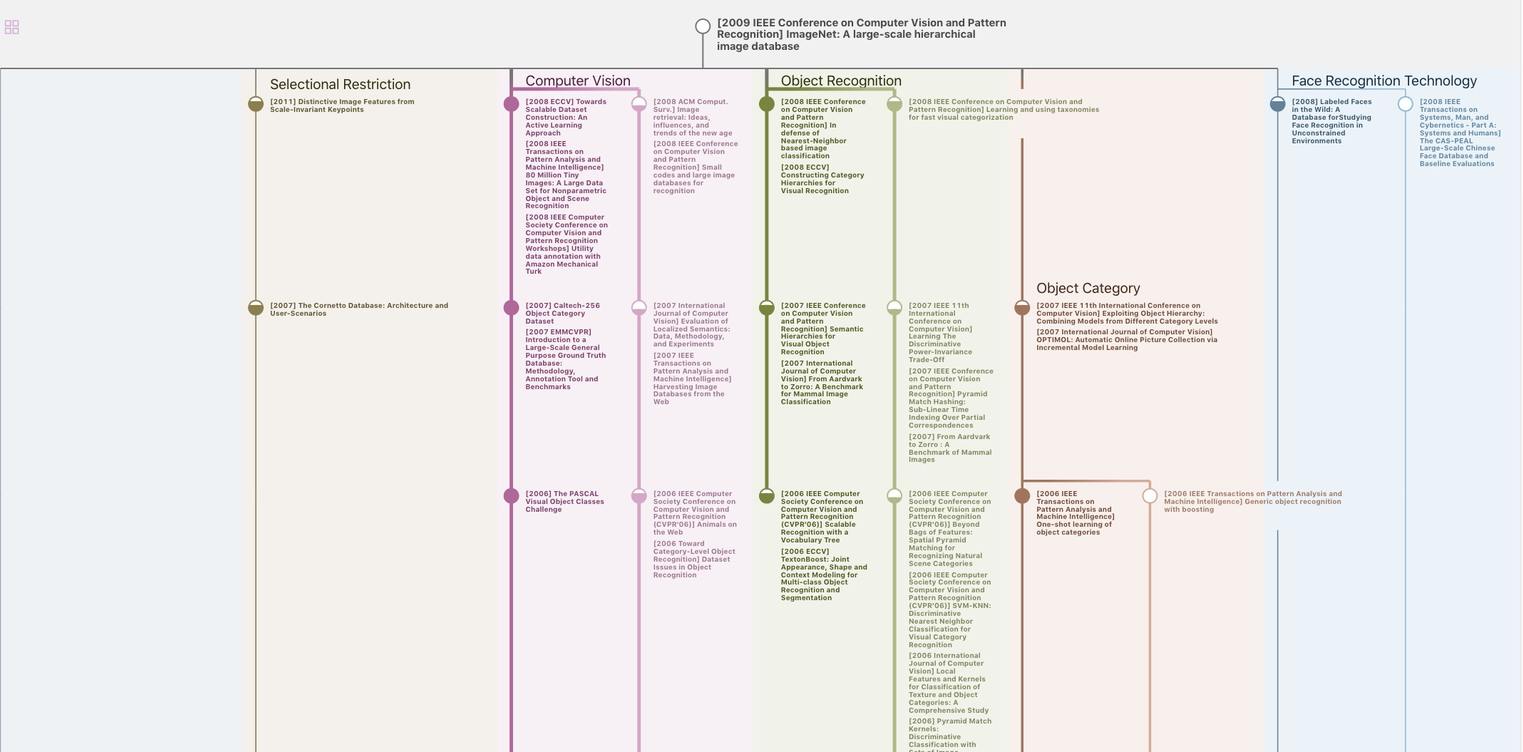
生成溯源树,研究论文发展脉络
Chat Paper
正在生成论文摘要