High Spatio-Temporal Resolution CYGNSS Soil Moisture Estimates Using Artificial Neural Networks.
REMOTE SENSING(2019)
摘要
This paper presents a learning-based, physics-aware soil moisture (SM) retrieval algorithm for NASA's Cyclone Global Navigation Satellite System (CYGNSS) mission. The goal of the proposed novel method is to advance CYGNSS-based SM estimations, exploiting the spatio-temporal resolution of the GNSS reflectometry (GNSS-R) signals to its highest potential within a machine learning framework. The methodology employs a fully connected Artificial Neural Network (ANN) regression model to perform SM predictions through learning the nonlinear relations of SM and other land geophysical parameters to the CYGNSS observables. In situ SM measurements from several International SM Network (ISMN) sites are used as reference labels; CYGNSS incidence angles, derived reflectivity and trailing edge slope (TES) values, as well as ancillary data, are exploited as input features for training and validation of the ANN model. In particular, the utilized ancillary data consist of normalized difference vegetation index (NDVI), vegetation water content (VWC), terrain elevation, terrain slope, and h-parameter (surface roughness). Land cover classification and inland water body masks are also used for the intermediate derivations and quality control purposes. The proposed algorithm assumes uniform SM over a 0.0833ffi similar to 0.0833ffi (approximately 9 km similar to 9 km around the equator) lat/lon grid for any CYGNSS observation that falls within this window. The proposed technique is capable of generating sub-daily and high-resolution SM predictions as it does not rely on time-series or spatial averaging of the CYGNSS observations. Once trained on the data from ISMN sites, the model is independent from other SM sources for retrieval. The estimation results obtained over unseen test data are promising: SM predictions with an unbiased root mean squared error of 0.0544 cm3/cm3 and Pearson correlation coefficient of 0.9009 are reported for 2017 and 2018.
更多查看译文
关键词
artificial neural networks,CYGNSS,soil moisture retrieval
AI 理解论文
溯源树
样例
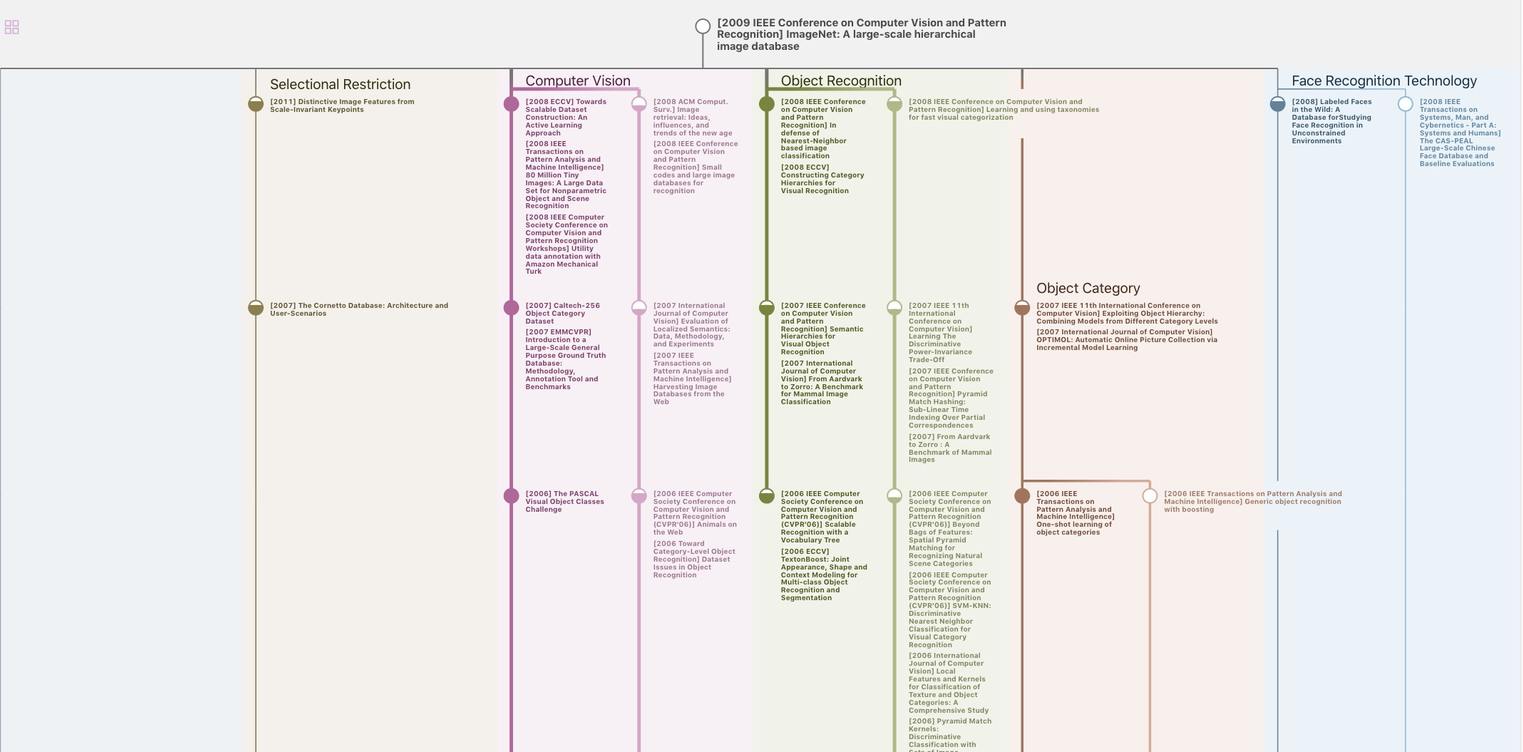
生成溯源树,研究论文发展脉络
Chat Paper
正在生成论文摘要