CoralSeg: Learning coral segmentation from sparse annotations.
JOURNAL OF FIELD ROBOTICS(2019)
摘要
Robotic advances and developments in sensors and acquisition systems facilitate the collection of survey data in remote and challenging scenarios. Semantic segmentation, which attempts to provide per-pixel semantic labels, is an essential task when processing such data. Recent advances in deep learning approaches have boosted this task's performance. Unfortunately, these methods need large amounts of labeled data, which is usually a challenge in many domains. In many environmental monitoring instances, such as the coral reef example studied here, data labeling demands expert knowledge and is costly. Therefore, many data sets often present scarce and sparse image annotations or remain untouched in image libraries. This study proposes and validates an effective approach for learning semantic segmentation models from sparsely labeled data. Based on augmenting sparse annotations with the proposed adaptive superpixel segmentation propagation, we obtain similar results as if training with dense annotations, significantly reducing the labeling effort. We perform an in-depth analysis of our labeling augmentation method as well as of different neural network architectures and loss functions for semantic segmentation. We demonstrate the effectiveness of our approach on publicly available data sets of different real domains, with the emphasis on underwater scenarios-specifically, coral reef semantic segmentation. We release new labeled data as well as an encoder trained on half a million coral reef images, which is shown to facilitate the generalization to new coral scenarios.
更多查看译文
关键词
coral reefs,learning,machine learning,perception,underwater robotics
AI 理解论文
溯源树
样例
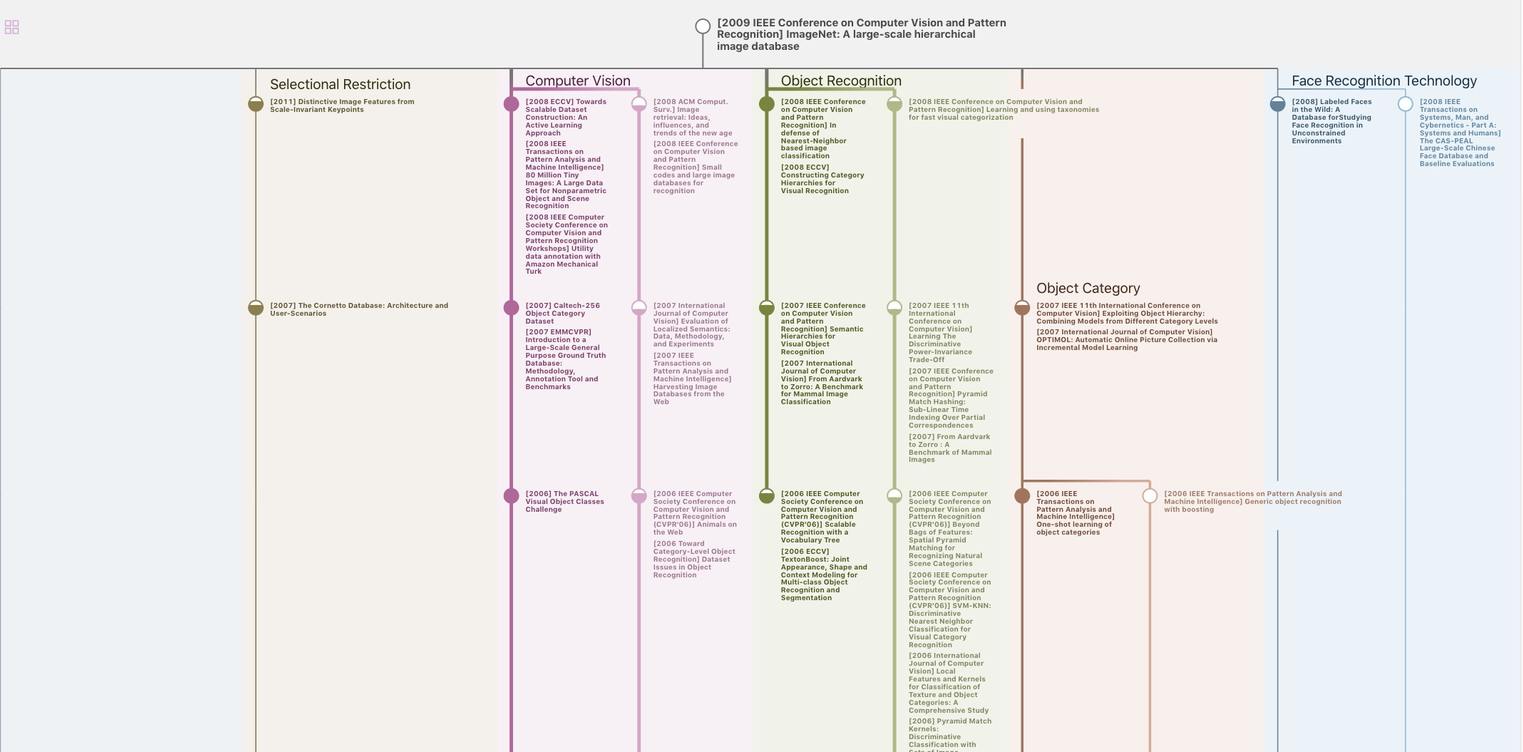
生成溯源树,研究论文发展脉络
Chat Paper
正在生成论文摘要