Very High Resolution Image Semantic Segmentation With Contextualized Convolutional Neural Network Coupled With Higher Order Conditional Random Fields
2019 IEEE 15TH INTERNATIONAL CONFERENCE ON CONTROL AND AUTOMATION (ICCA)(2019)
摘要
Feature representation and context incorporation play a key role in image semantic segmentation. We propose a framework for very high resolution image semantic segmentation which is able to combine learned internal representation and context in a unified manner. The proposed method is based on contextualized convolutional neural networks and high order conditional random field to learn feature representation and encode context feature respectively. We first devise an architecture to obtain learned feature representation which helps to keep semantic consistency within classes by encoding semantic edge context into convolutional neural networks. Then, the learned features and superpixel based higher order potential are integrated into a conditional random field to infer semantic segmentation. Both pixel-level and region-level potential are considered in conditional random field to address high variation within classes. Experimental results on public available semantic segmentation contest dataset demonstrated the effectiveness of the proposed method.
更多查看译文
关键词
higher order conditional random fields,context incorporation,high resolution image semantic segmentation,learned internal representation,contextualized convolutional neural network,high order conditional random field,encode context feature,learned feature representation,semantic consistency,semantic edge context,convolutional neural networks,superpixel,higher order potential,public available semantic segmentation contest dataset
AI 理解论文
溯源树
样例
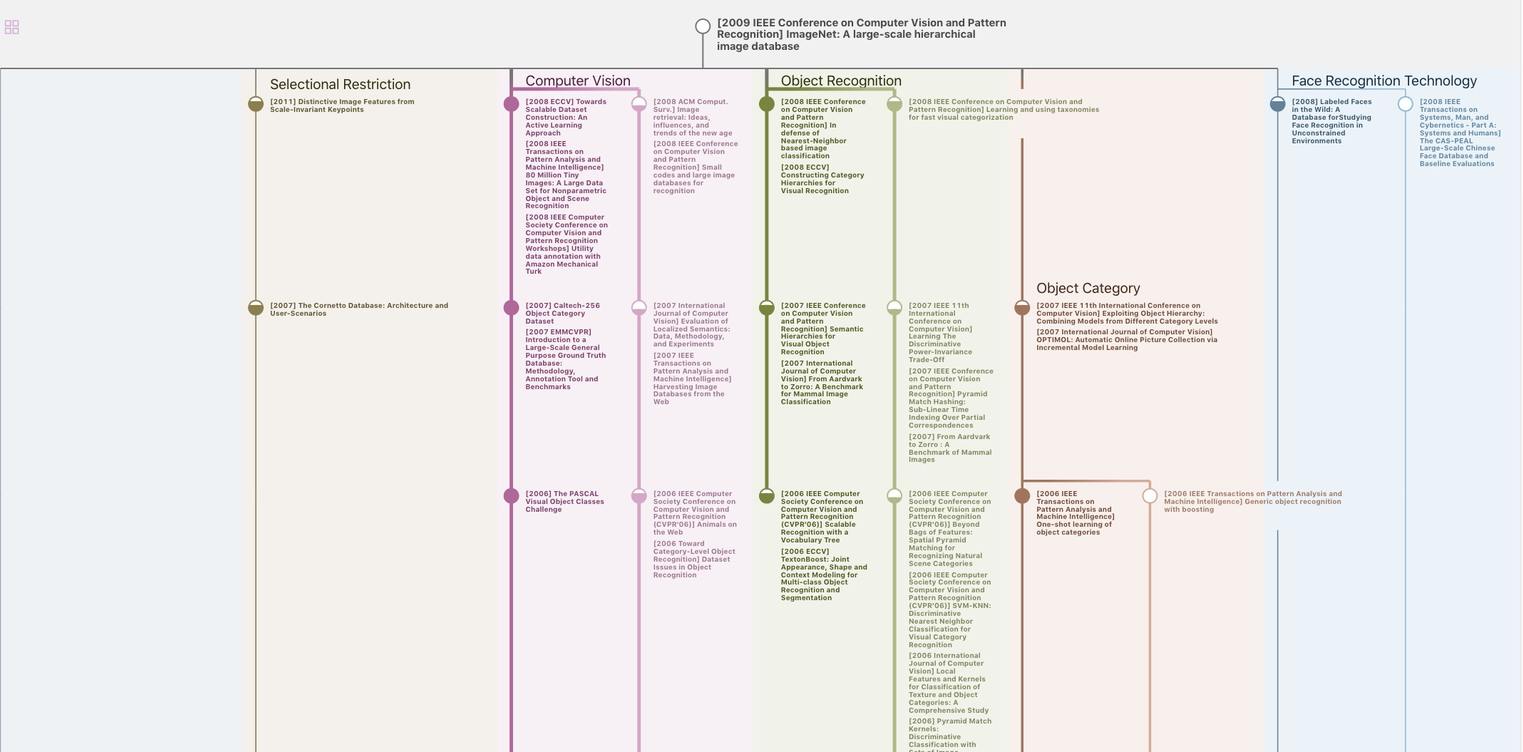
生成溯源树,研究论文发展脉络
Chat Paper
正在生成论文摘要