Speech Sentiment Analysis via Pre-trained Features from End-to-end ASR Models
2020 IEEE INTERNATIONAL CONFERENCE ON ACOUSTICS, SPEECH, AND SIGNAL PROCESSING(2019)
摘要
In this paper, we propose to use pre-trained features from end-to-end ASR models to solve the speech sentiment analysis problem as a down-stream task. We show that end-to-end ASR features, which integrate both acoustic and text information from speech, achieve promising results. We use RNN with self-attention as the sentiment classifier, which also provides an easy visualization through attention weights to help interpret model predictions. We use well benchmarked IEMOCAP dataset and a new large-scale sentiment analysis dataset SWBD-senti for evaluation. Our approach improves the-state-of-the-art accuracy on IEMOCAP from 66.6% to 71.7%, and achieves an accuracy of 70.10% on SWBD-senti with more than 49,500 utterances.
更多查看译文
关键词
Speech sentiment analysis, ASR pretraining, End-to-end ASR model
AI 理解论文
溯源树
样例
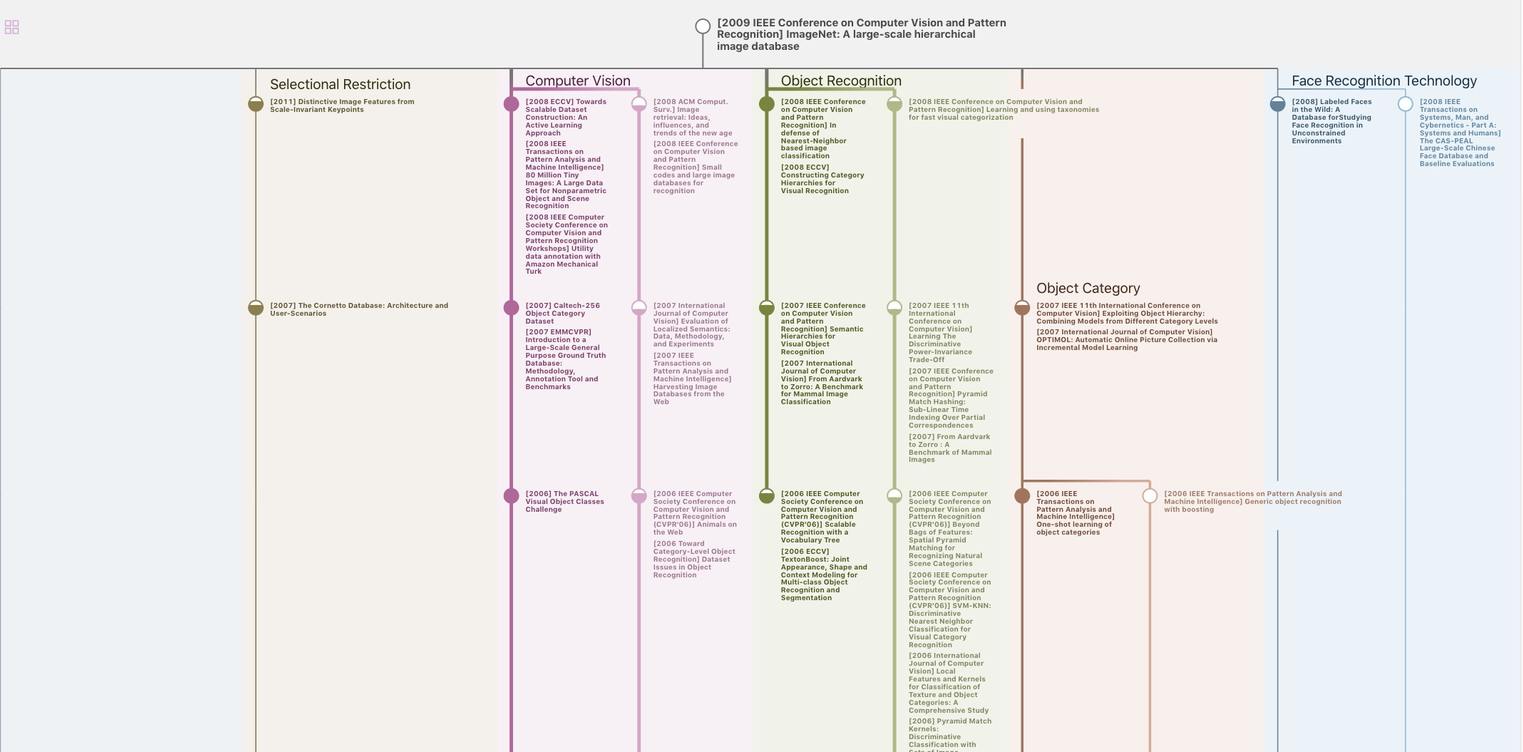
生成溯源树,研究论文发展脉络
Chat Paper
正在生成论文摘要