Fast Polynomial Kernel Classification for Massive Data
CoRR(2022)
摘要
In the era of big data, it is desired to develop efficient machine learning algorithms to tackle massive data challenges such as storage bottleneck, algorithmic scalability, and interpretability. In this paper, we develop a novel efficient classification algorithm, called fast polynomial kernel classification (FPC), to conquer the scalability and storage challenges. Our main tools are a suitable selected feature mapping based on polynomial kernels and an alternating direction method of multipliers (ADMM) algorithm for a related non-smooth convex optimization problem. Fast learning rates as well as feasibility verifications including the efficiency of an ADMM solver with convergence guarantees and the selection of center points are established to justify theoretical behaviors of FPC. Our theoretical assertions are verified by a series of simulations and real data applications. Numerical results demonstrate that FPC significantly reduces the computational burden and storage memory of existing learning schemes such as support vector machines, Nystr\"{o}m and random feature methods, without sacrificing their generalization abilities much.
更多查看译文
AI 理解论文
溯源树
样例
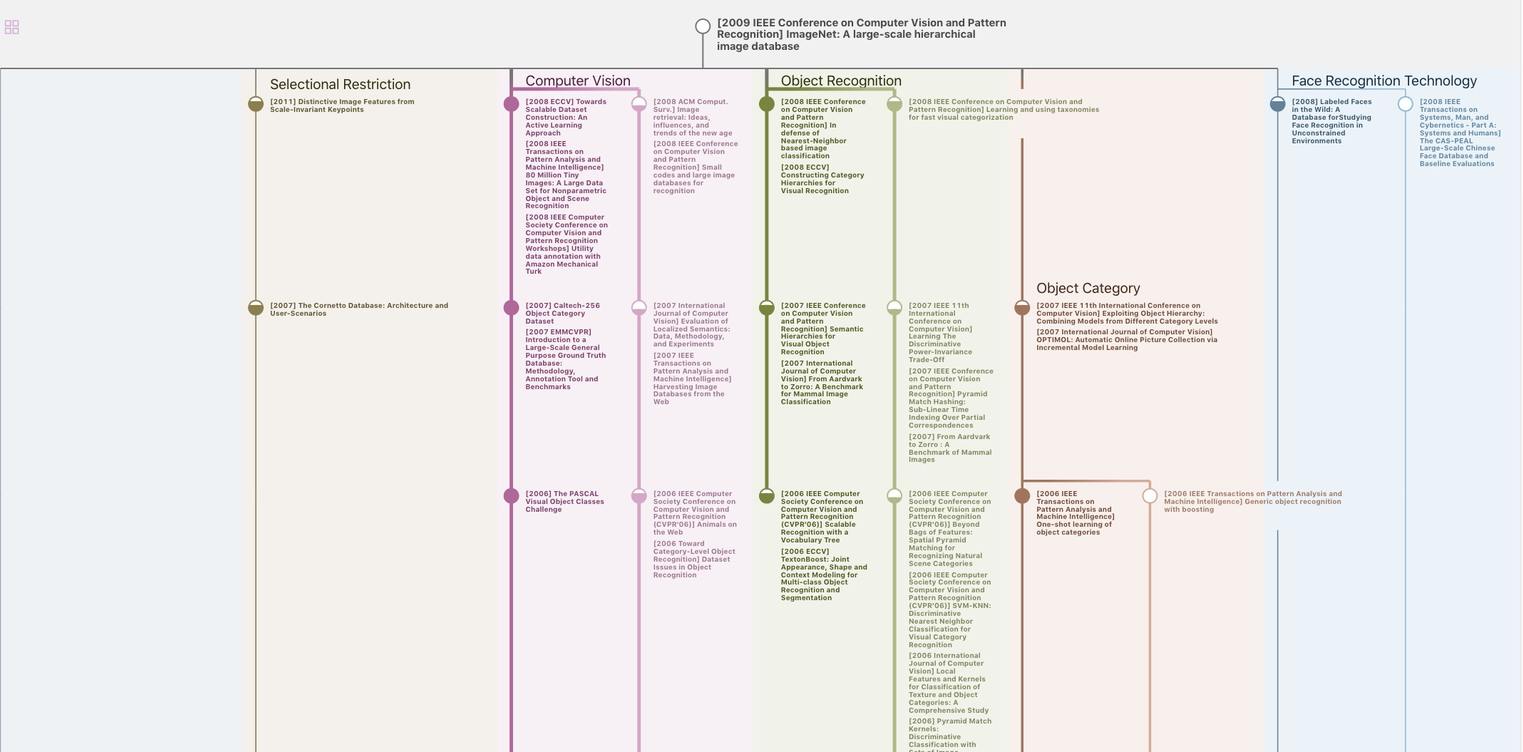
生成溯源树,研究论文发展脉络
Chat Paper
正在生成论文摘要