Trajectory Growth Lower Bounds for Random Sparse Deep ReLU Networks
arXiv (Cornell University)(2019)
摘要
This paper considers the growth in the length of one-dimensional trajectories as they are passed through random deep ReLU networks. We generalise existing results, providing an alternative, simpler method for lower bounding expected trajectory growth through random networks, for a more general class of weights distributions, including sparsely connected networks. We illustrate this approach by deriving bounds for sparse-Gaussian, sparse-uniform, and sparse-discrete-valued random nets. We prove that trajectory growth can remain exponential in such networks, with the sparsity parameter appearing in the base of the exponent.
更多查看译文
关键词
deep learning,random curves,random sparse matrices,expected arc length,expressivity
AI 理解论文
溯源树
样例
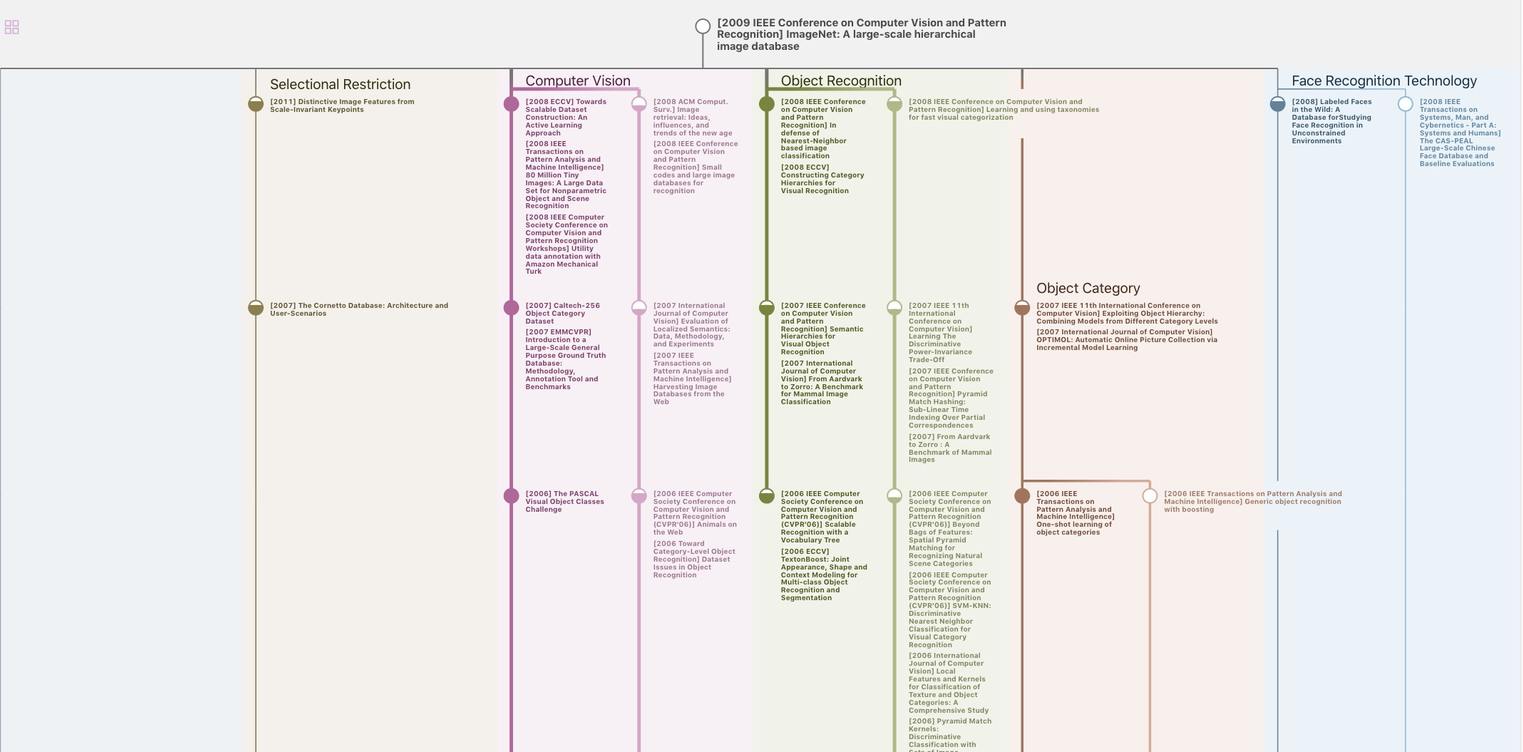
生成溯源树,研究论文发展脉络
Chat Paper
正在生成论文摘要